Competitive Balance in Team Sports Games
2020 IEEE Conference on Games (CoG)(2020)
摘要
Competition is a primary driver of player satisfaction and engagement in multiplayer online games. Traditional matchmaking systems aim at creating matches involving teams of similar aggregated individual skill levels, such as Elo score or TrueSkill. However, team dynamics cannot be solely captured using such linear predictors. Recently, it has been shown that nonlinear predictors that target to learn probability of winning as a function of player and team features significantly outperforms these linear skill-based methods. In this paper, we show that using final score difference provides yet a better prediction metric for competitive balance. We also show that a linear model trained on a carefully selected set of team and individual features achieves almost the performance of the more powerful neural network model while offering two orders of magnitude inference speed improvement. This shows significant promise for implementation in online matchmaking systems.
更多查看译文
关键词
matchmaking,game balancing,massively online multiplayer game,competitive balance,machine learning
AI 理解论文
溯源树
样例
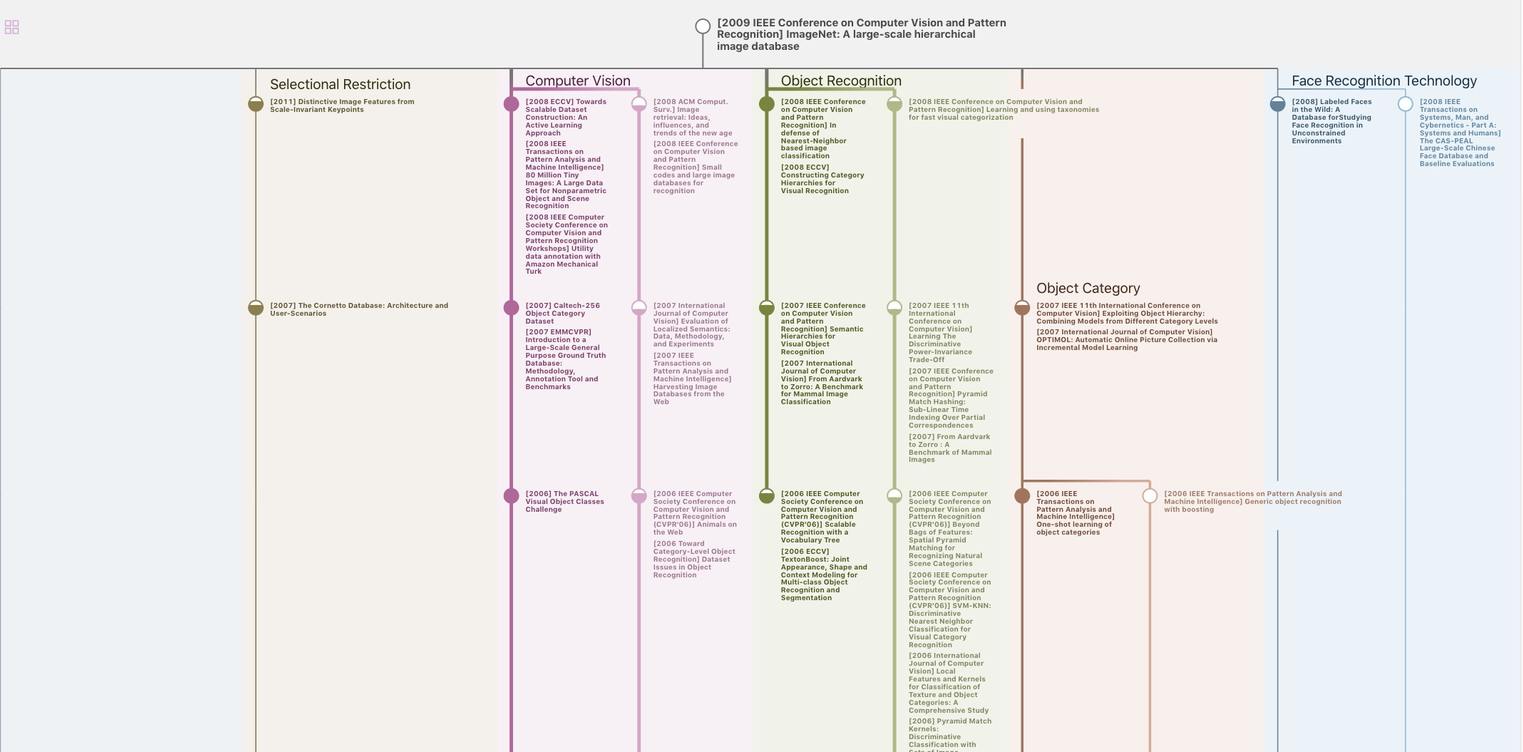
生成溯源树,研究论文发展脉络
Chat Paper
正在生成论文摘要