On the Divergence of Decentralized Non-Convex Optimization
arxiv(2020)
摘要
We study a generic class of decentralized algorithms in which N agents jointly optimize the non-convex objective f(u):=1/N∑_i=1^Nf_i(u), while only communicating with their neighbors. This class of problems has become popular in modeling many signal processing and machine learning applications, and many efficient algorithms have been proposed. However, by constructing some counter-examples, we show that when certain local Lipschitz conditions (LLC) on the local function gradient ∇ f_i's are not satisfied, most of the existing decentralized algorithms diverge, even if the global Lipschitz condition (GLC) is satisfied, where the sum function f has Lipschitz gradient. This observation raises an important open question: How to design decentralized algorithms when the LLC, or even the GLC, is not satisfied? To address the above question, we design a first-order algorithm called Multi-stage gradient tracking algorithm (MAGENTA), which is capable of computing stationary solutions with neither the LLC nor the GLC. In particular, we show that the proposed algorithm converges sublinearly to certain ϵ-stationary solution, where the precise rate depends on various algorithmic and problem parameters. In particular, if the local function f_i's are Qth order polynomials, then the rate becomes 𝒪(1/ϵ^Q-1). Such a rate is tight for the special case of Q=2 where each f_i satisfies LLC. To our knowledge, this is the first attempt that studies decentralized non-convex optimization problems with neither the LLC nor the GLC.
更多查看译文
关键词
optimization,divergence
AI 理解论文
溯源树
样例
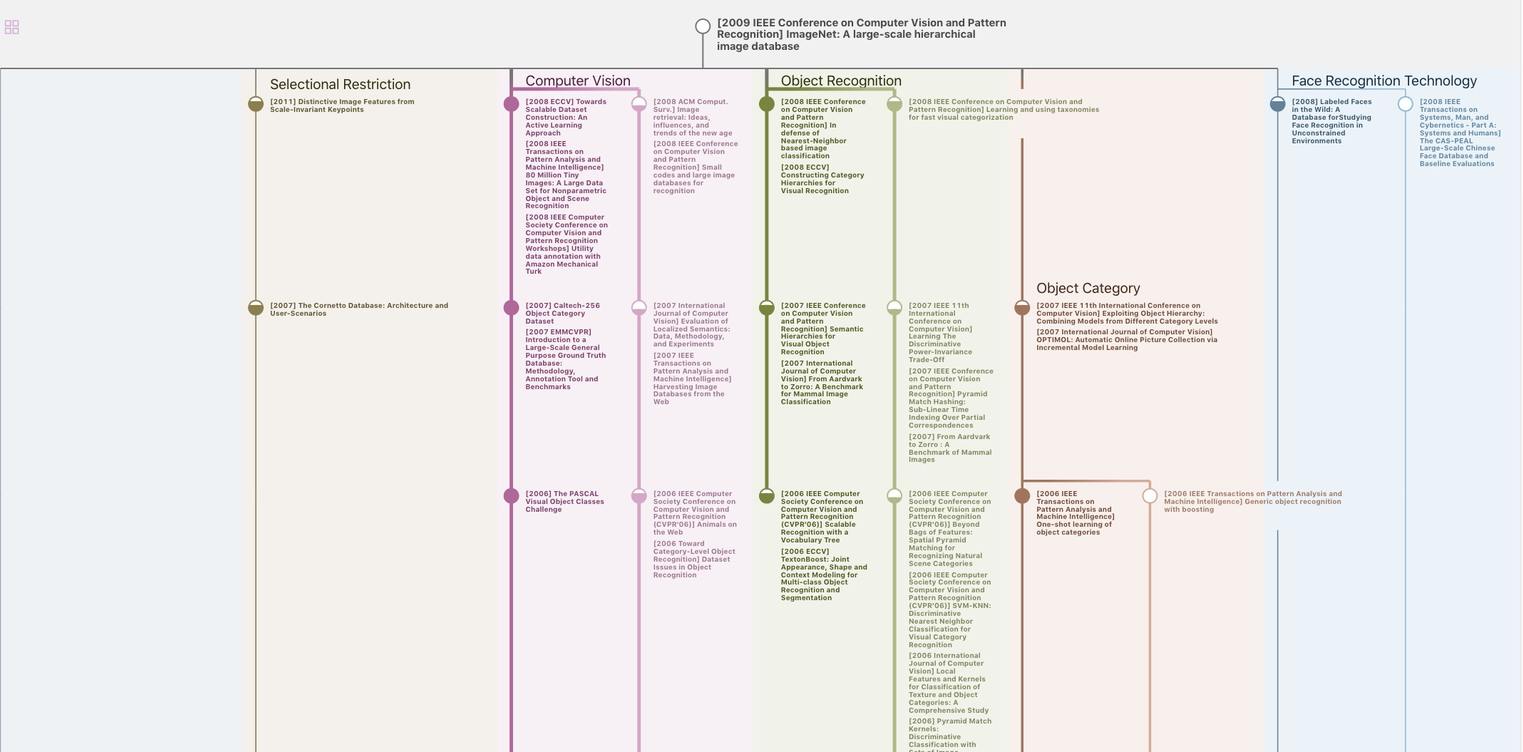
生成溯源树,研究论文发展脉络
Chat Paper
正在生成论文摘要