Effective Exploration For Mavs Based On The Expected Information Gain
DRONES(2018)
摘要
Micro aerial vehicles (MAVs) are an excellent platform for autonomous exploration. Most MAVs rely mainly on cameras for buliding a map of the 3D environment. Therefore, vision-based MAVs require an efficient exploration algorithm to select viewpoints that provide informative measurements. In this paper, we propose an exploration approach that selects in real time the next-best-view that maximizes the expected information gain of new measurements. In addition, we take into account the cost of reaching a new viewpoint in terms of distance and predictability of the flight path for a human observer. Finally, our approach selects a path that reduces the risk of crashes when the expected battery life comes to an end, while still maximizing the information gain in the process. We implemented and thoroughly tested our approach and the experiments show that it offers an improved performance compared to other state-of-the-art algorithms in terms of precision of the reconstruction, execution time, and smoothness of the path.
更多查看译文
关键词
exploration, information gain, vision
AI 理解论文
溯源树
样例
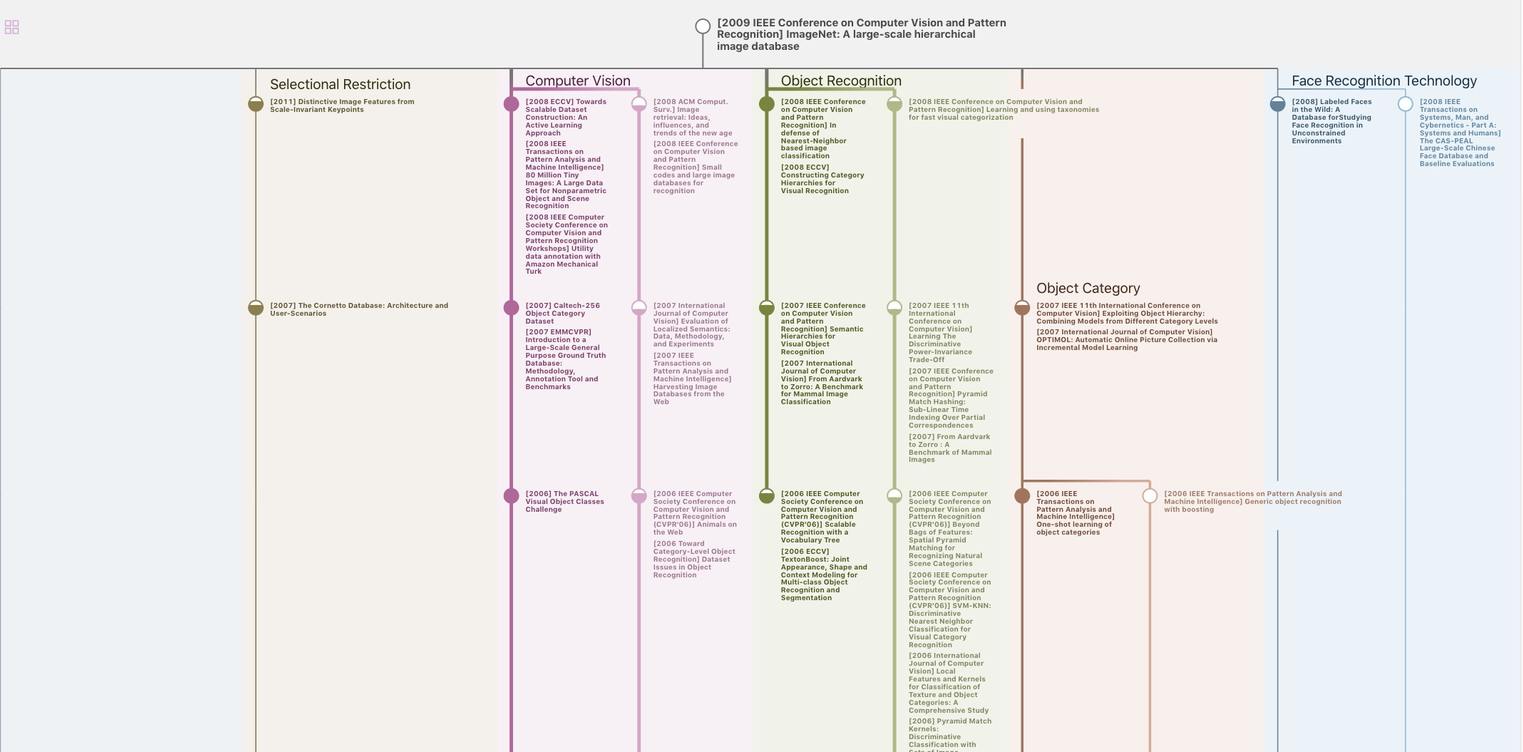
生成溯源树,研究论文发展脉络
Chat Paper
正在生成论文摘要