Approximation rates for neural networks with encodable weights in smoothness spaces
Neural Networks(2021)
摘要
We examine the necessary and sufficient complexity of neural networks to approximate functions from different smoothness spaces under the restriction of encodable network weights. Based on an entropy argument, we start by proving lower bounds for the number of nonzero encodable weights for neural network approximation in Besov spaces, Sobolev spaces and more. These results are valid for all sufficiently smooth activation functions. Afterwards, we provide a unifying framework for the construction of approximate partitions of unity by neural networks with fairly general activation functions. This allows us to approximate localized Taylor polynomials by neural networks and make use of the Bramble–Hilbert Lemma. Based on our framework, we derive almost optimal upper bounds in higher-order Sobolev norms. This work advances the theory of approximating solutions of partial differential equations by neural networks.
更多查看译文
关键词
Neural networks,Expressivity,Approximation rates,Smoothness spaces,Encodable weights
AI 理解论文
溯源树
样例
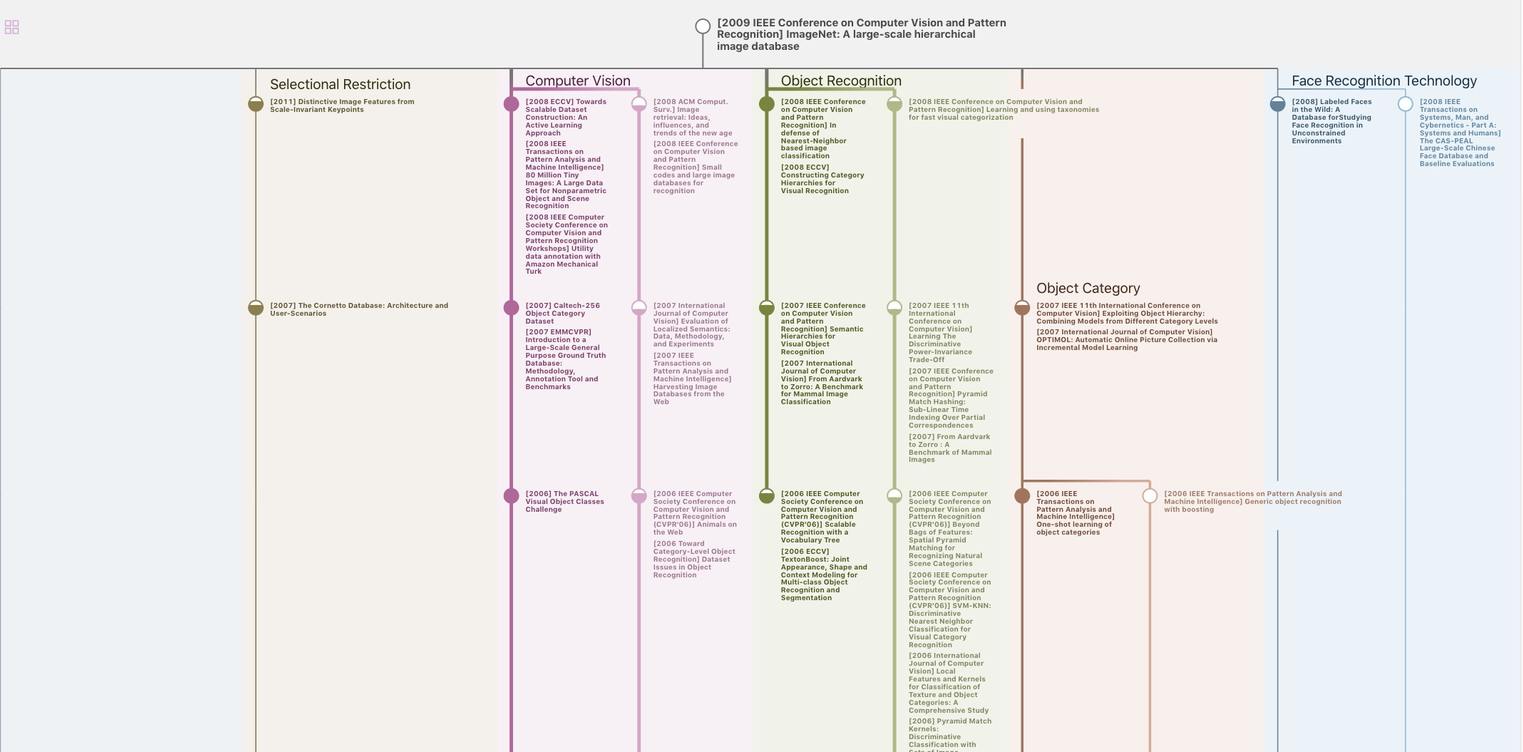
生成溯源树,研究论文发展脉络
Chat Paper
正在生成论文摘要