ShapeFlow: Learnable Deformation Flows Among 3D Shapes
arxiv(2020)
摘要
We present ShapeFlow, a flow-based model for learning a deformation space for entire classes of 3D shapes with large intra-class variations. ShapeFlow allows learning a multi-template deformation space that is agnostic to shape topology, yet preserves fine geometric details. Different from a generative space where a latent vector is directly decoded into a shape, a deformation space decodes a vector into a continuous flow that can advect a source shape towards a target. Such a space naturally allows the disentanglement of geometric style (coming from the source) and structural pose (conforming to the target). We parametrize the deformation between geometries as a learned continuous flow field via a neural network and show that such deformations can be guaranteed to have desirable properties, such as bijectivity, freedom from self-intersections, or volume preservation. We illustrate the effectiveness of this learned deformation space for various downstream applications, including shape generation via deformation, geometric style transfer, unsupervised learning of a consistent parameterization for entire classes of shapes, and shape interpolation.
更多查看译文
关键词
learnable deformation,3d shapes,shapeflow
AI 理解论文
溯源树
样例
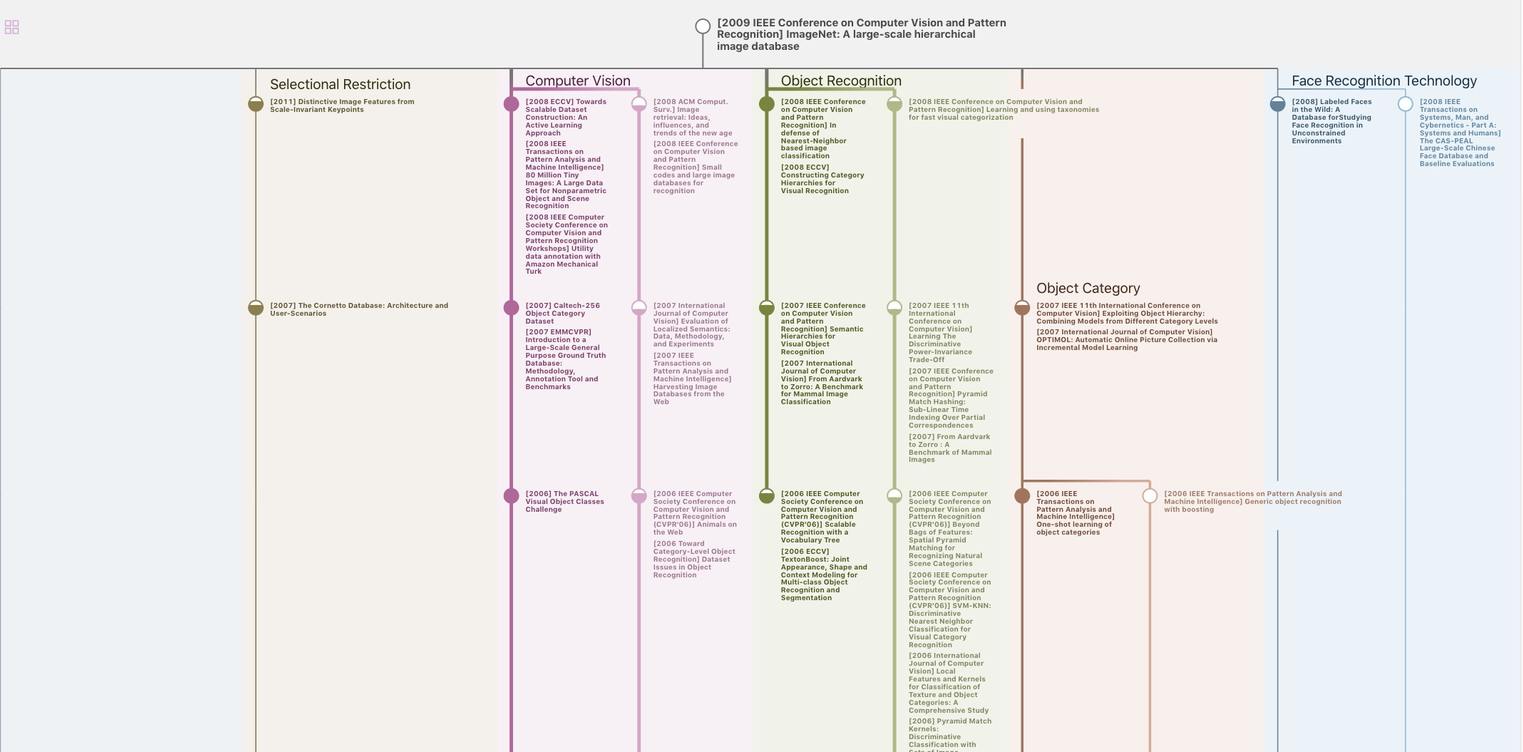
生成溯源树,研究论文发展脉络
Chat Paper
正在生成论文摘要