Intense bitterness of molecules: Machine learning for expediting drug discovery
Computational and Structural Biotechnology Journal(2021)
摘要
Drug development is a long, expensive and multistage process geared to achieving safe drugs with high efficacy. A crucial prerequisite for completing the medication regimen for oral drugs, particularly for pediatric and geriatric populations, is achieving taste that does not hinder compliance. Currently, the aversive taste of drugs is tested in late stages of clinical trials. This can result in the need to reformulate, potentially resulting in the use of more animals for additional toxicity trials, increased financial costs and a delay in release to the market. Here we present BitterIntense, a machine learning tool that classifies molecules into "very bitter" or "not very bitter", based on their chemical structure. The model, trained on chemically diverse compounds, has above 80% accuracy on several test sets. Our results suggest that about 25% of drugs are predicted to be very bitter, with even higher prevalence (similar to 40%) in COVID19 drug candidates and in microbial natural products. Only similar to 10% of toxic molecules are predicted to be intensely bitter, and it is also suggested that intense bitterness does not correlate with hepatotoxicity of drugs. However, very bitter compounds may be more cardiotoxic than not very bitter compounds, possessing significantly lower QPlogHERG values. BitterIntense allows quick and easy prediction of strong bitterness of compounds of interest for food, pharma and biotechnology industries. We estimate that implementation of BitterIntense or similar tools early in drug discovery process may lead to reduction in delays, in animal use and in overall financial burden. (C) 2021 The Authors. Published by Elsevier B.V. on behalf of Research Network of Computational and Structural Biotechnology.
更多查看译文
关键词
Machine learning,Bitter,Taste,Drugs,Drug discovery,Toxicity
AI 理解论文
溯源树
样例
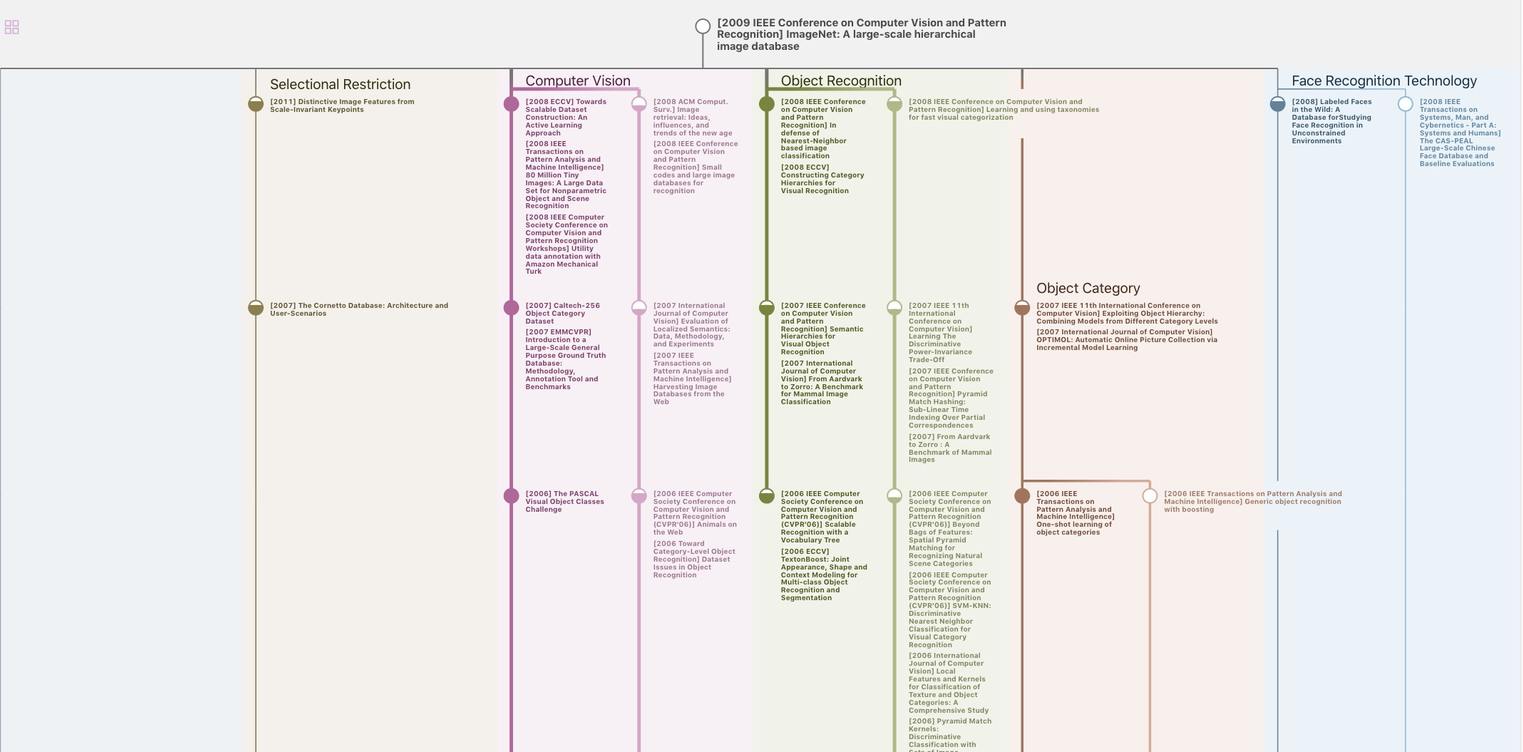
生成溯源树,研究论文发展脉络
Chat Paper
正在生成论文摘要