On The Convergence Of The Metropolis Algorithm With Fixed-Order Updates For Multivariate Binary Probability Distributions
24TH INTERNATIONAL CONFERENCE ON ARTIFICIAL INTELLIGENCE AND STATISTICS (AISTATS)(2021)
摘要
The Metropolis algorithm is arguably the most fundamental Markov chain Monte Carlo (MCMC) method. But the algorithm is not guaranteed to converge to the desired distribution in the case of multivariate binary distributions (e.g., Ising models or stochastic neural networks such as Boltzmann machines) if the variables (sites or neurons) are updated in a fixed order, a setting commonly used in practice. The reason is that the corresponding Markov chain may not be irreducible. We propose a modified Metropolis transition operator that behaves almost always identically to the standard Metropolis operator and prove that it ensures irreducibility and convergence to the limiting distribution in the multivariate binary case with fixed-order updates. The result provides an explanation for the behaviour of Metropolis MCMC in that setting and closes a long-standing theoretical gap. We experimentally studied the standard and modified Metropolis operator for models where they actually behave differently. If the standard algorithm also converges, the modified operator exhibits similar (if not better) performance in terms of convergence speed.
更多查看译文
关键词
metropolis algorithm,multivariate binary probability distributions,fixed-order
AI 理解论文
溯源树
样例
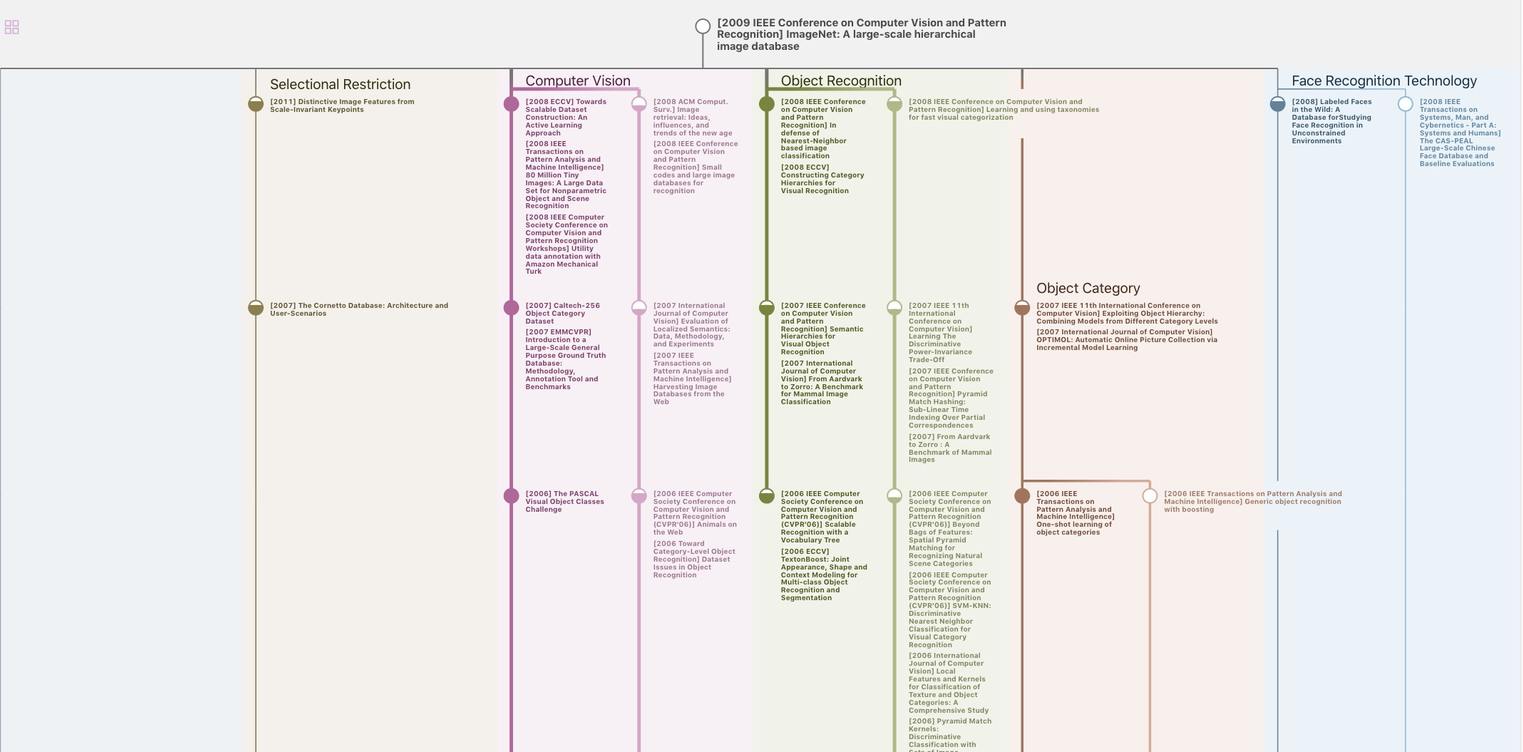
生成溯源树,研究论文发展脉络
Chat Paper
正在生成论文摘要