Robust Density Estimation under Besov IPM Losses
NIPS 2020(2020)
摘要
We study minimax convergence rates of nonparametric density estimation in the Huber contamination model, in which a proportion of the data comes from an unknown outlier distribution. We provide the first results for this problem under a large family of losses, called Besov integral probability metrics (IPMs), that includes ℒ^p, Wasserstein, Kolmogorov-Smirnov, and other common distances between probability distributions. Specifically, under a range of smoothness assumptions on the population and outlier distributions, we show that a re-scaled thresholding wavelet series estimator achieves minimax optimal convergence rates under a wide variety of losses. Finally, based on connections that have recently been shown between nonparametric density estimation under IPM losses and generative adversarial networks (GANs), we show that certain GAN architectures also achieve these minimax rates.
更多查看译文
关键词
besov ipm losses,robust density estimation
AI 理解论文
溯源树
样例
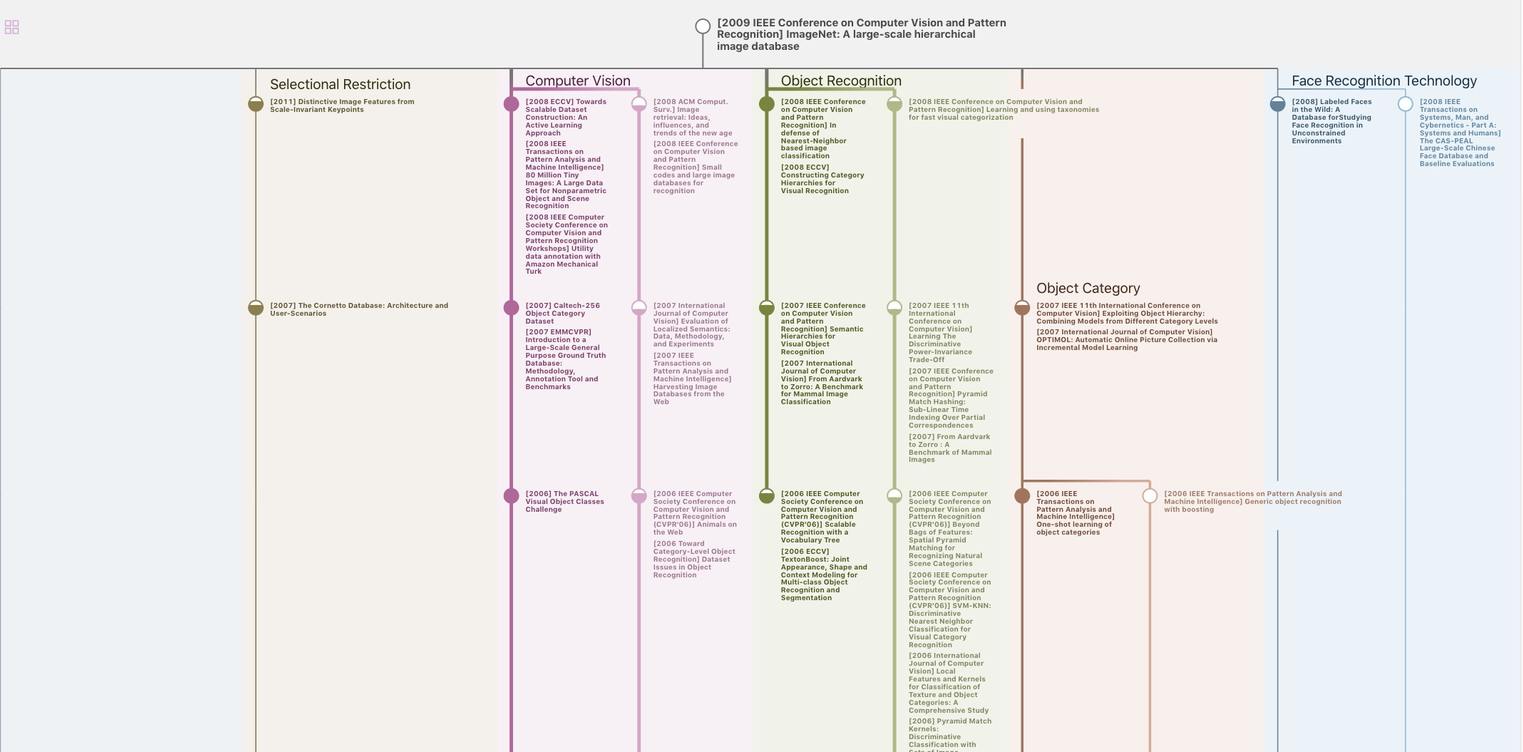
生成溯源树,研究论文发展脉络
Chat Paper
正在生成论文摘要