Domain Adaptive Classification on Heterogeneous Information Networks
IJCAI 2020(2020)
摘要
Heterogeneous Information Networks (HINs) are ubiquitous structures in that they can depict complex relational data. Due to their complexity, it is hard to obtain sufficient labeled data on HINs, hampering classification on HINs. While domain adaptation (DA) techniques have been widely utilized in images and texts, the heterogeneity and complex semantics pose specific challenges towards domain adaptive classification on HINs. On one hand, HINs involve multiple levels of semantics, making it demanding to do domain alignment among them. On the other hand, the trade-off between domain similarity and distinguishability must be elaborately chosen, in that domain invariant features have been shown to be homogeneous and uninformative for classification. In this paper, we propose Multi-space Domain Adaptive Classification (MuSDAC) to handle the problem of DA on HINs. Specifically, we utilize multi-channel shared weight GCNs, projecting nodes in HINs to multiple spaces where pairwise alignment is carried out. In addition, we propose a heuristic sampling algorithm that efficiently chooses the combination of channels featuring distinguishability, and moving-averaged weighted voting scheme to fuse the selected channels, minimizing both transfer and classification loss. Extensive experiments on pairwise datasets endorse not only our model's performance on domain adaptive classification on HINs and contributions by individual components.
更多查看译文
AI 理解论文
溯源树
样例
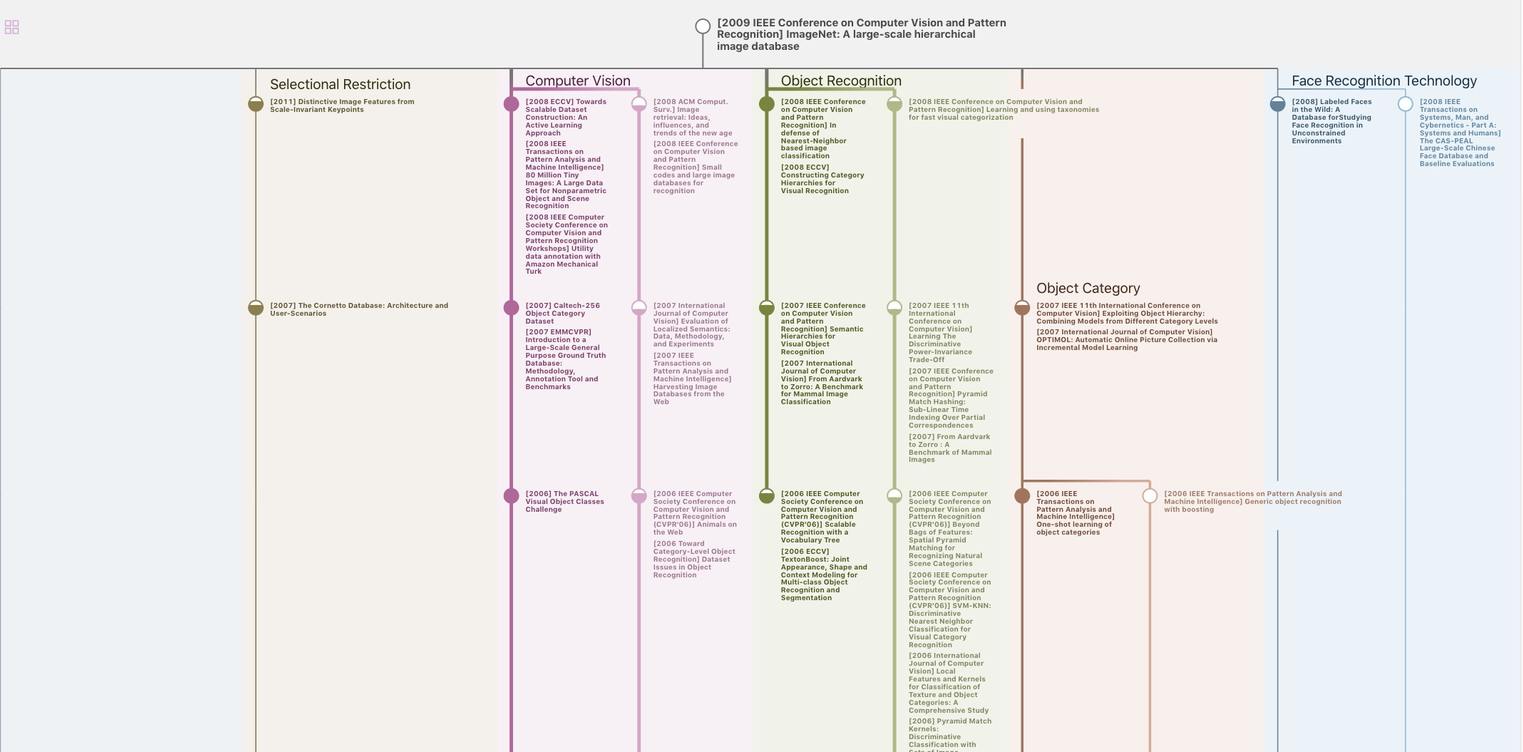
生成溯源树,研究论文发展脉络
Chat Paper
正在生成论文摘要