High-contrast "gaudy" images improve the training of deep neural network models of visual cortex
NIPS 2020(2020)
摘要
A key challenge in understanding the sensory transformations of the visual system is to obtain a highly predictive model of responses from visual cortical neurons. Deep neural networks (DNNs) provide a promising candidate for such a model. However, DNNs require orders of magnitude more training data than neuroscientists can collect from real neurons because experimental recording time is severely limited. This motivates us to find images that train highly-predictive DNNs with as little training data as possible. We propose gaudy images—high-contrast binarized versions of natural images—to efficiently train DNNs. In extensive simulation experiments, we find that training DNNs with gaudy images substantially reduces the number of training images needed to accurately predict the simulated responses of visual cortical neurons. We also find that gaudy images, chosen before training, outperform images chosen during training by active learning algorithms. Thus, gaudy images overemphasize features of natural images, especially edges, that are the most important for efficiently training DNNs. We believe gaudy images will aid in the modeling of visual cortical neurons, potentially opening new scientific questions about visual processing, as well as aid general practitioners that seek ways to improve the training of DNNs.
更多查看译文
关键词
images,deep neural network
AI 理解论文
溯源树
样例
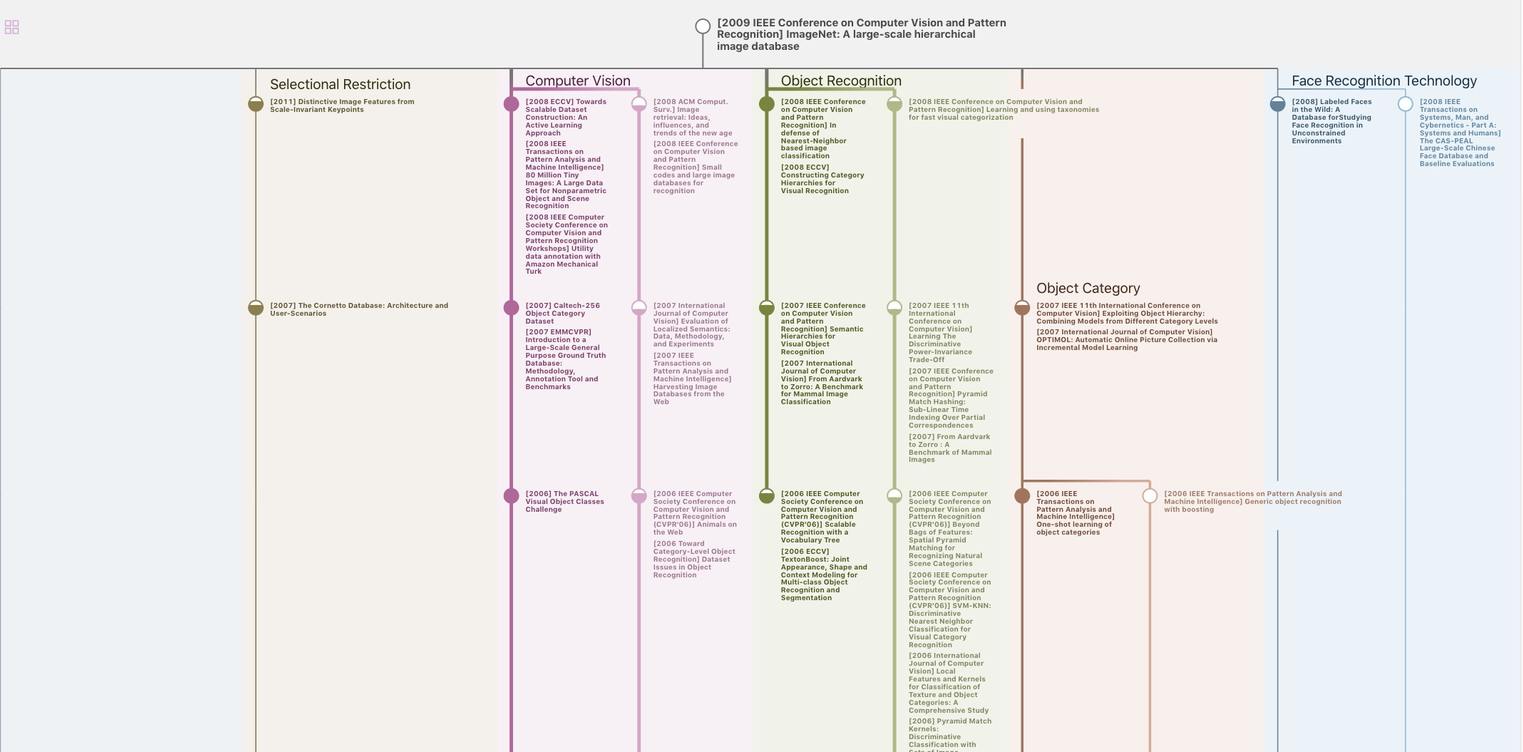
生成溯源树,研究论文发展脉络
Chat Paper
正在生成论文摘要