Understanding Gradient Clipping in Private SGD: A Geometric Perspective
NIPS 2020(2020)
摘要
Deep learning models are increasingly popular in many machine learning applications where the training data may contain sensitive information. To provide formal and rigorous privacy guarantee, many learning systems now incorporate differential privacy by training their models with (differentially) private SGD. A key step in each private SGD update is gradient clipping that shrinks the gradient of an individual example whenever its L2 norm exceeds some threshold. We first demonstrate how gradient clipping can prevent SGD from converging to stationary point. We then provide a theoretical analysis that fully quantifies the clipping bias on convergence with a disparity measure between the gradient distribution and a geometrically symmetric distribution. Our empirical evaluation further suggests that the gradient distributions along the trajectory of private SGD indeed exhibit symmetric structure that favors convergence. Together, our results provide an explanation why private SGD with gradient clipping remains effective in practice despite its potential clipping bias. Finally, we develop a new perturbation-based technique that can provably correct the clipping bias even for instances with highly asymmetric gradient distributions.
更多查看译文
关键词
private sgd,gradient clipping
AI 理解论文
溯源树
样例
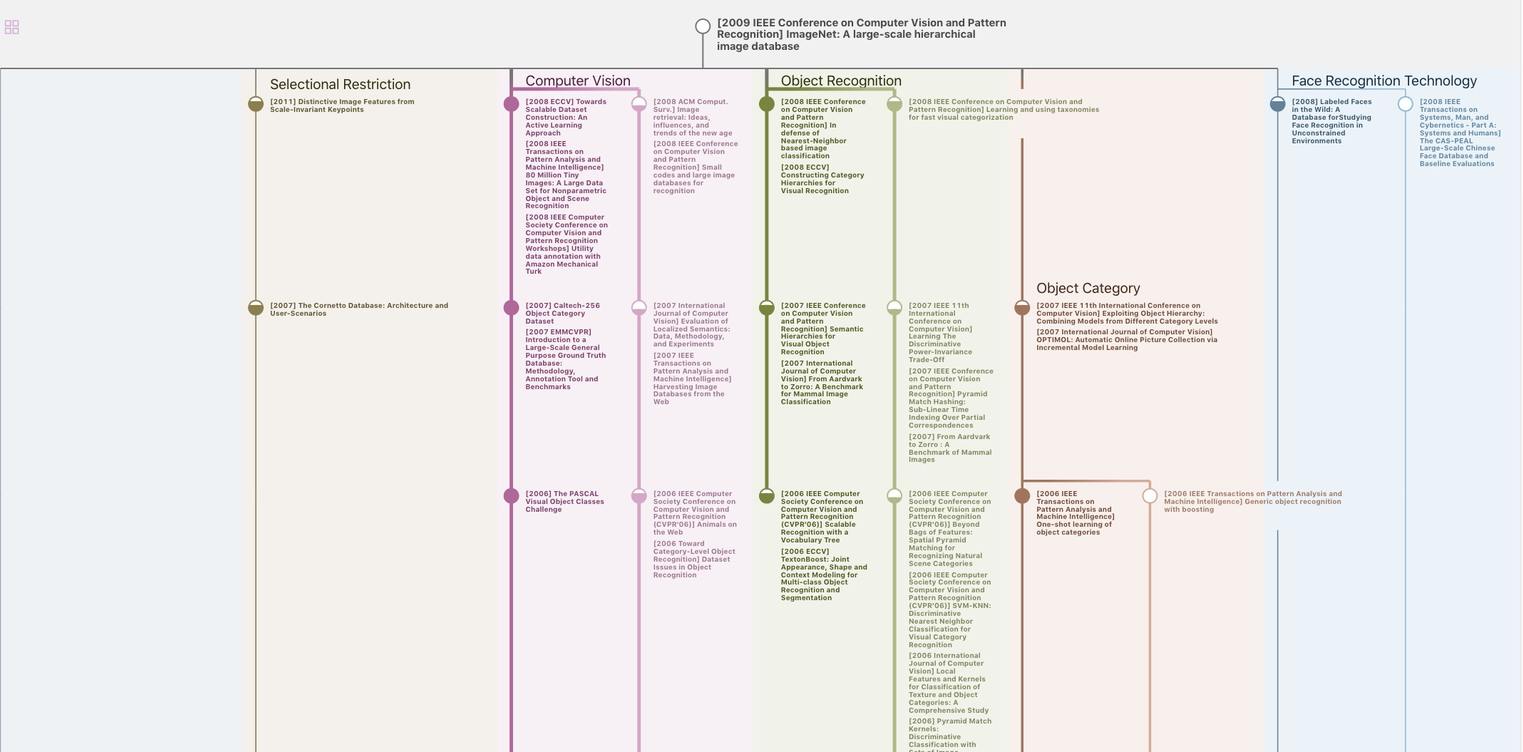
生成溯源树,研究论文发展脉络
Chat Paper
正在生成论文摘要