Learning Model with Error -- Exposing the Hidden Model of BAYHENN
IJCAI 2020(2020)
摘要
Privacy-preserving deep neural network (DNN) inference remains an intriguing problem even after the rapid developments of different communities. One challenge is that cryptographic techniques such as homomorphic encryption (HE) do not natively support non-linear computations (e.g., sigmoid). A recent work, BAYHENN (Xie et al., IJCAI'19), considers HE over the Bayesian neural network (BNN). The novelty lies in "meta-prediction" over a few noisy DNNs. The claim was that the clients can get intermediate outputs (to apply non-linear function) but are still prevented from learning the exact model parameters, which was justified via the widely-used learning-with-error (LWE) assumption (with Gaussian noises as the error). This paper refutes the security claim of BAYHENN via both theoretical and empirical analyses. We formally define a security game with different oracle queries capturing two realistic threat models. Our attack assuming a semi-honest adversary reveals all the parameters of single-layer BAYHENN, which generalizes to recovering the whole model that is "as good as" the BNN approximation of the original DNN, either under the malicious adversary model or with an increased number of oracle queries. This shows the need for rigorous security analysis ("the noise introduced by BNN can obfuscate the model" fails -- it is beyond what LWE guarantees) and calls for the collaboration between cryptographers and machine-learning experts to devise practical yet provably-secure solutions.
更多查看译文
关键词
Multidisciplinary Topics and Applications: Security and Privacy,Machine Learning: Deep Learning
AI 理解论文
溯源树
样例
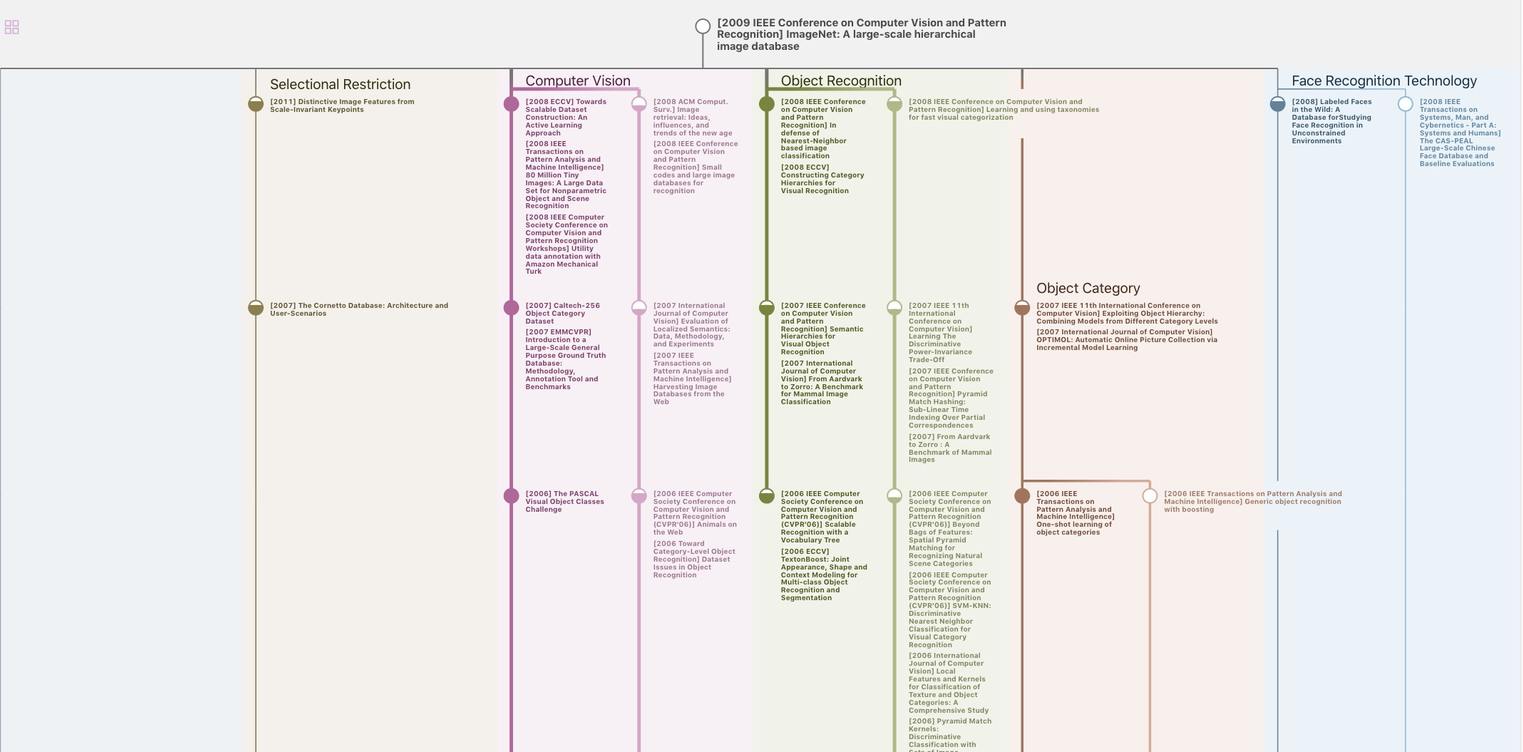
生成溯源树,研究论文发展脉络
Chat Paper
正在生成论文摘要