Spatio-Temporal Deep Residual Network with Hierarchical Attentions for Video Event Recognition
ACM Transactions on Multimedia Computing, Communications, and Applications(2020)
摘要
Event recognition in surveillance video has gained extensive attention from the computer vision community. This process still faces enormous challenges due to the tiny inter-class variations that are caused by various facets, such as severe occlusion, cluttered backgrounds, and so forth. To address these issues, we propose a spatio-temporal deep residual network with hierarchical attentions (STDRN-HA) for video event recognition. In the first attention layer, the ResNet fully connected feature guides the Faster R-CNN feature to generate object-based attention (O-attention) for target objects. In the second attention layer, the O-attention further guides the ResNet convolutional feature to yield the holistic attention (H-attention) in order to perceive more details of the occluded objects and the global background. In the third attention layer, the attention maps use the deep features to obtain the attention-enhanced features. Then, the attention-enhanced features are input into a deep residual recurrent network, which is used to mine more event clues from videos. Furthermore, an optimized loss function named softmax-RC is designed, which embeds the residual block regularization and center loss to solve the vanishing gradient in a deep network and enlarge the distance between inter-classes. We also build a temporal branch to exploit the long- and short-term motion information. The final results are obtained by fusing the outputs of the spatial and temporal streams. Experiments on the four realistic video datasets, CCV, VIRAT 1.0, VIRAT 2.0, and HMDB51, demonstrate that the proposed method has good performance and achieves state-of-the-art results.
更多查看译文
关键词
Event recognition,deep residual recurrent network,hierarchical attention,spatio-temporal,surveillance video
AI 理解论文
溯源树
样例
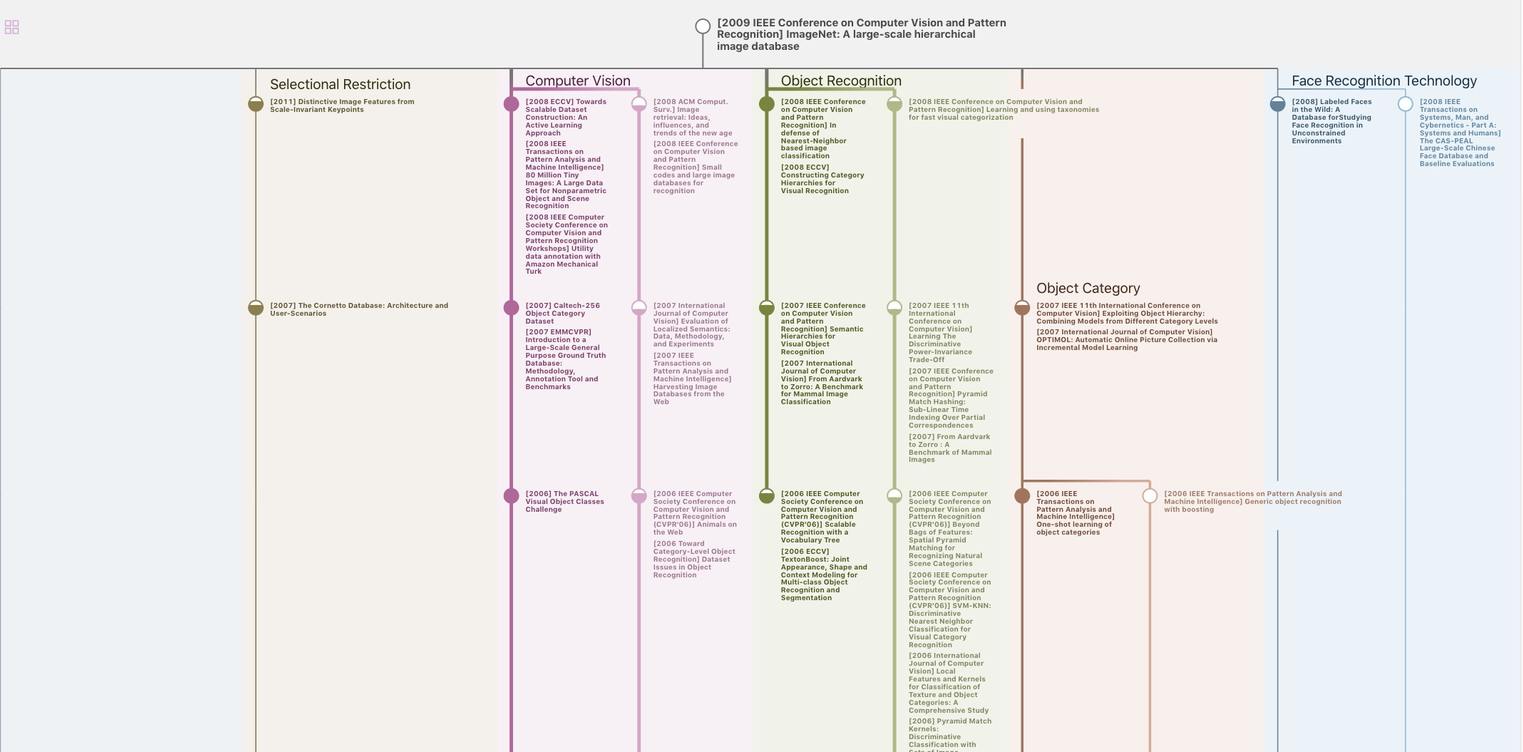
生成溯源树,研究论文发展脉络
Chat Paper
正在生成论文摘要