Accelerating Bayesian Structure Learning in Sparse Gaussian Graphical Models
JOURNAL OF THE AMERICAN STATISTICAL ASSOCIATION(2021)
摘要
Bayesian structure learning in Gaussian graphical models is often done by search algorithms over the graph space.The conjugate prior for the precision matrix satisfying graphical constraints is the well-known G-Wishart.With this prior, the transition probabilities in the search algorithms necessitate evaluating the ratios of the prior normalizing constants of G-Wishart.In moderate to high-dimensions, this ratio is often approximated by using sampling-based methods as computationally expensive updates in the search algorithm.Calculating this ratio so far has been a major computational bottleneck.We overcome this issue by representing a search algorithm in which the ratio of normalizing constants is carried out by an explicit closed-form approximation.Using this approximation within our search algorithm yields significant improvement in the scalability of structure learning without sacrificing structure learning accuracy.We study the conditions under which the approximation is valid.We also evaluate the efficacy of our method with simulation studies.We show that the new search algorithm with our approximation outperforms state-of-the-art methods in both computational efficiency and accuracy.The implementation of our work is available in the R package BDgraph.
更多查看译文
关键词
Bayes factors,Model selection,Normalizing constants,G-Wishart
AI 理解论文
溯源树
样例
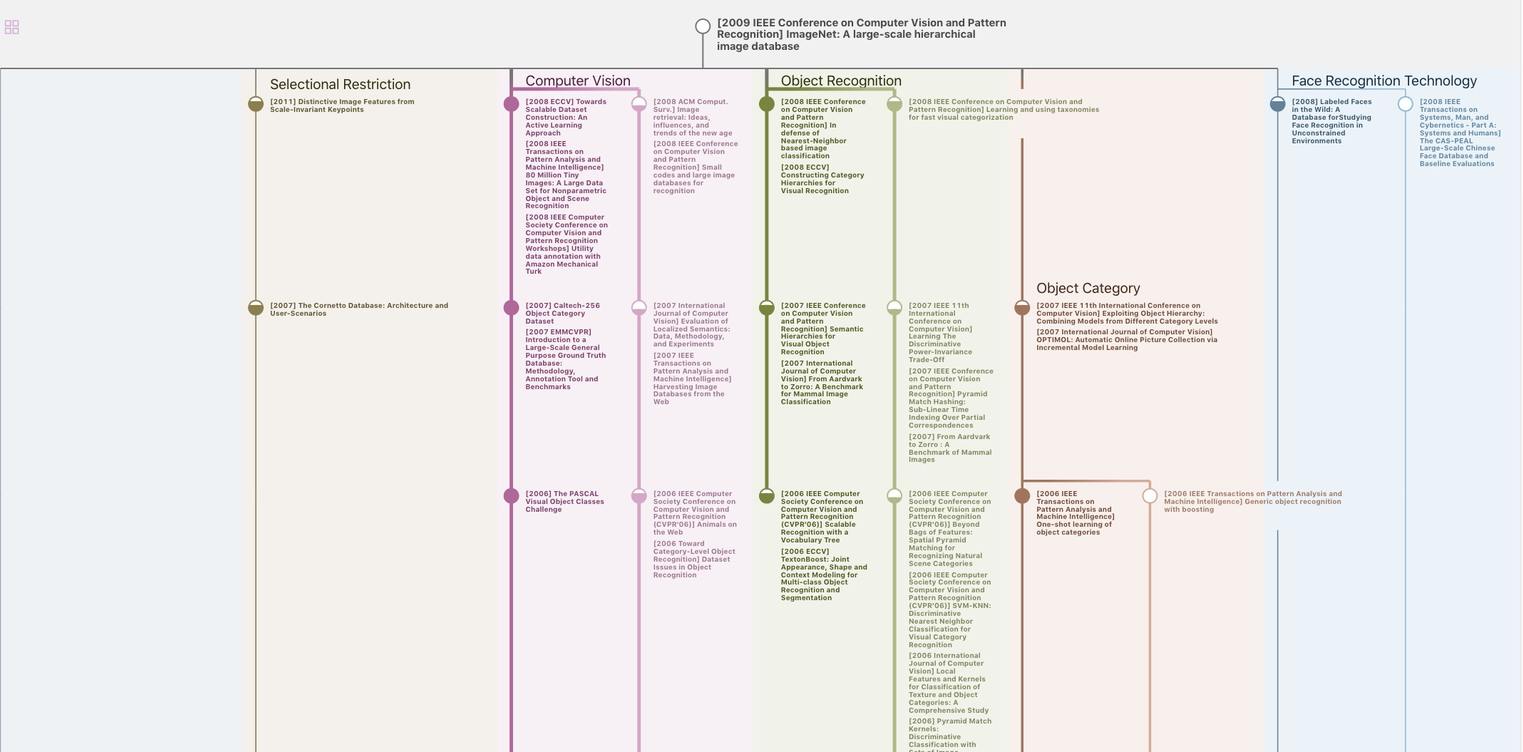
生成溯源树,研究论文发展脉络
Chat Paper
正在生成论文摘要