Identifiability of infection model parameters early in an epidemic.
biorxiv(2022)
摘要
It is known that the parameters in the deterministic and stochastic SEIR epidemic models are structurally identifiable. For example, from knowledge of the infected population time series () during the entire epidemic, the parameters can be successfully estimated. In this article we observe that estimation will fail in practice if only infected case data during the early part of the epidemic (prepeak) is available. This fact can be explained using a well-known phenomenon called dynamical compensation. We use this concept to derive an unidentifiability manifold in the parameter space of SEIR that consists of parameters indistinguishable from () early in the epidemic. Thus, identifiability depends on the extent of the system trajectory that is available for observation. Although the existence of the unidentifiability manifold obstructs the ability to exactly determine the parameters, we suggest that it may be useful for uncertainty quantification purposes. A variant of SEIR recently proposed for COVID-19 modeling is also analyzed, and an analogous unidentifiability surface is derived.
更多查看译文
关键词
92C60,92D30,SEIR models,epidemic models,identifiability
AI 理解论文
溯源树
样例
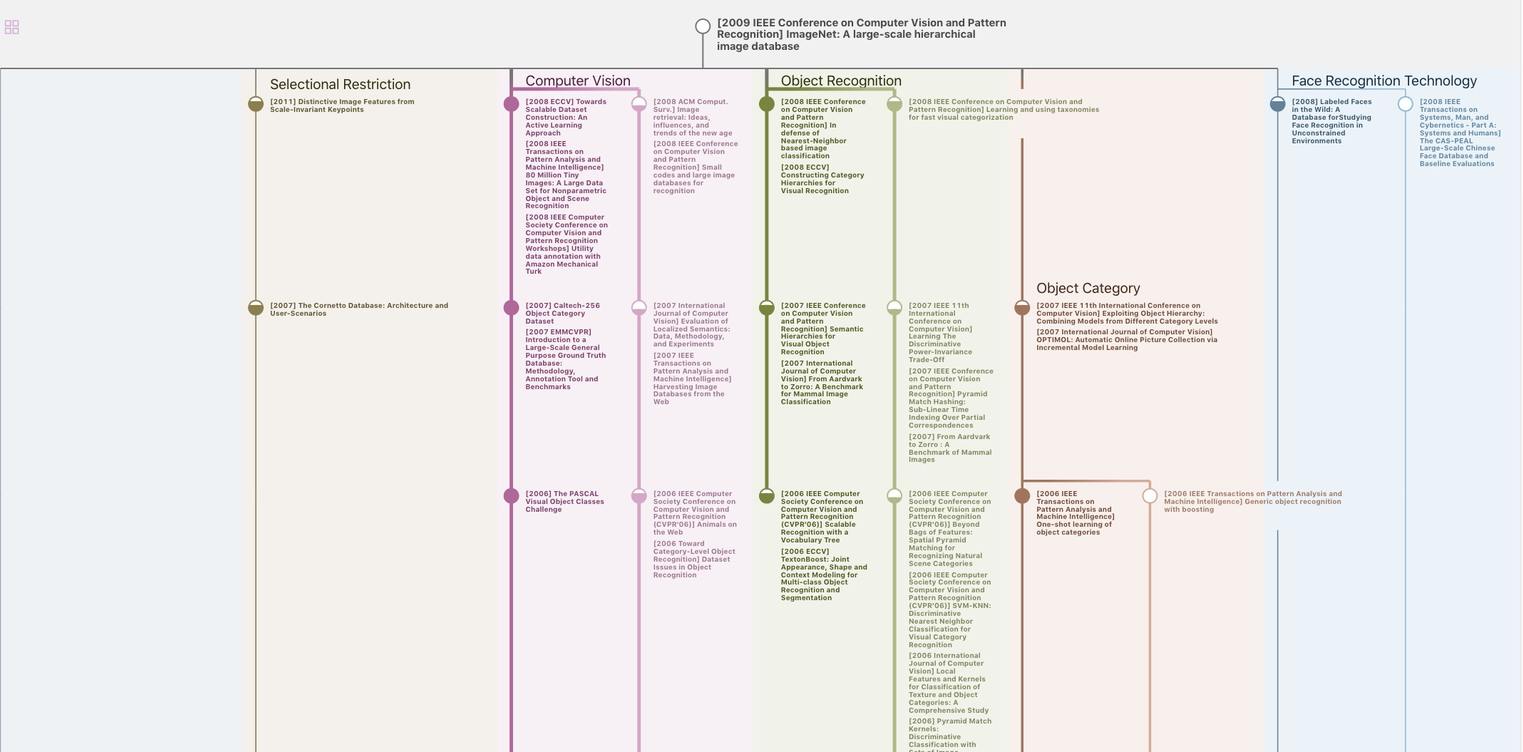
生成溯源树,研究论文发展脉络
Chat Paper
正在生成论文摘要