Learning a functional control for high-frequency finance
QUANTITATIVE FINANCE(2022)
摘要
We use a deep neural network to generate controllers for optimal trading on high-frequency data. For the first time, a neural network learns the mapping between the preferences of the trader, i.e. risk aversion parameters, and the optimal controls. An important challenge in learning this mapping is that, in intra-day trading, trader's actions influence price dynamics in closed loop via the market impact. The exploration-exploitation tradeoff generated by the efficient execution is addressed by tuning the trader's preferences to ensure long enough trajectories are produced during the learning phase. The issue of scarcity of financial data is solved by transfer learning: the neural network is first trained on trajectories generated thanks to a Monte-Carlo scheme, leading to a good initialization before training on historical trajectories. Moreover, to answer to genuine requests of financial regulators on the explainability of machine learning generated controls, we project the obtained 'blackbox controls' on the space usually spanned by the closed-form solution of the stylized optimal trading problem, leading to a transparent structure. For more realistic loss functions that have no closed-form solution, we show that the average distance between the generated controls and their explainable version remains small. This opens the door to the acceptance of ML-generated controls by financial regulators.
更多查看译文
关键词
Market microstructure, Optimal execution, Neural networks, Quantitative finance, Stochastic optimization, Optimal control
AI 理解论文
溯源树
样例
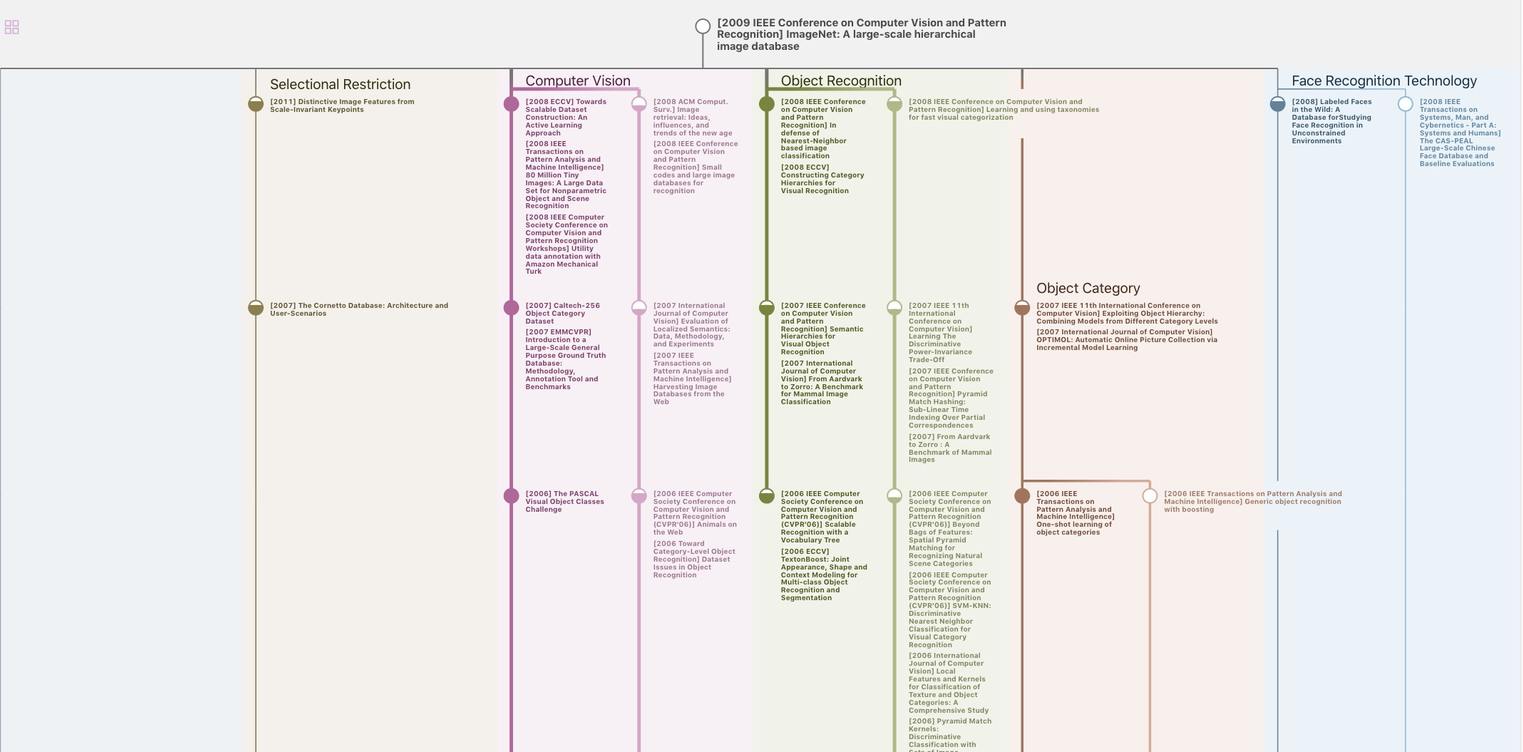
生成溯源树,研究论文发展脉络
Chat Paper
正在生成论文摘要