Compositional Generalization by Learning Analytical Expressions
NIPS 2020(2020)
摘要
Compositional generalization is a basic but essential intellective capability of human beings, which allows us to recombine known parts readily. However, existing neural network based models have been proven to be extremely deficient in such a capability. Inspired by work in cognition which argues compositionality can be captured by variable slots with symbolic functions, we present a refreshing view that connects a memory-augmented neural model with analytical expressions, to achieve compositional generalization. Our model consists of two cooperative neural modules Composer and Solver, fitting well with the cognitive argument while still being trained in an end-to-end manner via a hierarchical reinforcement learning algorithm. Experiments on a well-known benchmark SCAN demonstrate that our model seizes a great ability of compositional generalization, solving all challenges addressed by previous works with 100% accuracies.
更多查看译文
关键词
compositional generalization,expressions,learning
AI 理解论文
溯源树
样例
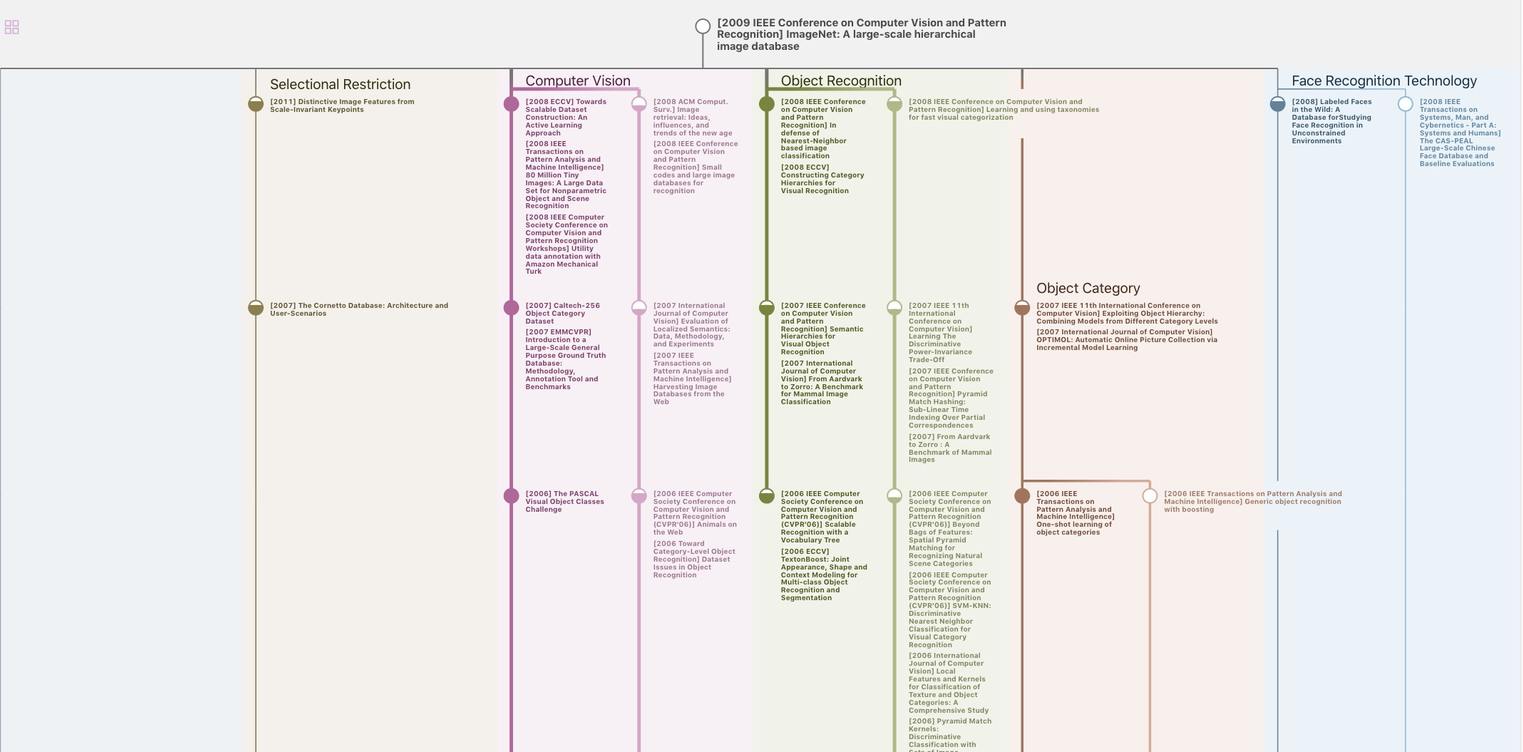
生成溯源树,研究论文发展脉络
Chat Paper
正在生成论文摘要