Deep Reinforcement Learning Based Indoor Air Quality Sensing By Cooperative Mobile Robots
2020 IEEE WIRELESS COMMUNICATIONS AND NETWORKING CONFERENCE (WCNC)(2020)
摘要
Confronted with the severe indoor air pollution nowadays, we propose the usage of multiple robots to detect the indoor air quality (IAQ) cooperatively for fewer sensors and larger sensing area. To acquire the complete real-time IAQ distribution map, we exploit the real statistical data to construct the IAQ data model and adopt Kalman Filter to obtain the estimation of the unmeasured area. Since the movement of the robots affects the estimation accuracy, a proper movement strategy should be planned to minimize the total estimation error. To solve this optimization problem, we design a deep Q-learning approach, which provides sub-optimal movement strategies for real-time robot sensing. By simulations, we verify the adopted IAQ data model and testify the effectiveness of the proposed solution. For application considerations, we have deployed this system in Peking University since Dec. 2018 and developed a website to visualize the IAQ distribution.
更多查看译文
关键词
deep reinforcement,indoor air quality sensing,cooperative mobile robots,indoor air pollution,larger sensing area,real-time IAQ distribution map,statistical data,Kalman filter,total estimation error,optimization problem,deep Q-learning approach,suboptimal movement strategies,real-time robot sensing,IAQ data model
AI 理解论文
溯源树
样例
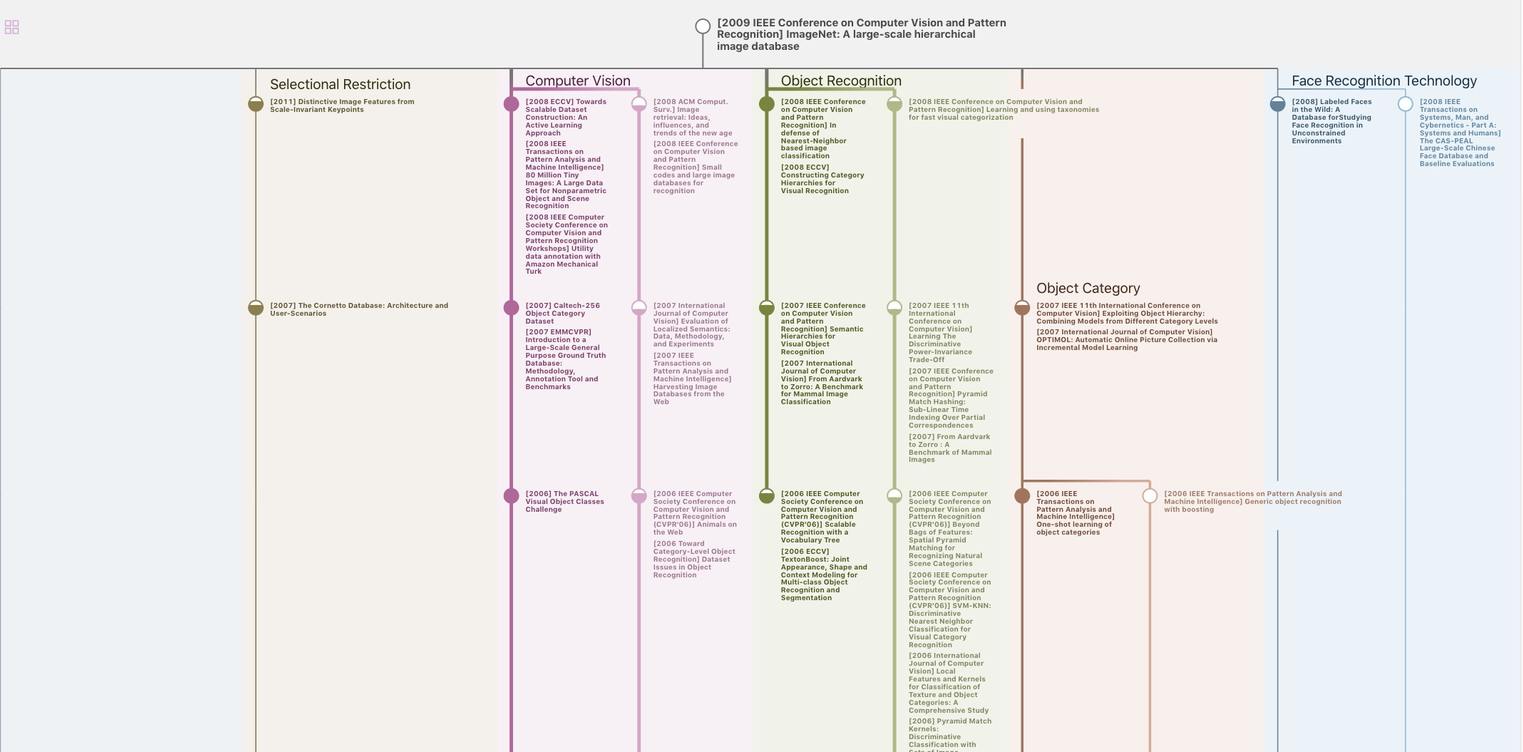
生成溯源树,研究论文发展脉络
Chat Paper
正在生成论文摘要