Manifold-Regularized Regression Network: A Novel End-to-End Method for Cell Counting and Localization
ACM Proceedings(2020)
摘要
Biomedical image analysis by machine learning enables accurate cell counting and localization, which are essential to clinic diagnosis and research. However, high-density population of the cells poses great challenges. The variability and intricacy in the shapes and colours of cells further jeopardize the performance of existing models. Aiming to overcome the difficulties, we propose an end-to-end manifold-regularized regression network (MRRN) for cell counting and localization. The backbone is a U-Net tweaked for regression and trained by density maps to preserve the spatial distribution of the cells. Based on graph Laplacian, the patch-wise manifold regularizer imposed on the U-Net can represent the intrinsically nonlinear and complex features of the images, thus increasing the generalizability of the network and lowering the risk of overfitting. Experimental results show that MRRN outperforms several state-of-the-art algorithms on a widely-adopted synthetic data set and a real-world carcinoma data set, which verifies the efficacy and applicability of the proposed model.
更多查看译文
关键词
Cellular Imaging,Medical Image Analysis,Bioimage Analysis
AI 理解论文
溯源树
样例
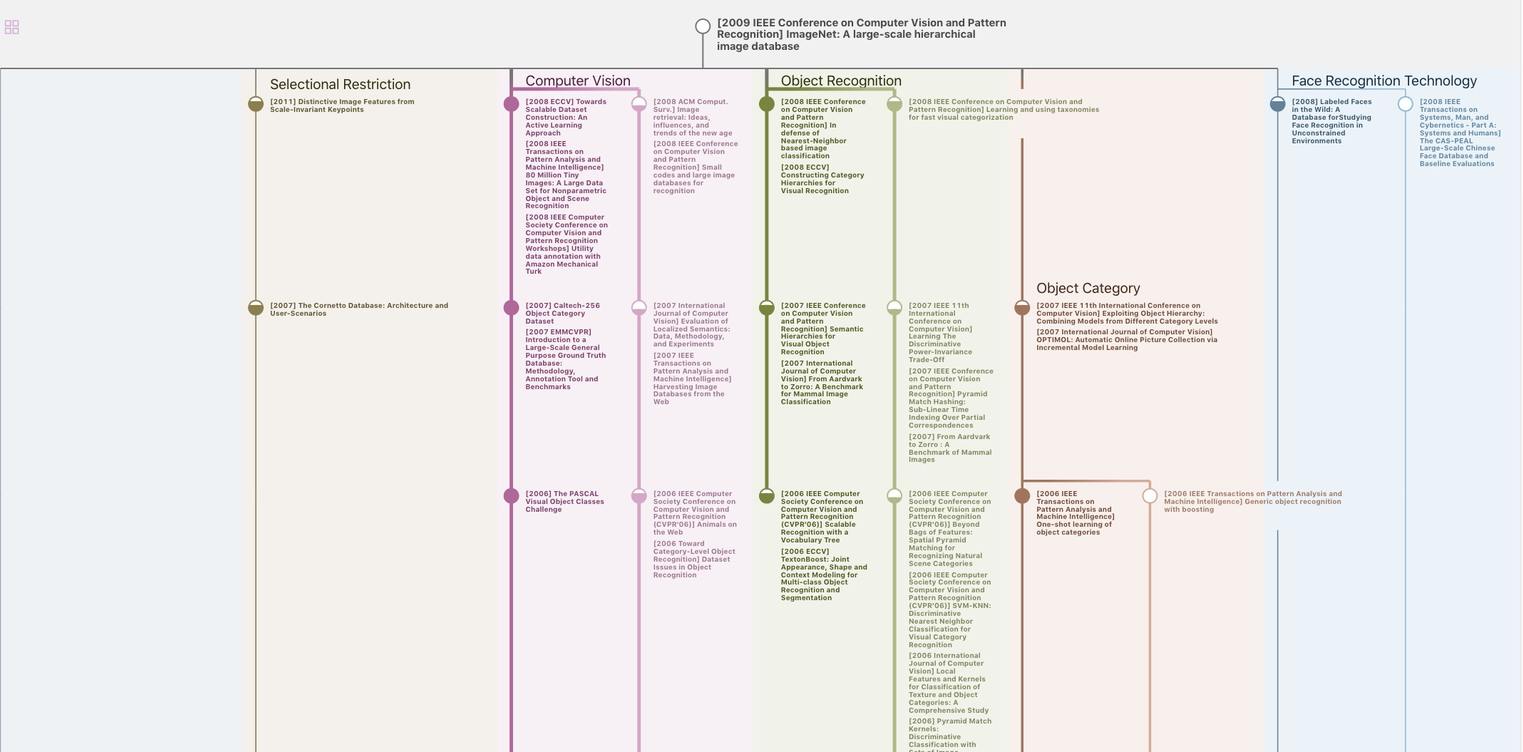
生成溯源树,研究论文发展脉络
Chat Paper
正在生成论文摘要