Directional Multivariate Ranking
KDD '20: The 26th ACM SIGKDD Conference on Knowledge Discovery and Data Mining Virtual Event CA USA July, 2020(2020)
摘要
User-provided multi-aspect evaluations manifest users' detailed feedback on the recommended items and enable fine-grained understanding of their preferences. Extensive studies have shown that modeling such data greatly improves the effectiveness and explainability of the recommendations. However, as ranking is essential in recommendation, there is no principled solution yet for collectively generating multiple item rankings over different aspects.
In this work, we propose a directional multi-aspect ranking criterion to enable a holistic ranking of items with respect to multiple aspects. Specifically, we view multi-aspect evaluation as an integral effort from a user that forms a vector of his/her preferences over aspects. Our key insight is that the direction of the difference vector between two multi-aspect preference vectors reveals the pairwise order of comparison. Hence, it is necessary for a multi-aspect ranking criterion to preserve the observed directions from such pairwise comparisons. We further derive a complete solution for the multi-aspect ranking problem based on a probabilistic multivariate tensor factorization model. Comprehensive experimental analysis on a large TripAdvisor multi-aspect rating dataset and a Yelp review text dataset confirms the effectiveness of our solution.
更多查看译文
关键词
multi-aspect ranking, directional statistics
AI 理解论文
溯源树
样例
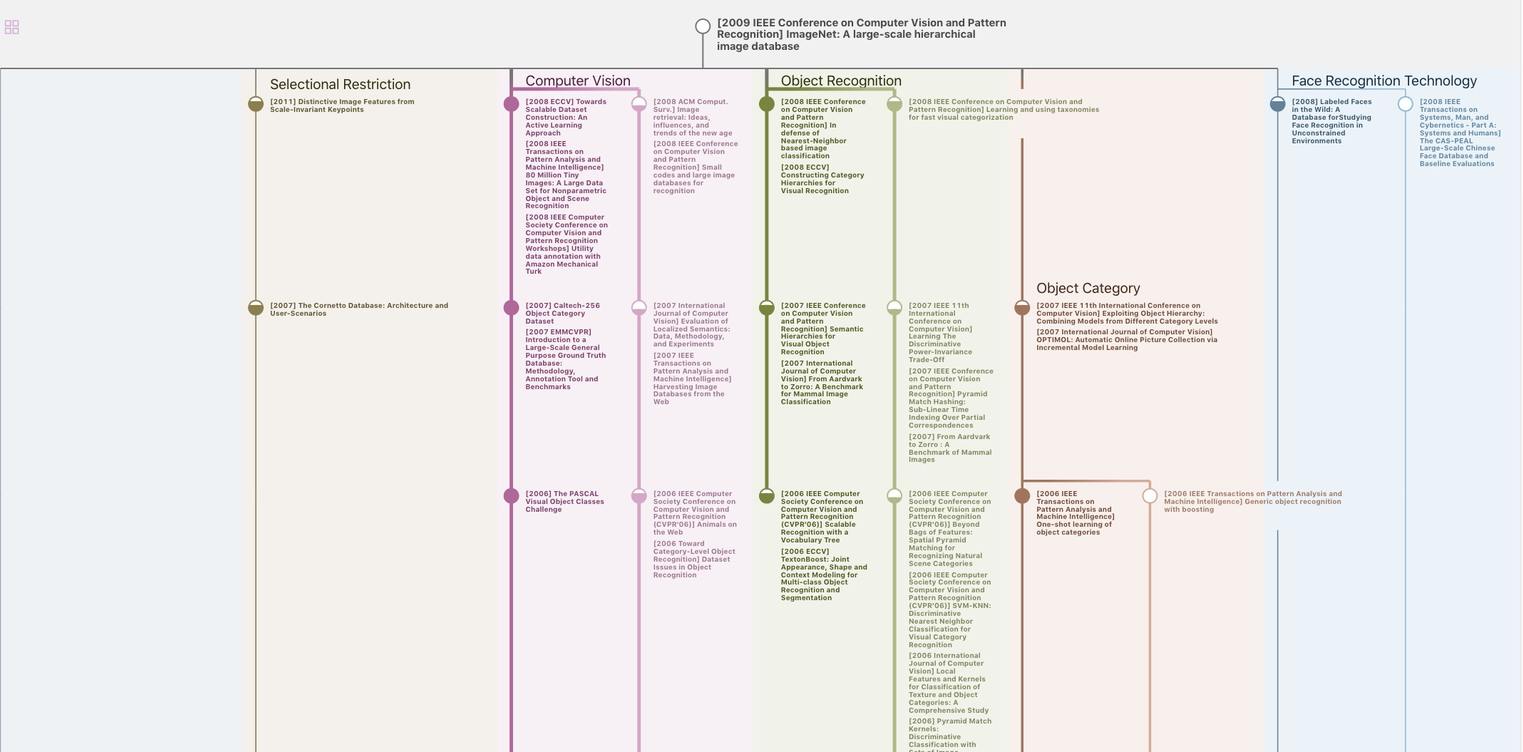
生成溯源树,研究论文发展脉络
Chat Paper
正在生成论文摘要