Autonomous lane change decision making using different deep reinforcement learning methods
CICTP 2019: Transportation in China - Connecting the World - Proceedings of the 19th COTA International Conference of Transportation Professionals,(2019)
摘要
Automated lane change decision making ability is significant for vehicles to adjust lanes to avoid collisions or to overtake other vehicles. However, traditional methods predicting all the possible situations using hand-crafted features is inefficient. In this paper, we propose a new lane change decision making agent based on deep reinforcement learning (DRL). In order to compare the performance differences of diverse DRL methods and state representations, our agents are trained via different DRL methods (DQN, A3C) and state representations. To demonstrate transferring ability, the agent trained in simple traffic condition is tested in more complicated conditions. Furthermore, a new way of combining DRL agents is implemented to improve agents' ability. These experiments show that our DRL agents are capable of lane change and have a superior transferring ability.
更多查看译文
AI 理解论文
溯源树
样例
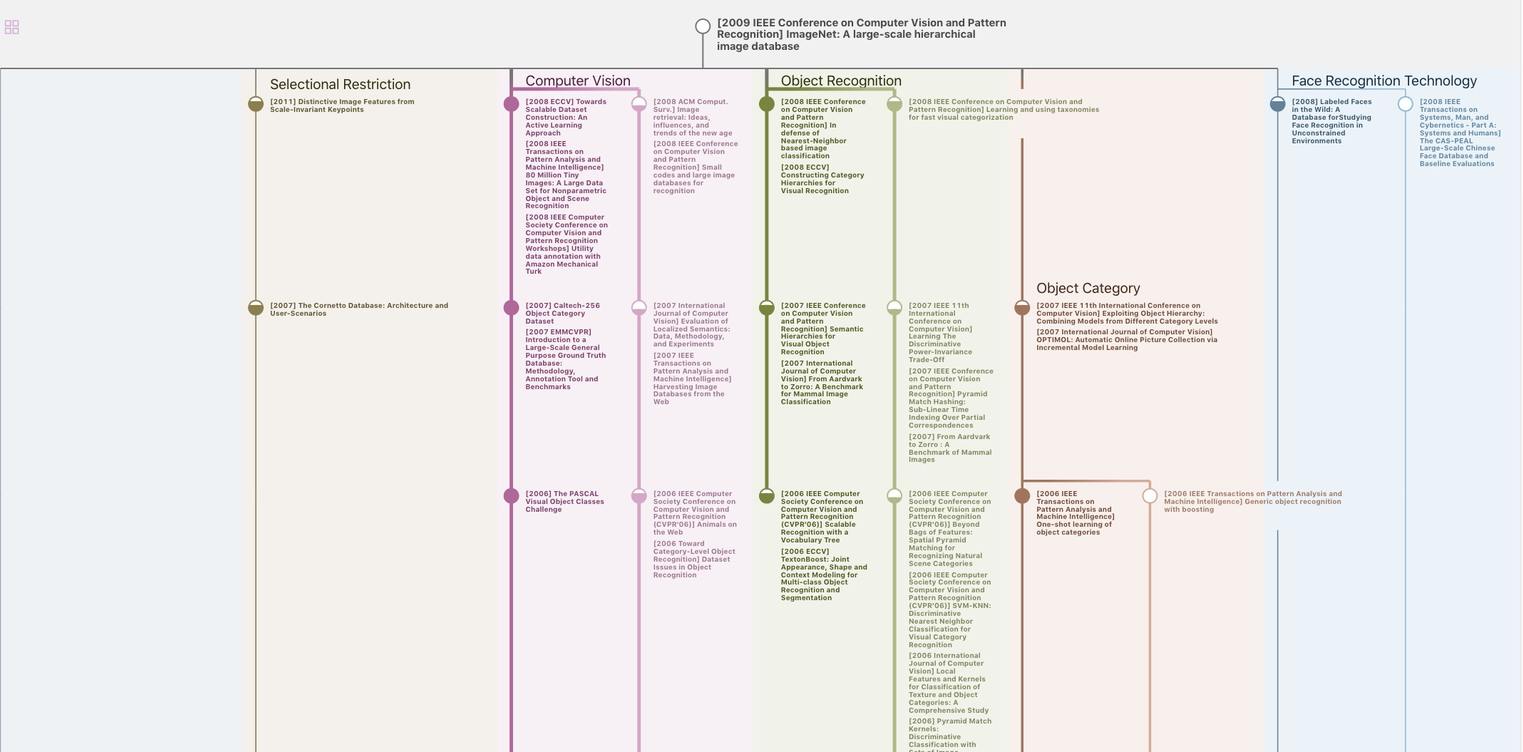
生成溯源树,研究论文发展脉络
Chat Paper
正在生成论文摘要