AD-NET: Age-adjust neural network for improved MCI to AD conversion prediction.
NeuroImage. Clinical(2020)
摘要
The prediction of Mild Cognitive Impairment (MCI) patients who are at higher risk converting to Alzheimer's Disease (AD) is critical for effective intervention and patient selection in clinical trials. Different biomarkers including neuroimaging have been developed to serve the purpose. With extensive methodology development efforts on neuroimaging, an emerging field is deep learning research. One great challenge facing deep learning is the limited medical imaging data available. To address the issue, researchers explore the use of transfer learning to extend the applicability of deep models on neuroimaging research for AD diagnosis and prognosis. Existing transfer learning models mostly focus on transferring the features from the pre-training into the fine-tuning stage. Recognizing the advantages of the knowledge gained during the pre-training, we propose an AD-NET (Age-adjust neural network) with the pre-training model serving two purposes: extracting and transferring features; and obtaining and transferring knowledge. Specifically, the knowledge being transferred in this research is an age-related surrogate biomarker. To evaluate the effectiveness of the proposed approach, AD-NET is compared with 8 classification models from literature using the same public neuroimaging dataset. Experimental results show that the proposed AD-NET outperforms the competing models in predicting the MCI patients at risk for conversion to the AD stage.
更多查看译文
关键词
ad-net conversion prediction,improved mci,age-adjust
AI 理解论文
溯源树
样例
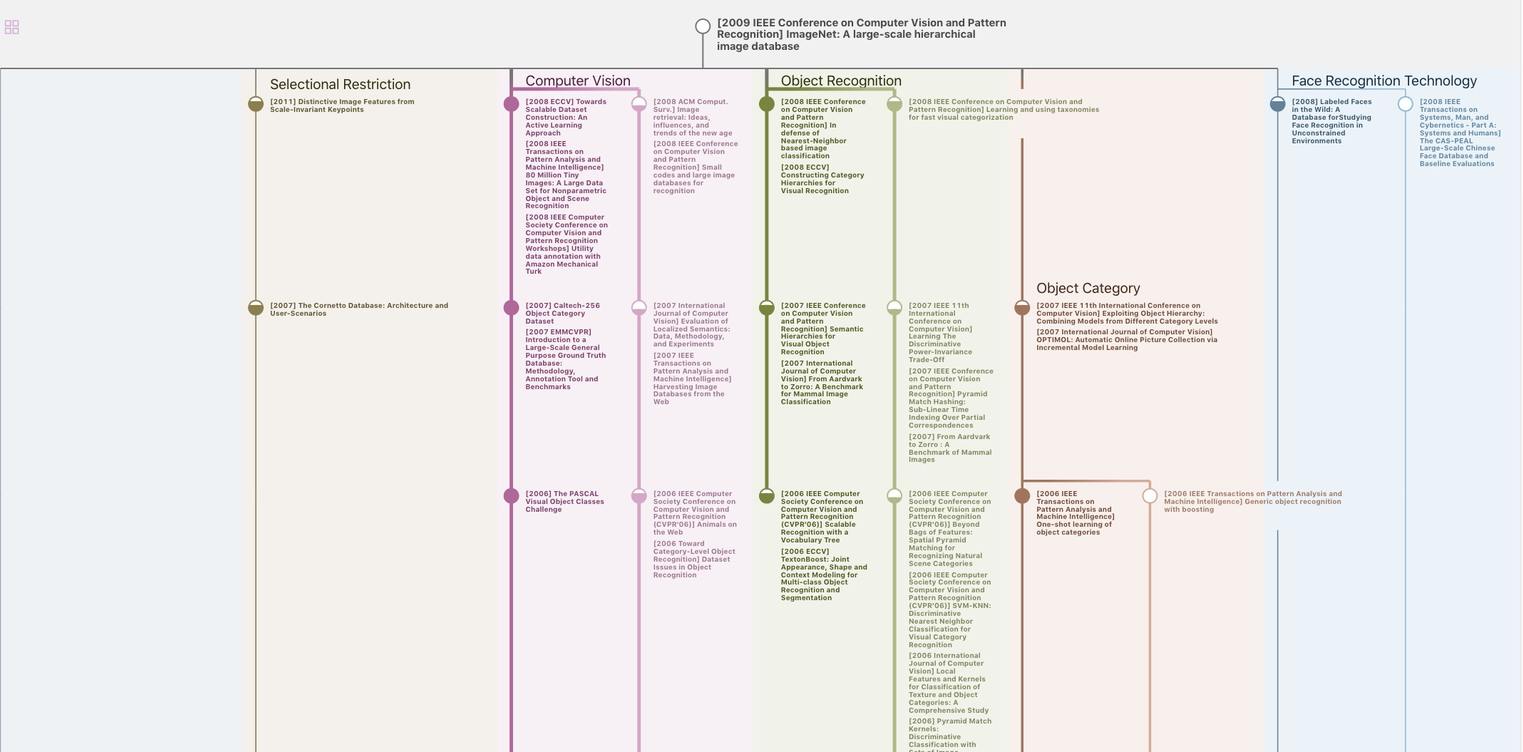
生成溯源树,研究论文发展脉络
Chat Paper
正在生成论文摘要