Continuous-Time Multi-Armed Bandits with Controlled Restarts
arxiv(2020)
摘要
Time-constrained decision processes have been ubiquitous in many fundamental applications in physics, biology and computer science. Recently, restart strategies have gained significant attention for boosting the efficiency of time-constrained processes by expediting the completion times. In this work, we investigate the bandit problem with controlled restarts for time-constrained decision processes, and develop provably good learning algorithms. In particular, we consider a bandit setting where each decision takes a random completion time, and yields a random and correlated reward at the end, with unknown values at the time of decision. The goal of the decision-maker is to maximize the expected total reward subject to a time constraint τ. As an additional control, we allow the decision-maker to interrupt an ongoing task and forgo its reward for a potentially more rewarding alternative. For this problem, we develop efficient online learning algorithms with O(log(τ)) and O(√(τlog(τ))) regret in a finite and continuous action space of restart strategies, respectively. We demonstrate an applicability of our algorithm by using it to boost the performance of SAT solvers.
更多查看译文
关键词
controlled,continuous-time,multi-armed
AI 理解论文
溯源树
样例
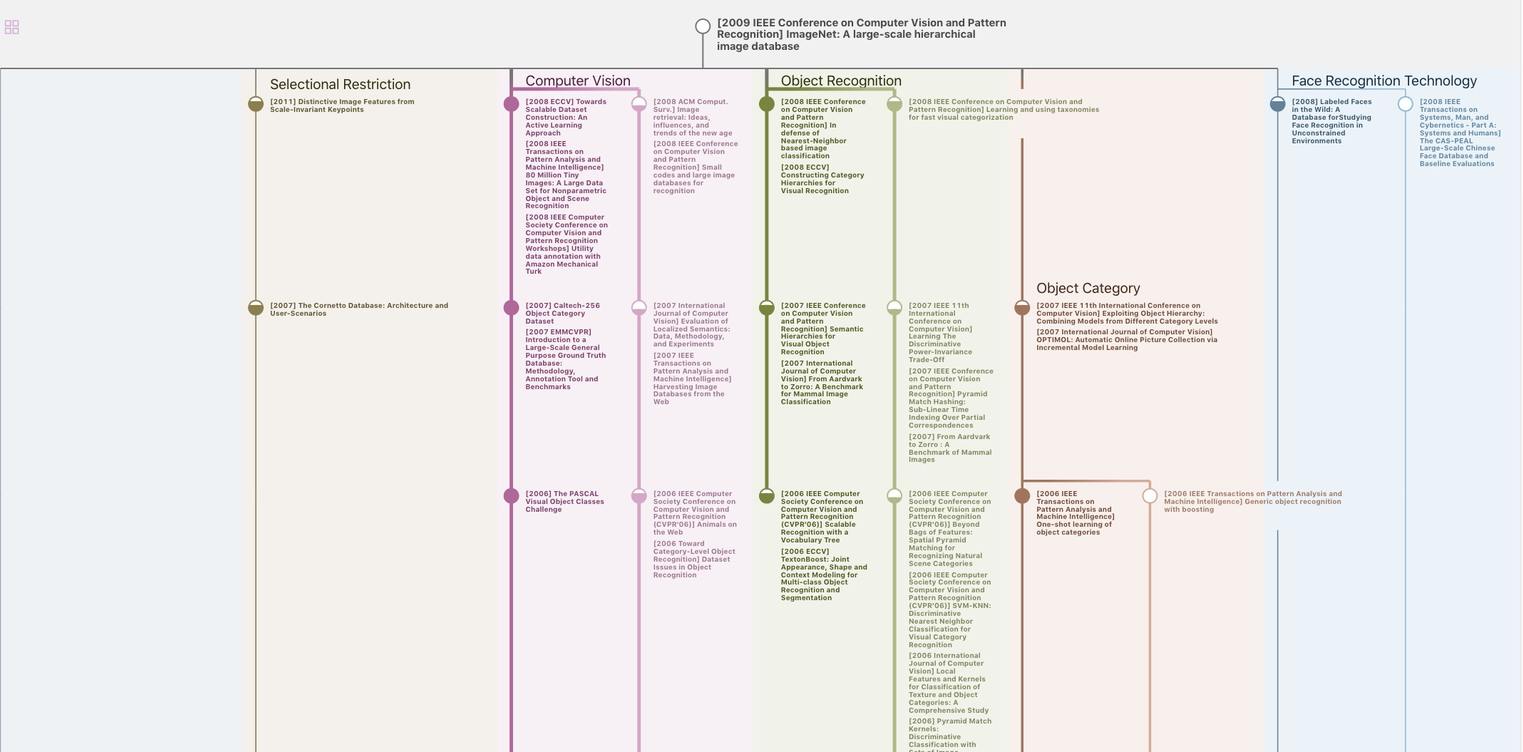
生成溯源树,研究论文发展脉络
Chat Paper
正在生成论文摘要