Diagnostic accuracy of deep learning in orthopaedic fractures: a systematic review and meta-analysis.
CLINICAL RADIOLOGY(2020)
摘要
AIM: To gather and compare related clinical studies, and to investigate the accuracy and reliability of deep learning in detecting orthopaedic fractures. MATERIALS AND METHODS: This study is a retrospective combination and interpretation of prospectively acquired data. Articles from PubMed, EMBASE, the Cochrane library databases, and reference lists of the qualified articles were retrieved. Heterogeneity between studies was assessed using a random effective model. Pooled sensitivity, specificity, diagnostic odds ratio, and area under the receiver operating characteristic curve (AUC) were obtained by a random model. This work was managed from October 2018 to March 2020. RESULTS: Fourteen studies were included in this systematic review and nine were synthesized in the meta-analysis. The pooled sensitivity and specificity for the whole group (17 trials, 5,434 images) were 0.87 and 0.91, respectively. The AUC was 0.95. Eight trials (1,574 images) were included in the long-bone group, which contained seven studies. The pooled sensitivity was 0.96 and specificity was 0.94. The AUC was 0.99. Heterogeneity existed in the four pooled results of the whole group and the pooled specificity of the long-bone group. CONCLUSIONS: Deep learning is reliable in fracture diagnosis and has high diagnostic accuracy, which is similar to that of general physicians and is unlikely to produce a large number of false diagnoses; however, the ability of deep learning to localize the fracture needs more attention and testing. Deep learning can be extremely helpful with pre-classification of clinical diagnoses. (C) 2020 The Royal College of Radiologists. Published by Elsevier Ltd. All rights reserved.
更多查看译文
AI 理解论文
溯源树
样例
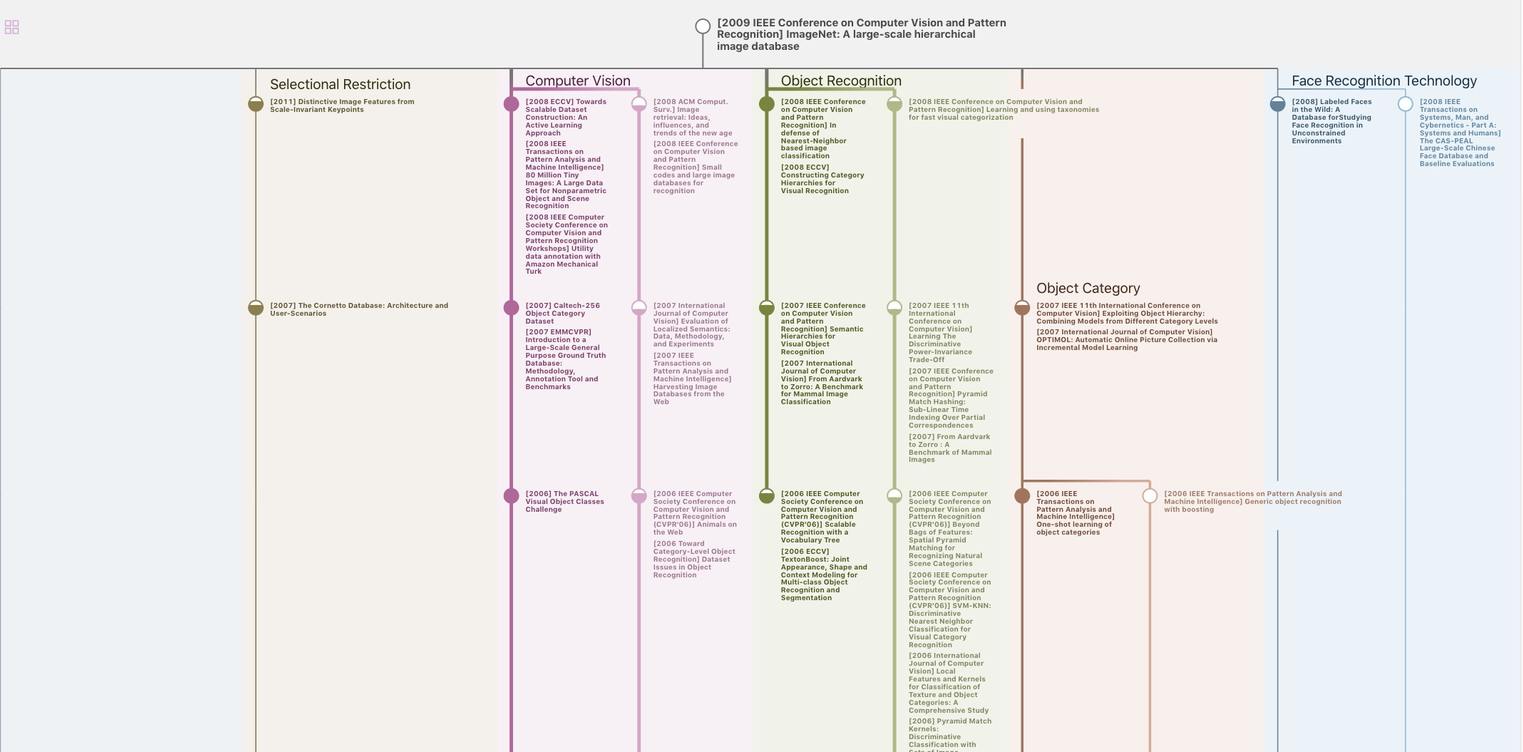
生成溯源树,研究论文发展脉络
Chat Paper
正在生成论文摘要