Improving VQA and its Explanations by Comparing Competing Explanations
arxiv(2020)
摘要
Most recent state-of-the-art Visual Question Answering (VQA) systems are opaque black boxes that are only trained to fit the answer distribution given the question and visual content. As a result, these systems frequently take shortcuts, focusing on simple visual concepts or question priors. This phenomenon becomes more problematic as the questions become complex that requires more reasoning and commonsense knowledge. To address this issue, we present a novel framework that uses explanations for competing answers to help VQA systems select the correct answer. By training on human textual explanations, our framework builds better representations for the questions and visual content, and then reweights confidences in the answer candidates using either generated or retrieved explanations from the training set. We evaluate our framework on the VQA-X dataset, which has more difficult questions with human explanations, achieving new state-of-the-art results on both VQA and its explanations.
更多查看译文
关键词
competing explanations,vqa
AI 理解论文
溯源树
样例
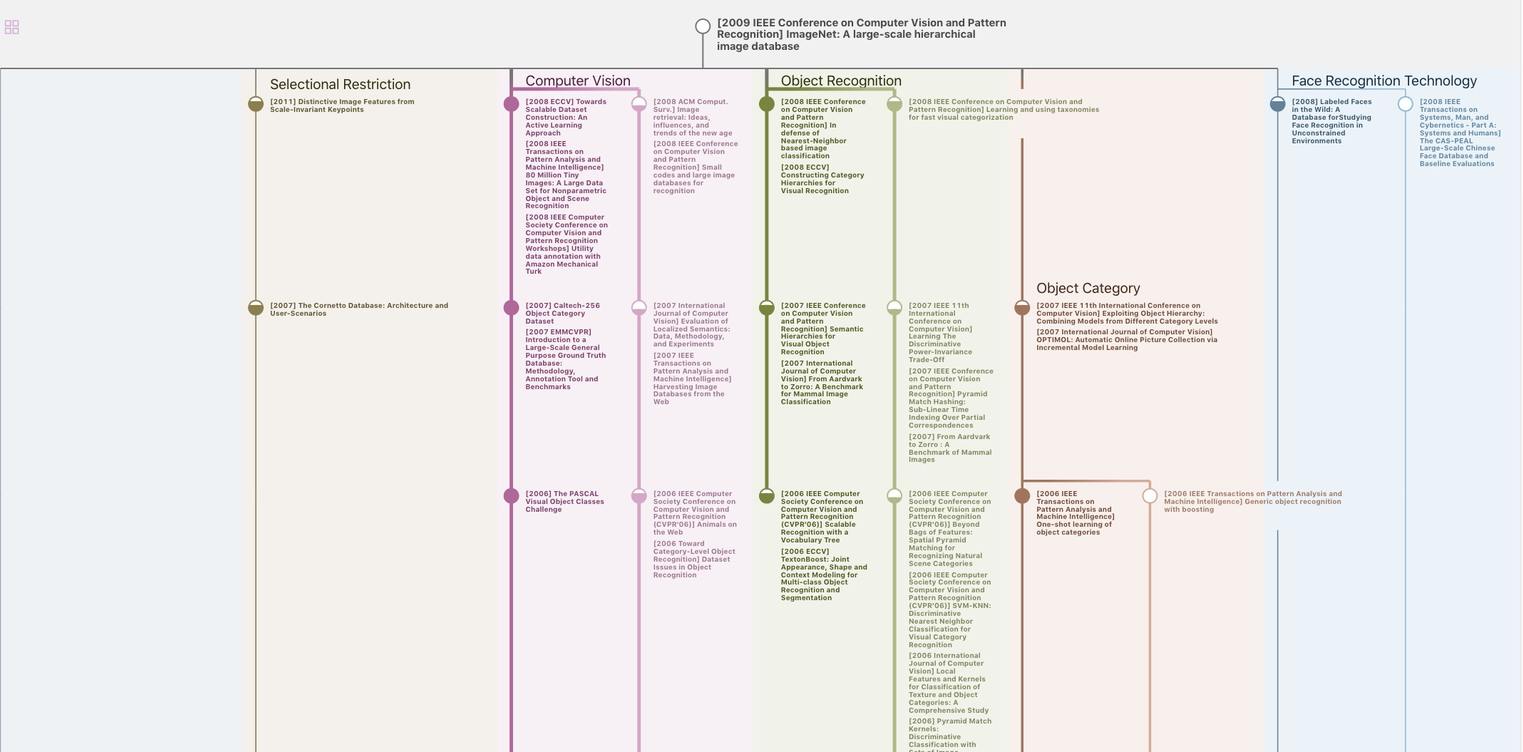
生成溯源树,研究论文发展脉络
Chat Paper
正在生成论文摘要