Comparison of Statistical Modelling Approaches for Estimating Tropical Forest Aboveground Biomass Stock and Reporting Their Changes in Low-Intensity Logging Areas Using Multi-Temporal LiDAR Data.
REMOTE SENSING(2020)
摘要
Accurately quantifying forest aboveground biomass (AGB) is one of the most significant challenges in remote sensing, and is critical for understanding global carbon sequestration. Here, we evaluate the effectiveness of airborne LiDAR (Light Detection and Ranging) for monitoring AGB stocks and change (Delta AGB) in a selectively logged tropical forest in eastern Amazonia. Specifically, we compare results from a suite of different modelling methods with extensive field data. The calibration AGB values were derived from 85 square field plots sized 50 x 50 m field plots established in 2014 and which were estimated using airborne LiDAR data acquired in 2012, 2014, and 2017. LiDAR-derived metrics were selected based upon Principal Component Analysis (PCA) and used to estimate AGB stock and change. The statistical approaches were: ordinary least squares regression (OLS), and nine machine learning approaches: random forest (RF), several variations of k-nearest neighbour (k-NN), support vector machine (SVM), and artificial neural networks (ANN). Leave-one-out cross-validation (LOOCV) was used to compare performance based upon root mean square error (RMSE) and mean difference (MD). The results show that OLS had the best performance with an RMSE of 46.94 Mg/ha (19.7%) and R-2 = 0.70. RF, SVM, and ANN were adequate, and all approaches showed RMSE <= 54.48 Mg/ha (22.89%). Models derived from k-NN variations all showed RMSE >= 64.61 Mg/ha (27.09%). The OLS model was thus selected to map AGB across the time-series. The mean (+/- sd-standard deviation) predicted AGB stock at the landscape level was 229.10 (+/- 232.13) Mg/ha in 2012, 258.18 (+/- 106.53) in 2014, and 240.34 (sd +/- 177.00) Mg/ha in 2017, showing the effect of forest growth in the first period and logging in the second period. In most cases, unlogged areas showed higher AGB stocks than logged areas. Our methods showed an increase in AGB in unlogged areas and detected small changes from reduced-impact logging (RIL) activities occurring after 2012. We also detected that the AGB increase in areas logged before 2012 was higher than in unlogged areas. Based on our findings, we expect our study could serve as a basis for programs such as REDD+ and assist in detecting and understanding AGB changes caused by selective logging activities in tropical forests.
更多查看译文
关键词
Amazon,forest structure,remote sensing,modelling,mapping
AI 理解论文
溯源树
样例
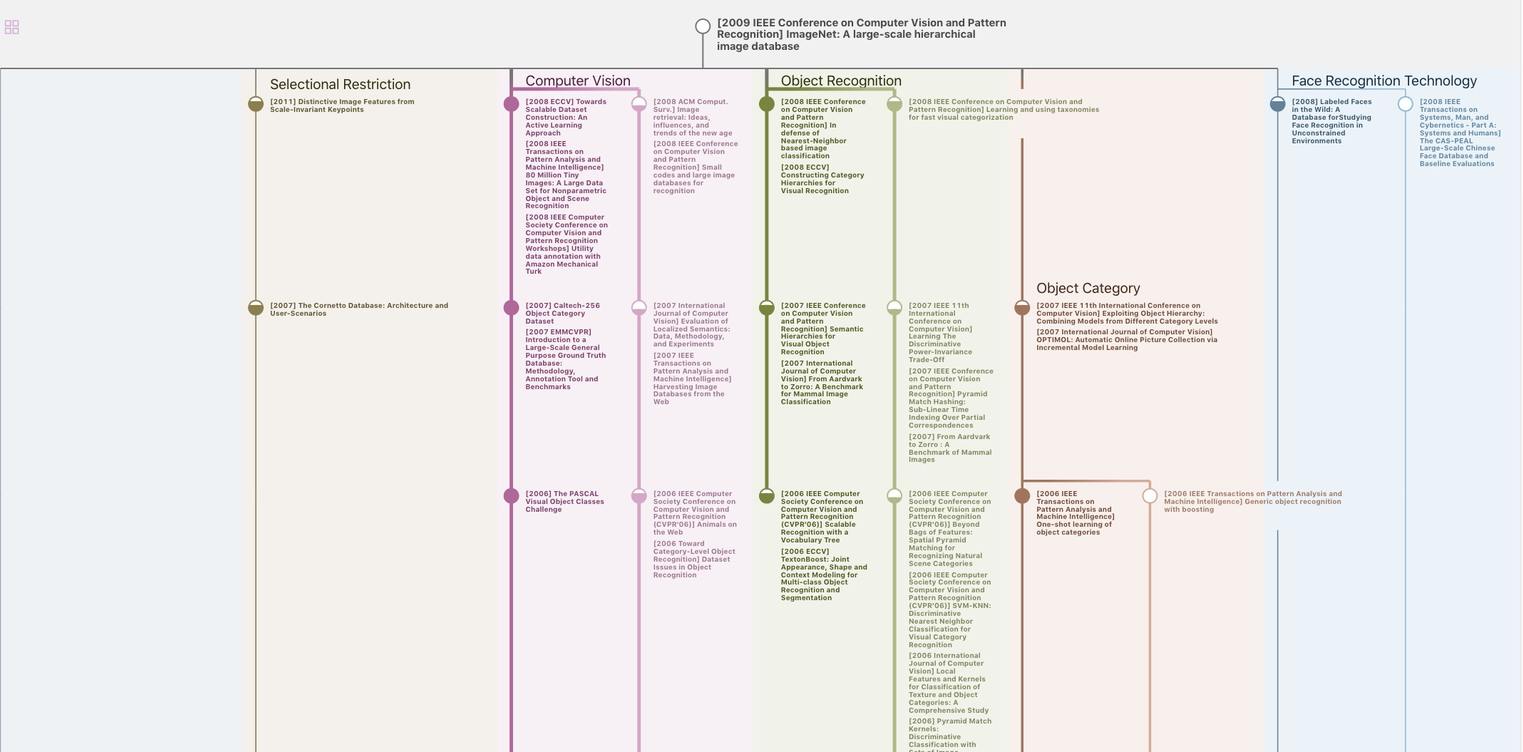
生成溯源树,研究论文发展脉络
Chat Paper
正在生成论文摘要