HAF-SVG: Hierarchical Stochastic Video Generation with Aligned Features
IJCAI 2020(2020)
摘要
Stochastic video generation methods predict diverse videos based on observed frames, where the main challenge lies in modeling the complex future uncertainty and generating realistic frames. Numerous of Recurrent-VAE-based methods have achieved state-of-the-art results. However, on the one hand, the independence assumption of the variables of approximate posterior limits the inference performance [Zhao et al., 2017; Blei et al., 2017]. On the other hand, although these methods adopt skip connections between encoder and decoder to utilize multi-level features, they still produce blurry generation due to the spatial misalignment between encoder and decoder features at different time steps. In this paper, we propose a hierarchical recurrent VAE with a feature aligner, which can not only relax the independence assumption in typical VAE but also use a feature aligner to enable the decoder to obtain the aligned spatial information from the last observed frames. The proposed model is named Hierarchical Stochastic Video Generation network with Aligned Features, referred to as HAF-SVG. Experiments on Moving-MNIST, BAIR, and KTH datasets demonstrate that hierarchical structure is helpful for modeling more accurate future uncertainty, and the feature aligner is beneficial to generate realistic frames. Besides, the HAF-SVG exceeds SVG on both prediction accuracy and the quality of generated frames.
更多查看译文
AI 理解论文
溯源树
样例
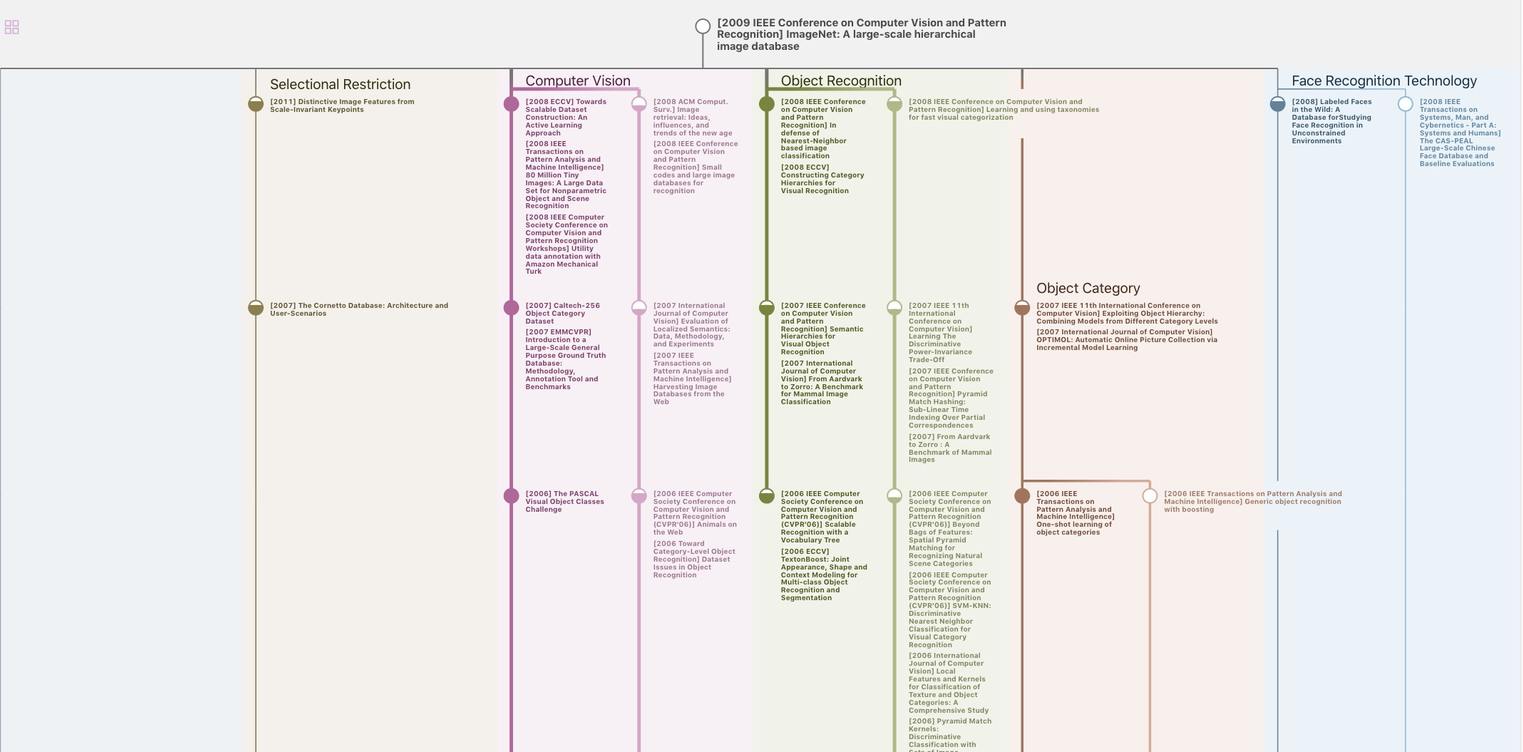
生成溯源树,研究论文发展脉络
Chat Paper
正在生成论文摘要