Part-dependent Label Noise: Towards Instance-dependent Label Noise
NIPS 2020(2020)
摘要
Learning with the \textit{instance-dependent} label noise is challenging, because it is hard to model such real-world noise. Note that there are psychological and physiological evidences showing that we humans perceive instances by decomposing them into parts. Annotators are therefore more likely to annotate instances based on the parts rather than the whole instances. Motivated by this human cognition, in this paper, we approximate the instance-dependent label noise by exploiting \textit{parts-dependent} label noise. Specifically, since instances can be approximately reconstructed by a combination of parts, we approximate the instance-dependent \textit{transition matrix} for an instance by a combination of the transition matrices for the parts of the instance. The transition matrices for parts can be learned by exploiting anchor points (i.e., data points that belong to a specific class almost surely). Empirical evaluations on synthetic and real-world datasets demonstrate our method is superior to the state-of-the-art approaches for learning from the instance-dependent label noise.
更多查看译文
关键词
label,noise,parts-dependent,instance-dependent
AI 理解论文
溯源树
样例
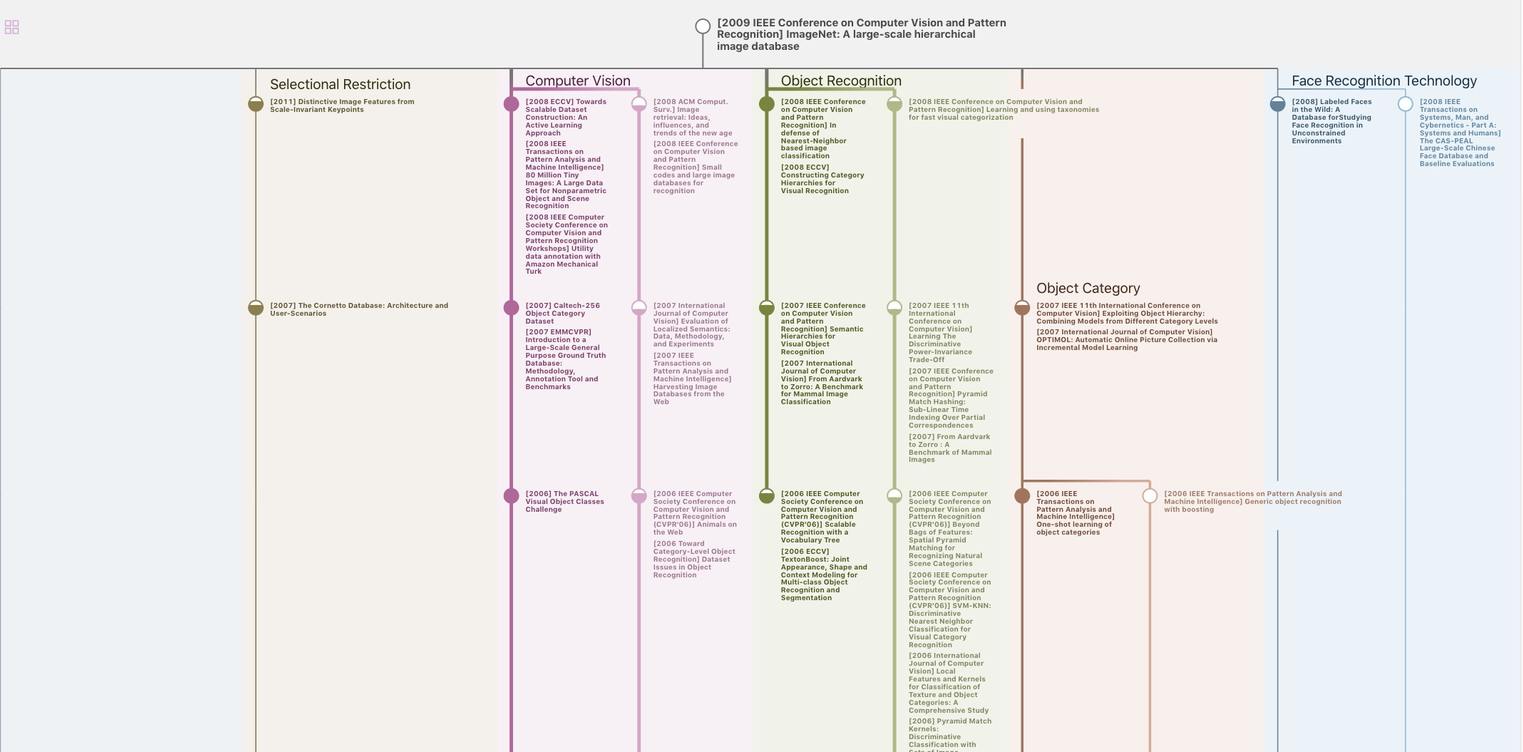
生成溯源树,研究论文发展脉络
Chat Paper
正在生成论文摘要