Wildlife Poaching Forecasting Based on Ranger-Collected Data and Evaluation through Field Tests
Artificial Intelligence and Conservation(2019)
摘要
Wildlife poaching continues to be a global problem as key species are hunted toward extinction. For example, the latest African census showed a 30 percent decline in elephant populations between 2007 and 2014 (Convention on International Trade in Endangered Species of Wild Fauna and Flora 2016; Great Elephant Census 2016). Wildlife conservation areas have been established to protect these species from poachers, and these areas are protected by park rangers. These areas are vast, and rangers do not have sufficient resources to patrol everywhere with high intensity and frequency. At many sites now, rangers patrol the protected areas and collect data related to snares they confiscate, poachers they arrest, and other observations. Given rangers’ resource constraints, patrol managers could benefit from tools that analyze these data and provide future poaching predictions. However, this domain presents unique challenges. First, this domain’s real-world data are few, extremely noisy, and incomplete. To illustrate, one of the rangers’ primary patrol goals is to find wire snares, which are deployed by poachers to catch animals. However, these snares are usually well-hidden (eg, in dense grass), and thus rangers may not find them and so (incorrectly) label an area as not having any snares. Second, poaching activity changes over time, and predictive models must account for this temporal component. Third, because poaching happens in the real world, there are mutual spatial and neighborhood effects that influence poaching activity. Finally, while field tests are crucial in determining a model’s efficacy in the world, the difficulties involved in …
更多查看译文
AI 理解论文
溯源树
样例
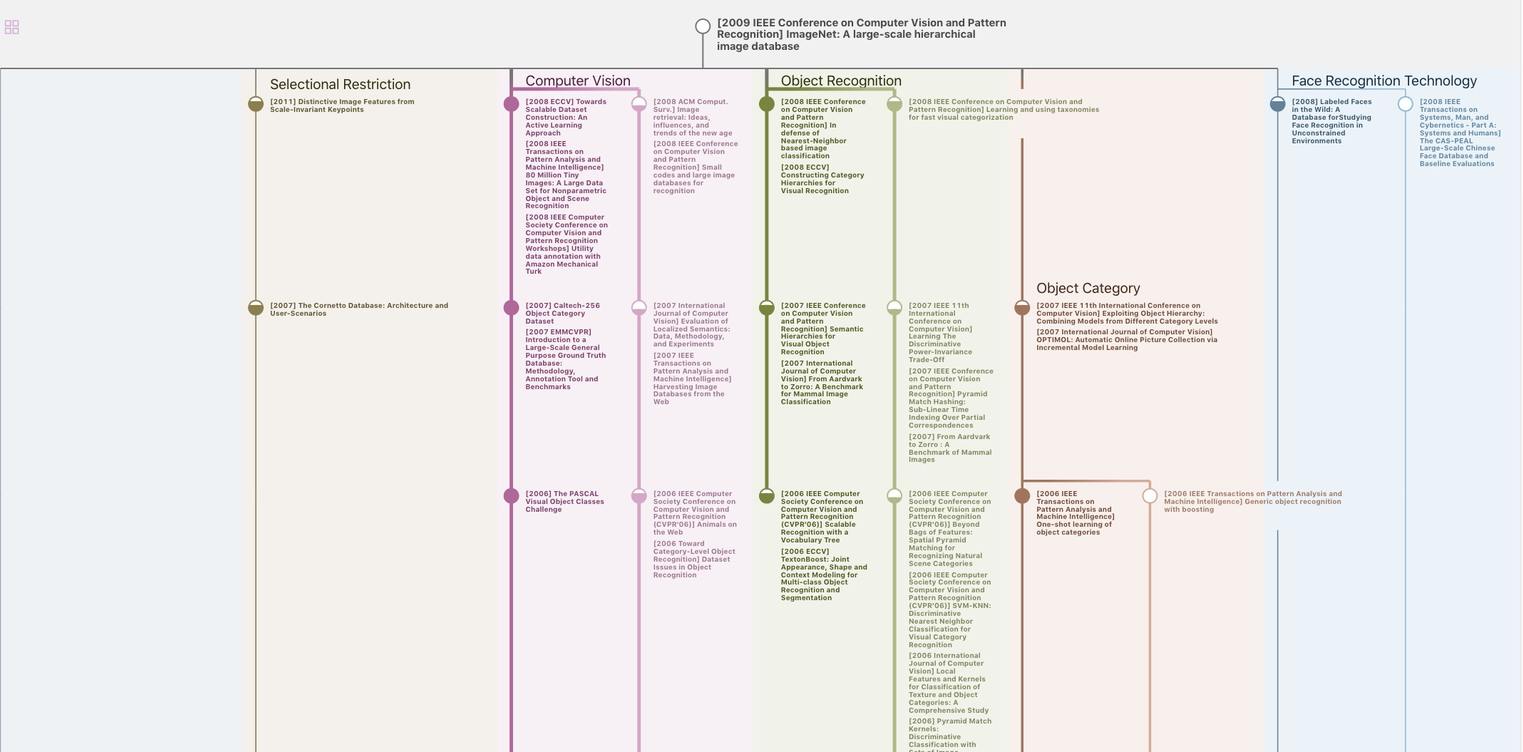
生成溯源树,研究论文发展脉络
Chat Paper
正在生成论文摘要