First-Line Toxicological Screening With Fully Automated Extraction
JOURNAL OF ANALYTICAL TOXICOLOGY(2021)
摘要
In clinical toxicology, laboratories need screening methods allowing unambiguous identification of the compounds in a short turnaround time to either confirm or exclude the hypothesis of drug overdose or poisoning with a toxicant. We developed a fully automated screening procedure designed to identify and quantify in a single run 245 compounds of interest in clinical toxicology. Sample extraction was carried out by a programmable liquid handler directly coupled to a liquid chromatography-tandem mass spectrometry (LC-MS-MS) system. Data acquisition was performed in the positive and negative ionization modes with up to 15 multiple reaction monitoring (MRM) transitions per compound, each with optimized collision energy to enable both qualitative library searching and quantitation. The method was validated according to the ISO 15189 requirements and was applied to real patient samples (n = 127). The 15 MRM transitions per compound provided higher confidence for the identification of all the compounds. The quantitative method was fully validated with satisfactory intra- and inter-assay imprecision and inaccuracy with CV% lower than 20%. For only nine molecules, imprecision and inaccuracy were relatively high but never exceeded 31.7%. Comparison with dedicated quantitative methods using conventional MRM monitoring performed using 127 patient samples (n= 175 pairs of measured concentrations) showed excellent correlation (R-2 = 0.96). A robustness study showed that calibration curves prepared for up to 1 month yielded uncertainty <20%. Retention times ranged from 0.89 min for metformin to 9.72 min for difenacoum. The automated sample preparation required 8 min and was followed by 10 min chromatographic separation. This first-line screening procedure yields high confidence in compound detection and should be useful in core labs facing clinical toxicology situations where rapid and reliable results are needed.
更多查看译文
AI 理解论文
溯源树
样例
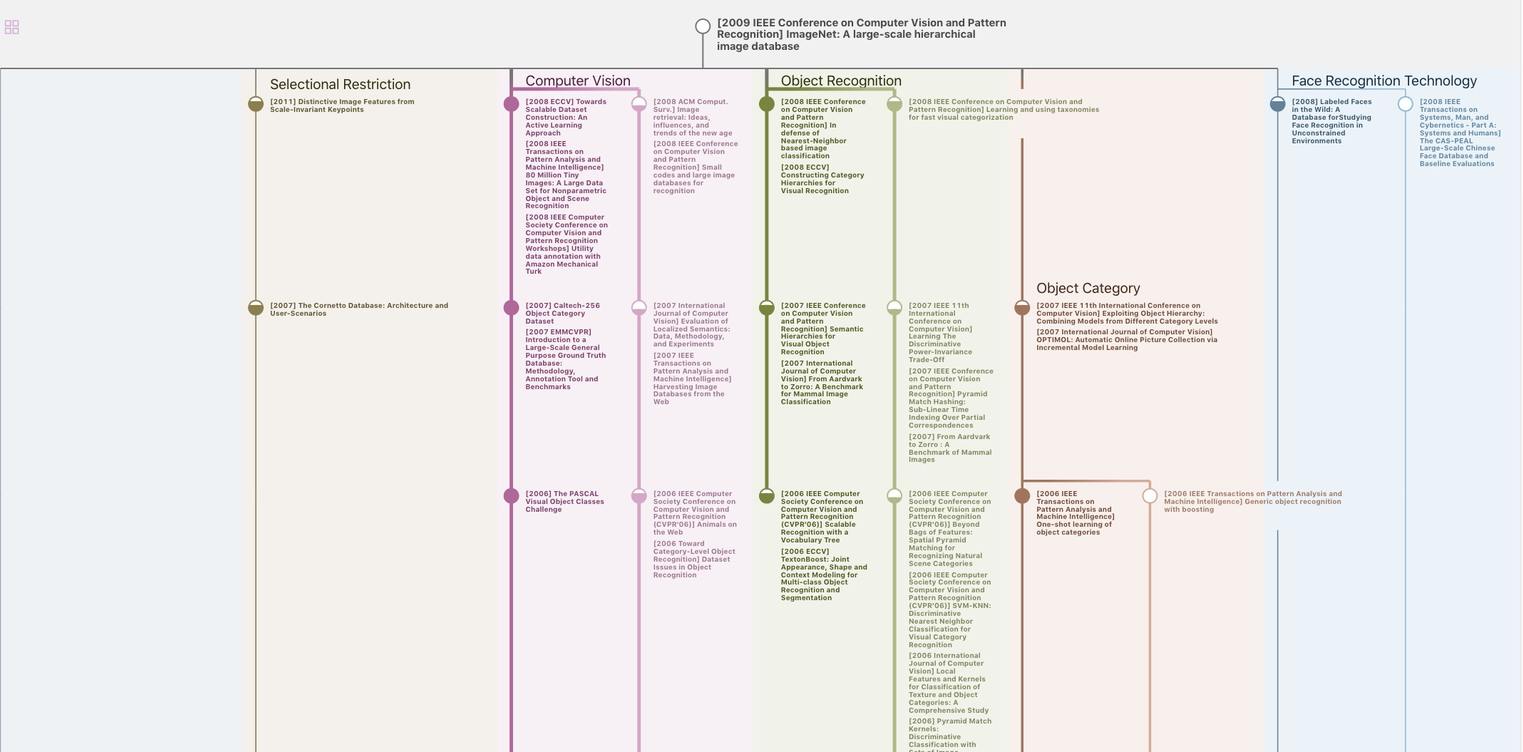
生成溯源树,研究论文发展脉络
Chat Paper
正在生成论文摘要