NROWAN-DQN: A stable noisy network with noise reduction and online weight adjustment for exploration
Expert Systems with Applications(2022)
摘要
Deep reinforcement learning has been applied more and more widely nowadays, especially in various complex control tasks. Noise is vital to reinforcement learning exploration. The appropriate noise not only avoids local optimum solutions due to the lack of exploration, but also prevents unstable learning caused by excessive perturbations. Noisy networks can bring more efficient exploration to reinforcement learning. They enable agents to take actions more randomly at the start of training and tend to make the agents produce stable outputs in the subsequent learning. However, this tendency is not always enough to find a stable policy for an agent, which decreases the efficiency and stability. To address this issue, this paper proposes NROWAN-DQN, i.e., Noise Reduction and Online Weight Adjustment NoisyNet-DQN. Firstly, we develop a novel noise regularization method for NoisyNet-DQN to reduce the noise of output layers. Secondly, we design an online weight adjustment strategy for noise reduction. Finally, we evaluate this algorithm in four standard domains and analyze properties of hyper-parameters. Our results show that NROWAN-DQN outperforms prior algorithms in all these domains. In addition, NROWAN-DQN also shows better stability. The variance of the NROWAN-DQN score is significantly reduced, especially in some action-sensitive environments. This means that in some environments where high stability is required, NROWAN-DQN will be more appropriate than NoisyNet-DQN.
更多查看译文
关键词
Deep reinforcement learning,Exploration,Noisy networks,Noise reduction,Online weight adjustment
AI 理解论文
溯源树
样例
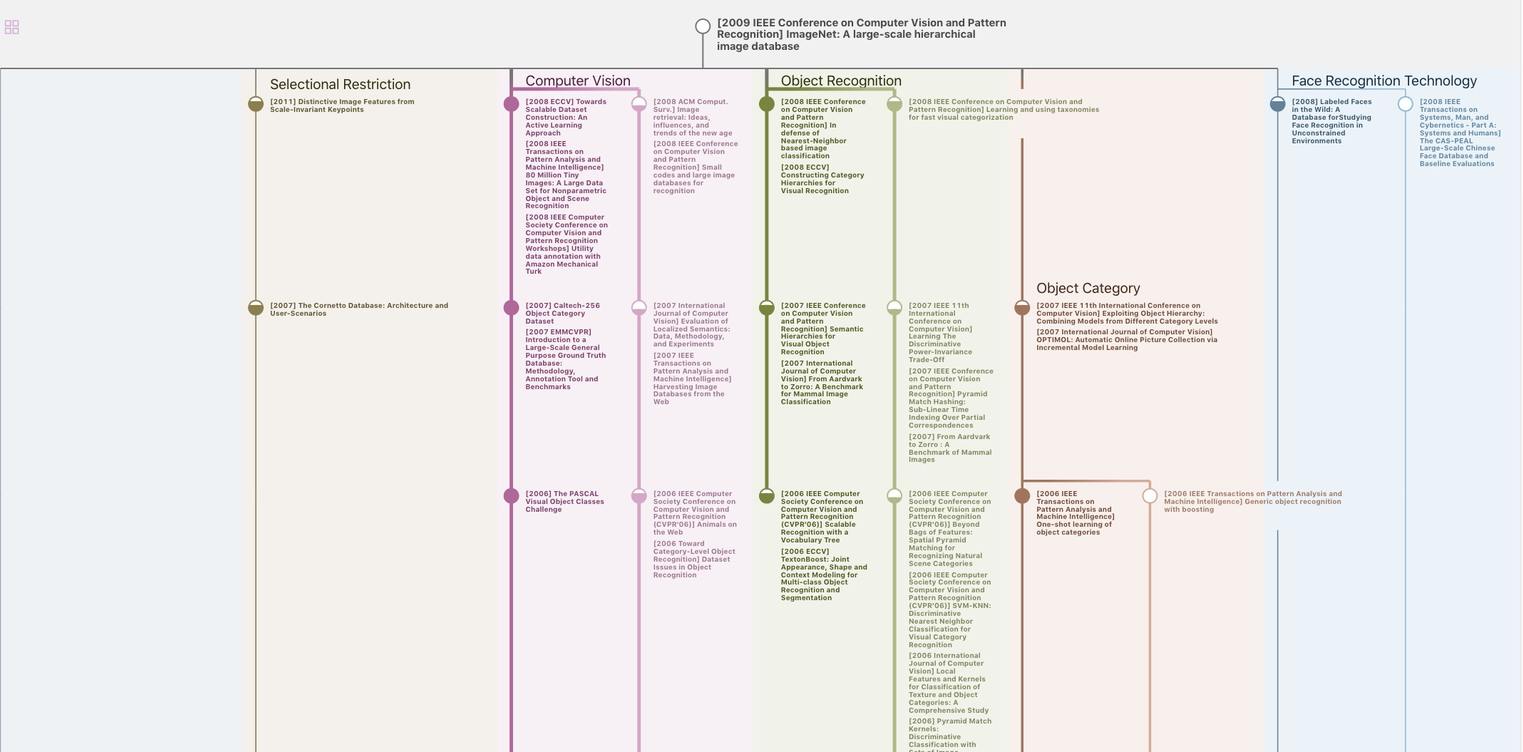
生成溯源树,研究论文发展脉络
Chat Paper
正在生成论文摘要