Defense against Adversarial Attacks in NLP via Dirichlet Neighborhood Ensemble
arxiv(2020)
摘要
Despite neural networks have achieved prominent performance on many natural language processing (NLP) tasks, they are vulnerable to adversarial examples. In this paper, we propose Dirichlet Neighborhood Ensemble (DNE), a randomized smoothing method for training a robust model to defense substitution-based attacks. During training, DNE forms virtual sentences by sampling embedding vectors for each word in an input sentence from a convex hull spanned by the word and its synonyms, and it augments them with the training data. In such a way, the model is robust to adversarial attacks while maintaining the performance on the original clean data. DNE is agnostic to the network architectures and scales to large models for NLP applications. We demonstrate through extensive experimentation that our method consistently outperforms recently proposed defense methods by a significant margin across different network architectures and multiple data sets.
更多查看译文
关键词
adversarial attacks,dirichlet neighborhood ensemble,nlp
AI 理解论文
溯源树
样例
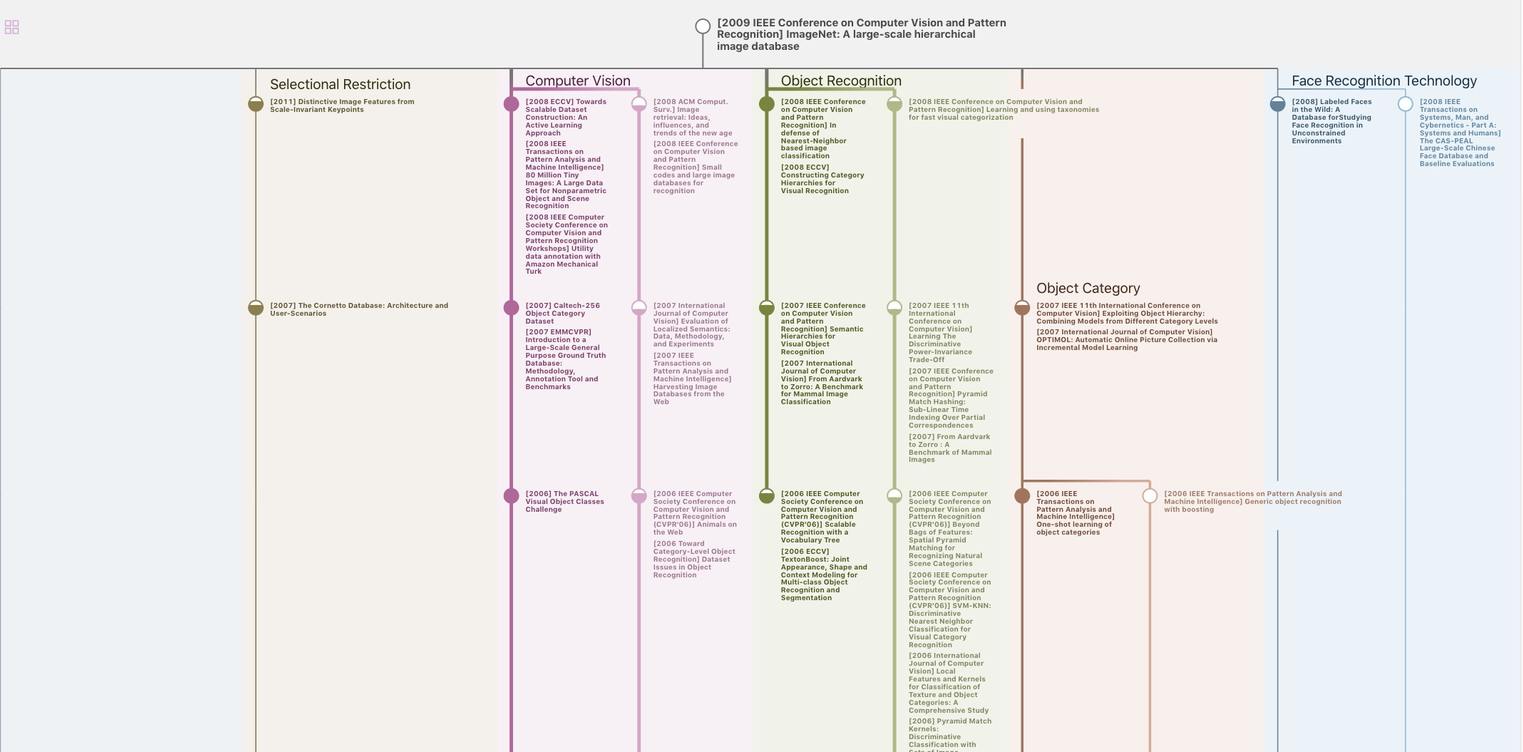
生成溯源树,研究论文发展脉络
Chat Paper
正在生成论文摘要