Groundwater contamination sources identification based on kernel extreme learning machine and its effect due to wavelet denoising technique
ENVIRONMENTAL SCIENCE AND POLLUTION RESEARCH(2020)
摘要
Measurements of contaminant concentrations inevitably contain noise because of accidental and systematic errors. However, groundwater contamination sources identification (GCSI) is highly dependent on the data measurements, which directly affect the accuracy of the identification results. Thus, in the present study, the wavelet hierarchical threshold denoising method was employed to denoise concentration measurements and the denoised measurements were then used for GCSI. A 0–1 mixed-integer nonlinear programming optimization model (0–1 MINLP) based on a kernel extreme learning machine (KELM) was applied to identify the location and release history of a contamination source. The results showed the following. (1) The wavelet hierarchical threshold denoising method was not very effective when applied to concentration measurements observed every 2 months (the number of measurements is small and relatively discrete) compared with those obtained every 2 days (the number of measurements is large and relatively continuous). (2) When the concentration measurements containing noise were employed for GCSI, the identifications results were further from the true values when the measurements contained more noise. The approximation of the identification results to the true values improved when the denoised concentration measurements were employed for GCSI. (3) The 0–1 MINLP based on the surrogate KELM model could simultaneously identify the location and release history of contamination sources, as well reducing the computational load and decreasing the calculation time by 96.5% when solving the 0–1 MINLP.
更多查看译文
关键词
Groundwater contamination,Kernel extreme learning machine,Wavelet hierarchical threshold denoising
AI 理解论文
溯源树
样例
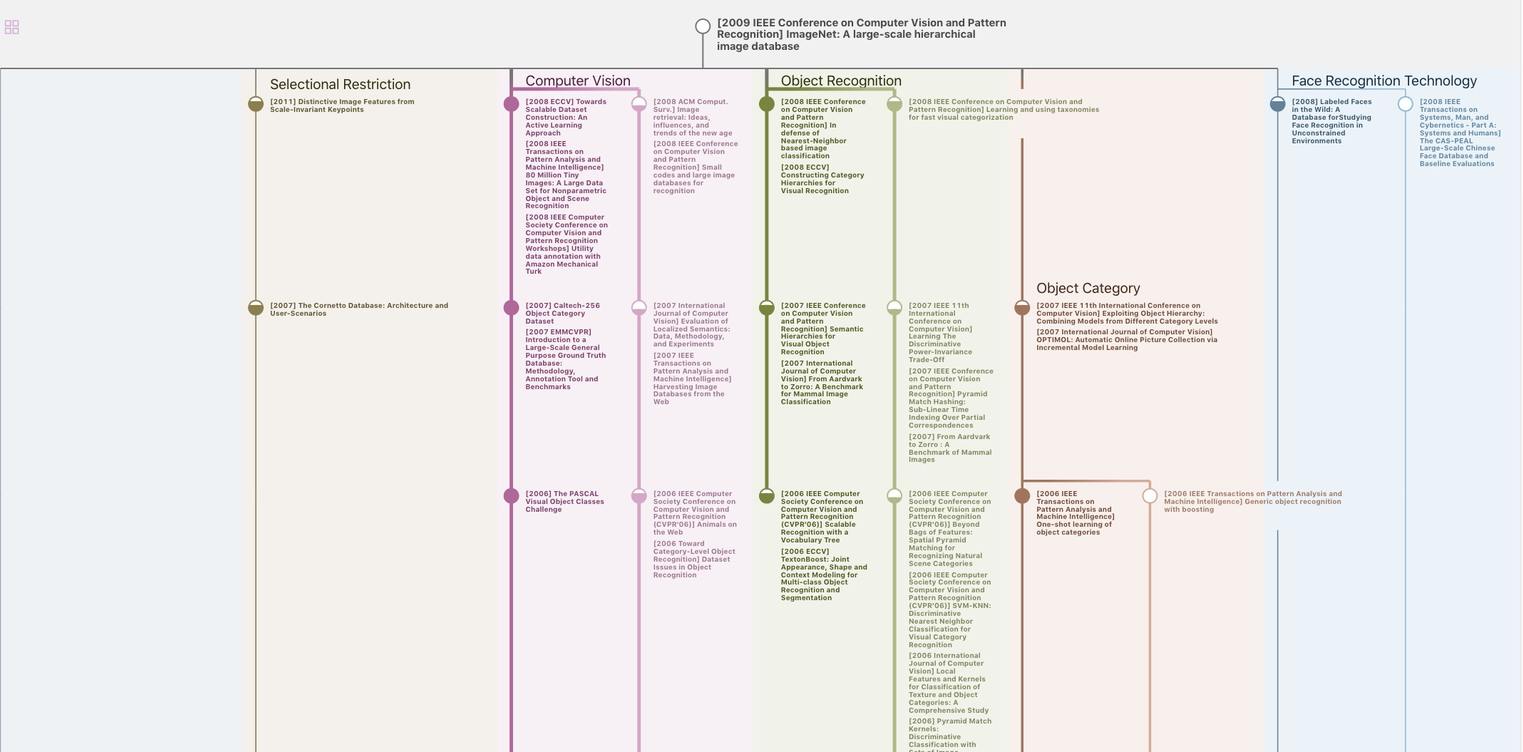
生成溯源树,研究论文发展脉络
Chat Paper
正在生成论文摘要