HARMONIES: A Hybrid Approach for Microbiome Networks Inference Via Exploiting Sparsity
Frontiers in genetics(2020)
摘要
The human microbiome is a collection of microorganisms. They form complex communities and collectively affect host health. Recently, the advances in next-generation sequencing technology enable the high-throughput profiling of the human microbiome. This calls for a statistical model to construct microbial networks from the microbiome sequencing count data. As microbiome count data are high-dimensional and suffer from uneven sampling depth, over-dispersion, and zero-inflation, these characteristics can bias the network estimation and require specialized analytical tools. Here we propose a general framework, HARMONIES, Hybrid Approach foR MicrobiOme Network Inferences via Exploiting Sparsity, to infer a sparse microbiome network. HARMONIES first utilizes a zero-inflated negative binomial (ZINB) distribution to model the skewness and excess zeros in the microbiome data, as well as incorporates a stochastic process prior for sample-wise normalization. This approach infers a sparse and stable network by imposing non-trivial regularizations based on the Gaussian graphical model. In comprehensive simulation studies, HARMONIES outperformed four other commonly used methods. When using published microbiome data from a colorectal cancer study, it discovered a novel community with disease-enriched bacteria. In summary, HARMONIES is a novel and useful statistical framework for microbiome network inference, and it is available at.
更多查看译文
关键词
Bayesian statistics,microbiome network,Gaussian graphical model,Dirichlet process prior,hierarchical model
AI 理解论文
溯源树
样例
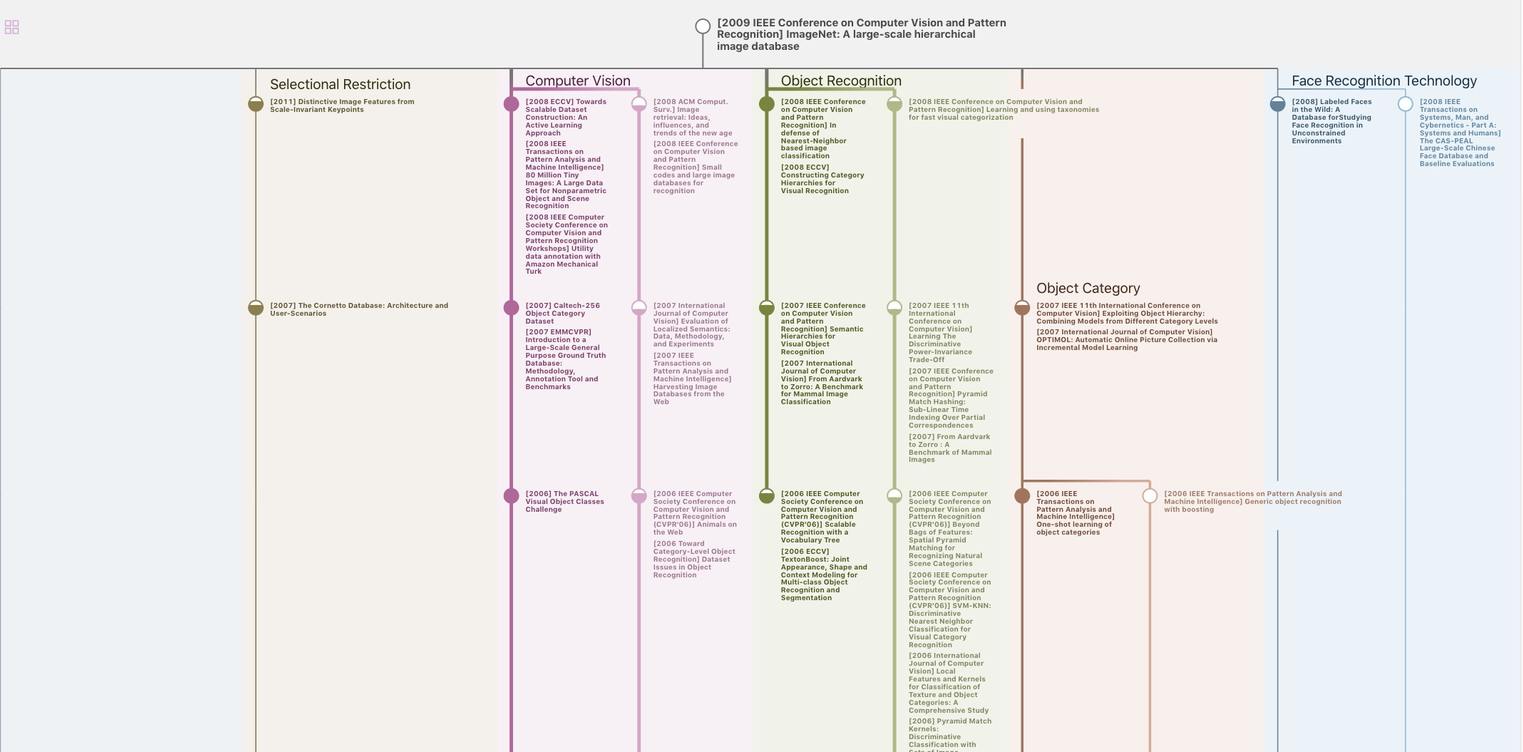
生成溯源树,研究论文发展脉络
Chat Paper
正在生成论文摘要