Learning Interclass Relations for Image Classification
arxiv(2020)
摘要
In standard classification, we typically treat class categories as independent of one-another. In many problems, however, we would be neglecting the natural relations that exist between categories, which are often dictated by an underlying biological or physical process. In this work, we propose novel formulations of the classification problem, based on a realization that the assumption of class-independence is a limiting factor that leads to the requirement of more training data. First, we propose manual ways to reduce our data needs by reintroducing knowledge about problem-specific interclass relations into the training process. Second, we propose a general approach to jointly learn categorical label representations that can implicitly encode natural interclass relations, alleviating the need for strong prior assumptions, which are not always available. We demonstrate this in the domain of medical images, where access to large amounts of labelled data is not trivial. Specifically, our experiments show the advantages of this approach in the classification of Intravenous Contrast enhancement phases in CT images, which encapsulate multiple interesting inter-class relations.
更多查看译文
关键词
interclass relations,classification,learning,image
AI 理解论文
溯源树
样例
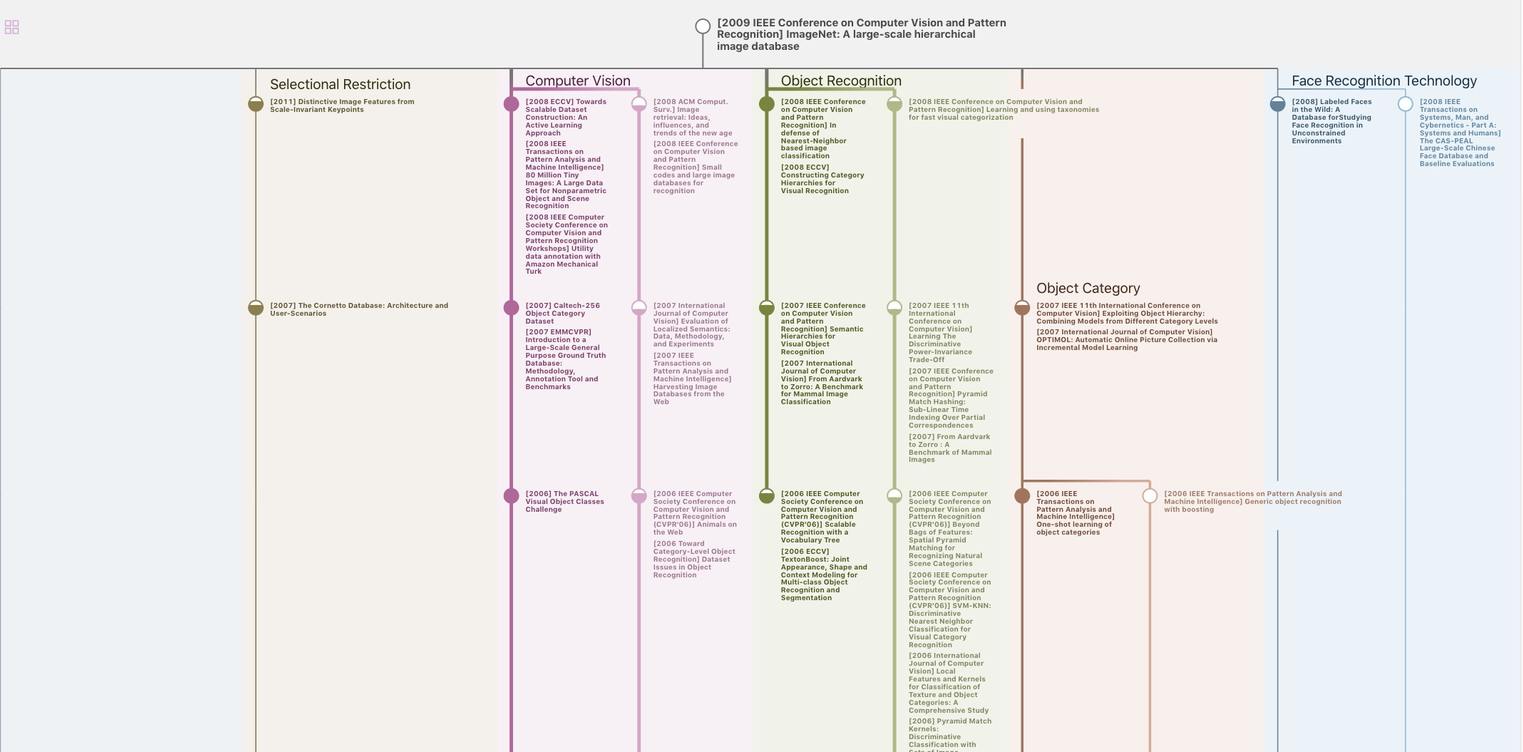
生成溯源树,研究论文发展脉络
Chat Paper
正在生成论文摘要