How does this interaction affect me? Interpretable attribution for feature interactions
NIPS 2020(2020)
摘要
Machine learning transparency calls for interpretable explanations of how inputs relate to predictions. Feature attribution is a way to analyze the impact of features on predictions. Feature interactions are the contextual dependence between features that jointly impact predictions. There are a number of methods that extract feature interactions in prediction models; however, the methods that assign attributions to interactions are either uninterpretable, model-specific, or non-axiomatic. We propose an interaction attribution and detection framework called Archipelago which addresses these problems and is also scalable in real-world settings. Our experiments on standard annotation labels indicate our approach provides significantly more interpretable explanations than comparable methods, which is important for analyzing the impact of interactions on predictions. We also provide accompanying visualizations of our approach that give new insights into deep neural networks.
更多查看译文
关键词
interpretable attribution,feature interactions
AI 理解论文
溯源树
样例
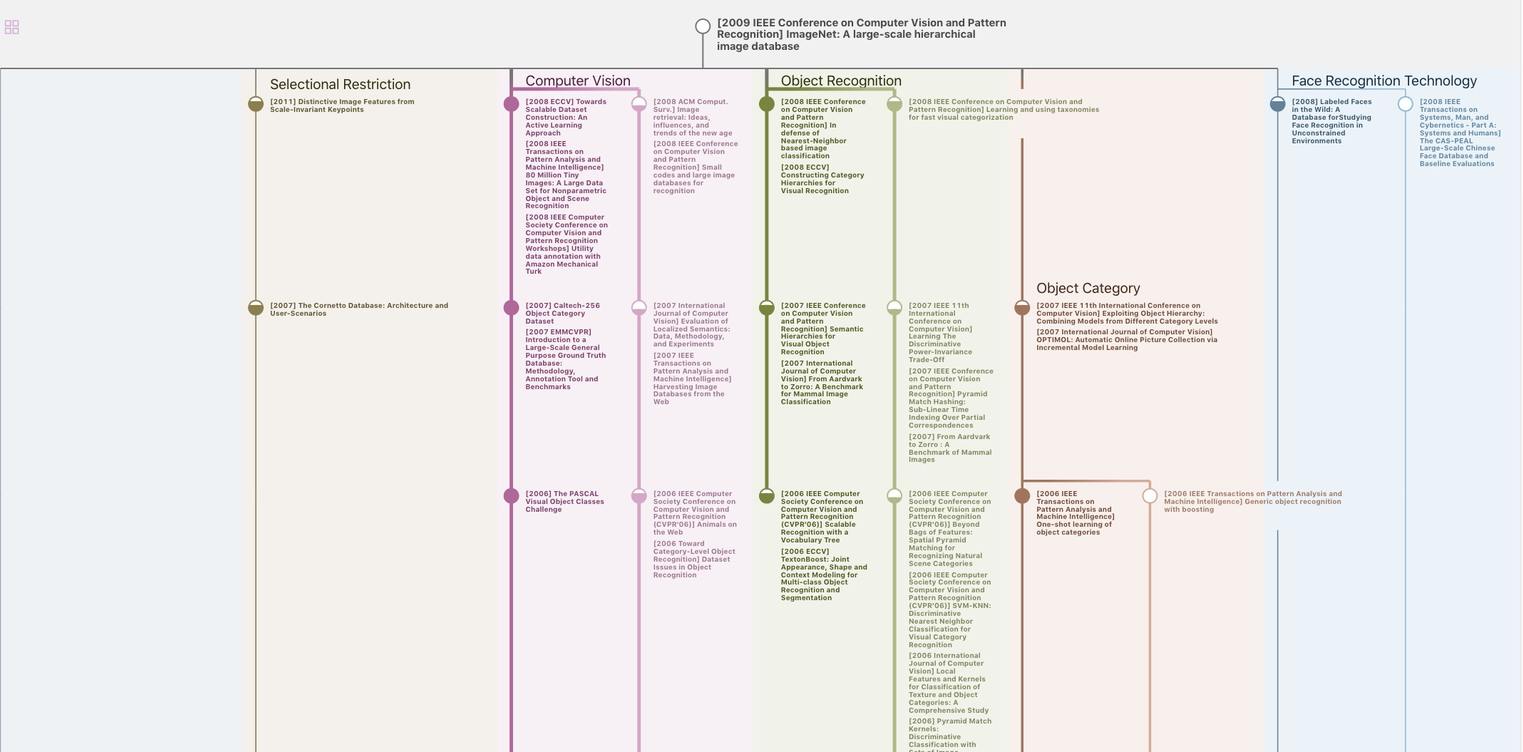
生成溯源树,研究论文发展脉络
Chat Paper
正在生成论文摘要