Multipoint Channel Charting With Multiple-Input Multiple-Output Convolutional Autoencoder.
PLANS(2020)
摘要
We study the multipoint channel charting problem, where the channel state information (CSI) from multiple bases is used to generate channel charts for user relative positioning and many other applications. In previous work, only non-parametric methods are considered. In this paper, we fill the gap by proposing a novel neural network architecture - a multiple-input multiple-output (MIMO) convolutional autoencoder (CAE) - to solve the problem. Based on an open-source dataset, we demonstrate that for the use cases of user relative positioning and in region location verification (IRLV), compared with a baseline autoencoder (AE) with all fully-connected layers, the proposed network is able to achieve similar or better performance with a much smaller network size. In addition, we note that the proposed network is more capable of extracting useful features from CSI data and thus more promising for end-to-end learning.
更多查看译文
关键词
channel state information,multiple bases,channel charts,user relative positioning,nonparametric methods,multiple-input multiple-output convolutional autoencoder,baseline autoencoder,smaller network size,multipoint channel charting problem,CAE,neural network architecture,MIMO
AI 理解论文
溯源树
样例
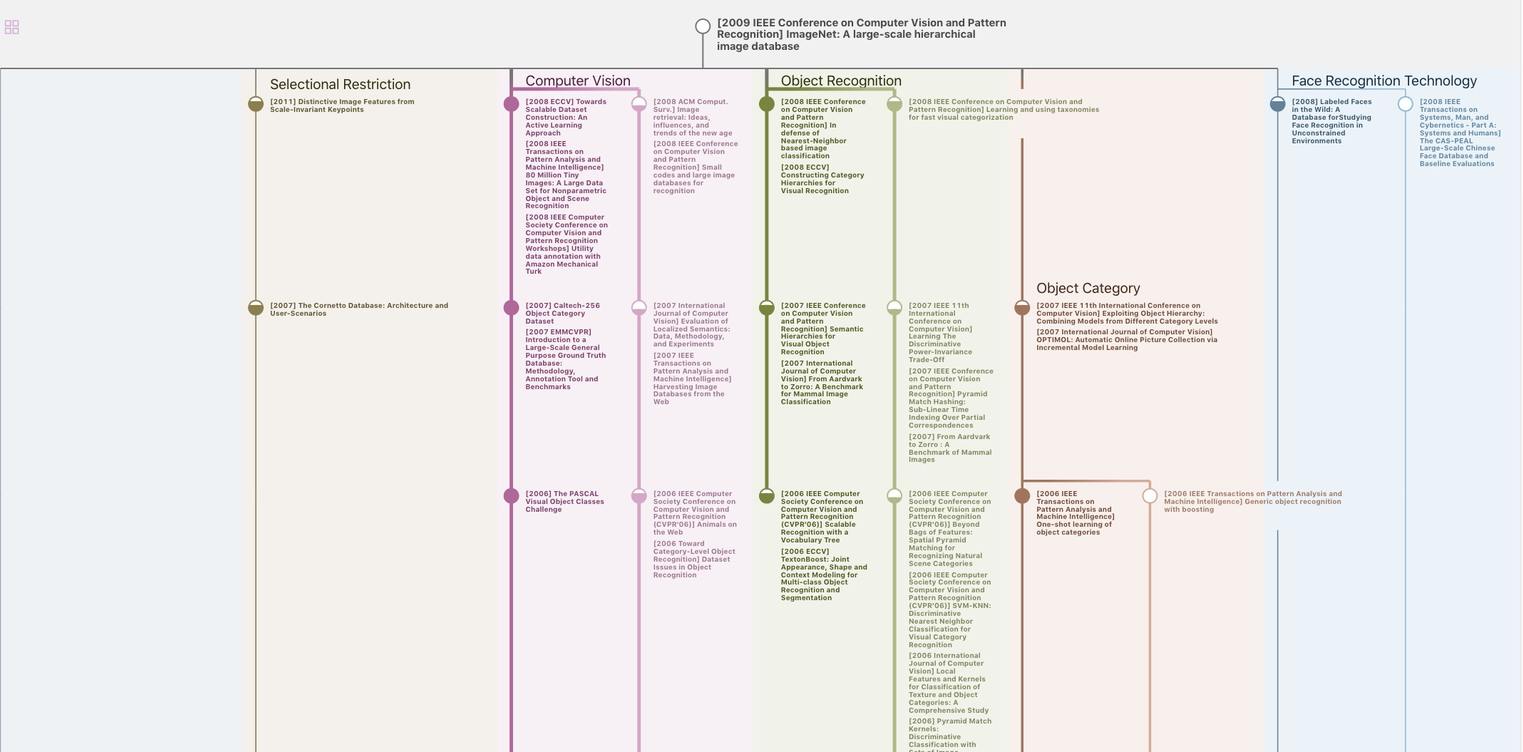
生成溯源树,研究论文发展脉络
Chat Paper
正在生成论文摘要