On The Completeness Of Causal Discovery In The Presence Of Latent Confounding With Tiered Background Knowledge
INTERNATIONAL CONFERENCE ON ARTIFICIAL INTELLIGENCE AND STATISTICS, VOL 108(2020)
摘要
The discovery of causal relationships is a core part of scientific research. Accordingly, over the past several decades, algorithms have been developed to discover the causal structure for a system of variables from observational data. Learning ancestral graphs is of particular interest due to their ability to represent latent confounding implicitly with bi-directed edges. The well-known FCI algorithm provably recovers an ancestral graph for a system of variables encoding the sound and complete set of causal relationships identifiable from observational data.(1) Additional causal relationships become identifiable with the incorporation of background knowledge; however, it is not known for what types of knowledge FCI remains complete. In this paper, we define tiered background knowledge and show that FCI is sound and complete with the incorporation of this knowledge.
更多查看译文
AI 理解论文
溯源树
样例
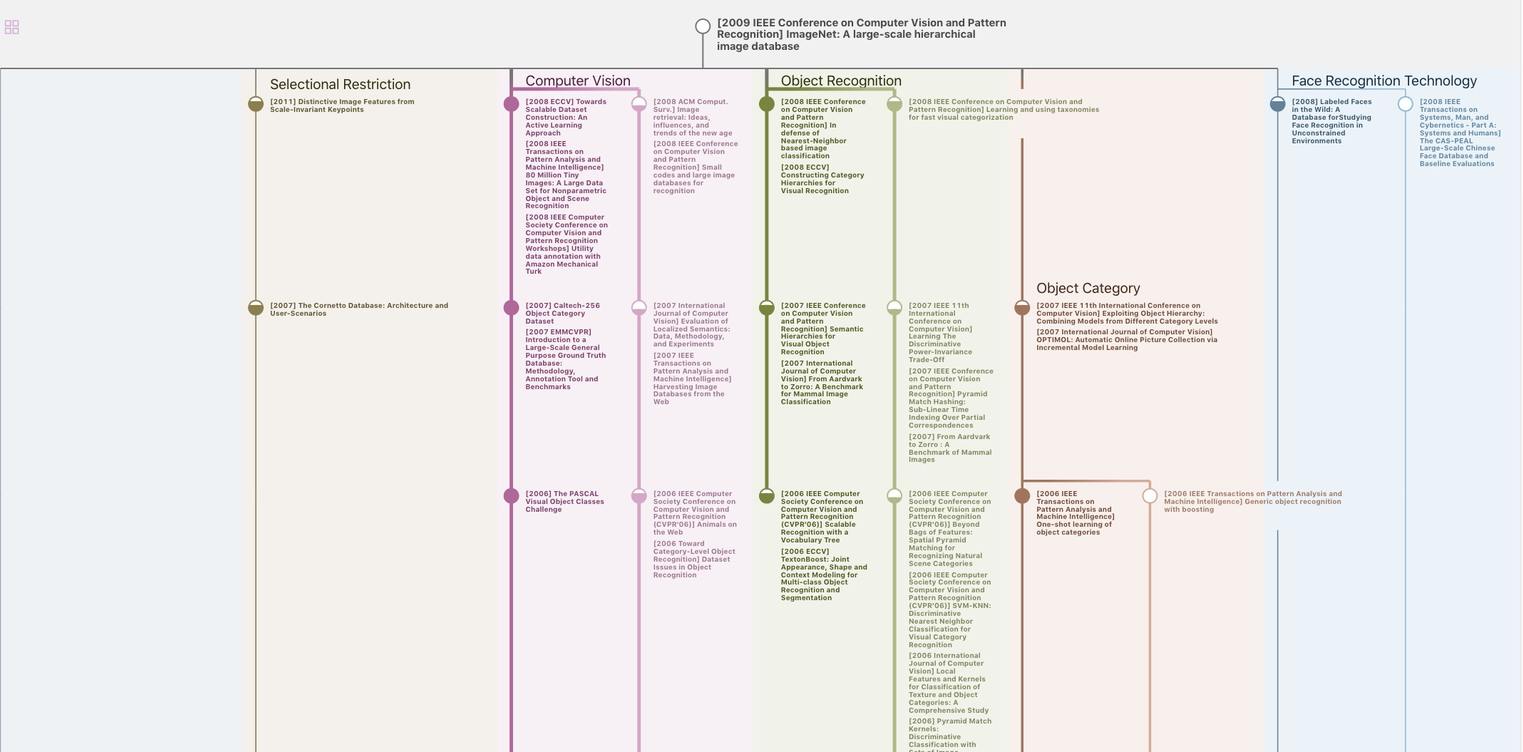
生成溯源树,研究论文发展脉络
Chat Paper
正在生成论文摘要