Cross-Correlated Attention Networks for Person Re-Identification
Image and Vision Computing(2020)
摘要
Deep neural networks need to make robust inference in the presence of occlusion, background clutter, pose and viewpoint variations -to name a few- when the task of person re-identification is considered. Attention mechanisms have recently proven to be successful in handling the aforementioned challenges to some degree. However previous designs fail to capture inherent inter-dependencies between the attended features; leading to restricted interactions between the attention blocks. In this paper, we propose a new attention module called Cross-Correlated Attention (CCA); which aims to overcome such limitations by maximizing the information gain between different attended regions. Moreover, we also propose a novel deep network that makes use of different attention mechanisms to learn robust and discriminative representations of person images. The resulting model is called the Cross-Correlated Attention Network (CCAN). Extensive experiments demonstrate that the CCAN comfortably outperforms current state-of-the-art algorithms by a tangible margin.
更多查看译文
关键词
Attention,Feature extraction,Cross correlation,Person Re-Identification,Surveillance
AI 理解论文
溯源树
样例
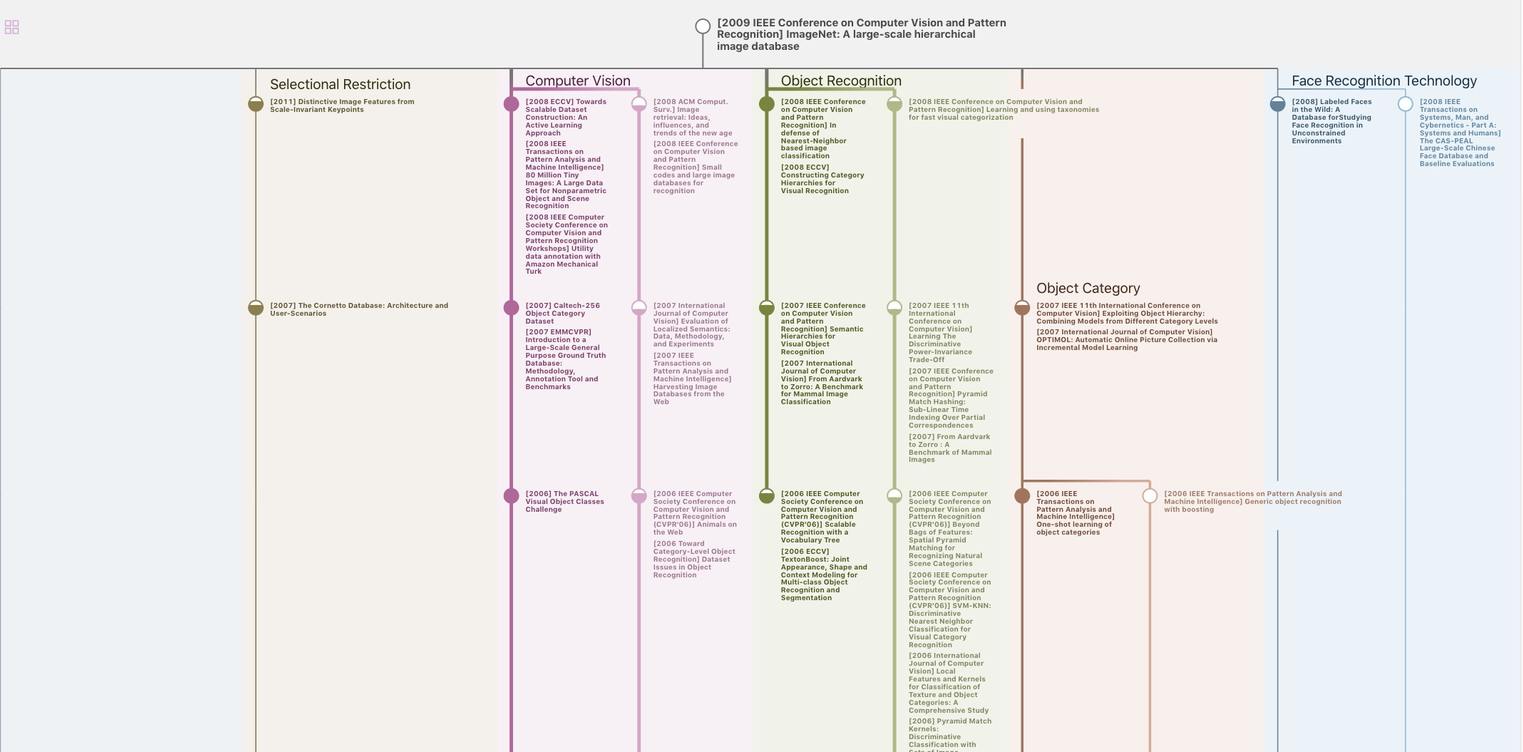
生成溯源树,研究论文发展脉络
Chat Paper
正在生成论文摘要