Deep Encoder, Shallow Decoder: Reevaluating the Speed-Quality Tradeoff in Machine Translation
arxiv(2020)
摘要
State-of-the-art neural machine translation models generate outputs autoregressively, where every step conditions on the previously generated tokens. This sequential nature causes inherent decoding latency. Non-autoregressive translation techniques, on the other hand, parallelize generation across positions and speed up inference at the expense of translation quality. Much recent effort has been devoted to non-autoregressive methods, aiming for a better balance between speed and quality. In this work, we re-examine the trade-off and argue that transformer-based autoregressive models can be substantially sped up without loss in accuracy. Specifically, we study autoregressive models with encoders and decoders of varied depths. Our extensive experiments show that given a sufficiently deep encoder, a one-layer autoregressive decoder yields state-of-the-art accuracy with comparable latency to strong non-autoregressive models. Our findings suggest that the latency disadvantage for autoregressive translation has been overestimated due to a suboptimal choice of layer allocation, and we provide a new speed-quality baseline for future research toward fast, accurate translation.
更多查看译文
关键词
deep encoder,shallow decoder,translation,speed-quality
AI 理解论文
溯源树
样例
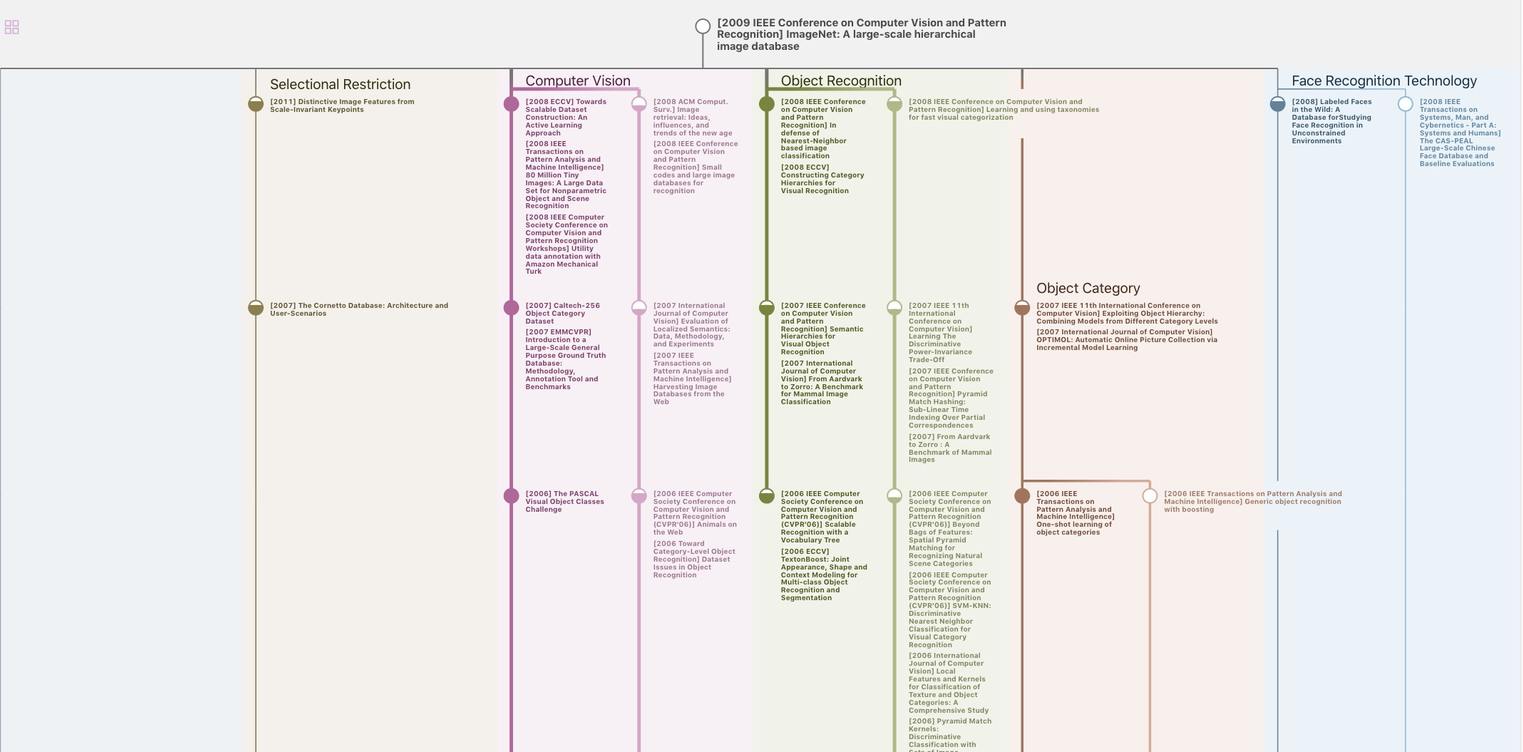
生成溯源树,研究论文发展脉络
Chat Paper
正在生成论文摘要