Latency Guaranteed Edge Inference Via Dynamic Compression Ratio Selection
2020 IEEE WIRELESS COMMUNICATIONS AND NETWORKING CONFERENCE (WCNC)(2020)
摘要
With the development of intelligent Internet of things (IoT) devices, implementing machine learning algorithms at the network edge has become essential to many applications, such as autonomous driving, environment monitoring. However, the limited computation capability and energy constraint results in difficulties of running complex machine learning algorithms on edge devices subject to latency requirements, and one solution is to offload the computation tasks to the edge server. However, the wireless transmission of raw data from devices to the server is time consuming and may violate the latency requirement. To this end, lossy data compression can be helpful, but the information loss may lead to erroneous learning result, e.g., wrong classification. In this paper, we propose a transmission scheme with compression ratio selection for inference tasks with task completion latency guarantee. By dynamically selecting the optimal compression ratio with the awareness of the remaining latency budget, more tasks can be timely completed and get the correct inference results under the communication resource constraint. Furthermore, retransmitting less compressed data of tasks with erroneous inference results can potentially enhance the average accuracy. However, it is often hard to know whether the inference result is correct or not. We therefore use uncertainty to estimate the confidence of the results, and based on that, jointly optimize the retransmission and compression ratio selection.
更多查看译文
关键词
compression ratio selection,wireless data transmission,retransmission ratio selection,task completion latency,intelligent Internet of things devices,latency guaranteed edge inference,erroneous inference results,compressed data,communication resource constraint,correct inference results,remaining latency budget,optimal compression ratio,inference tasks,transmission scheme,lossy data compression,wireless transmission,edge server,computation tasks,latency requirement,edge devices,complex machine learning algorithms,energy constraint results,environment monitoring,autonomous driving,network edge,dynamic compression ratio selection
AI 理解论文
溯源树
样例
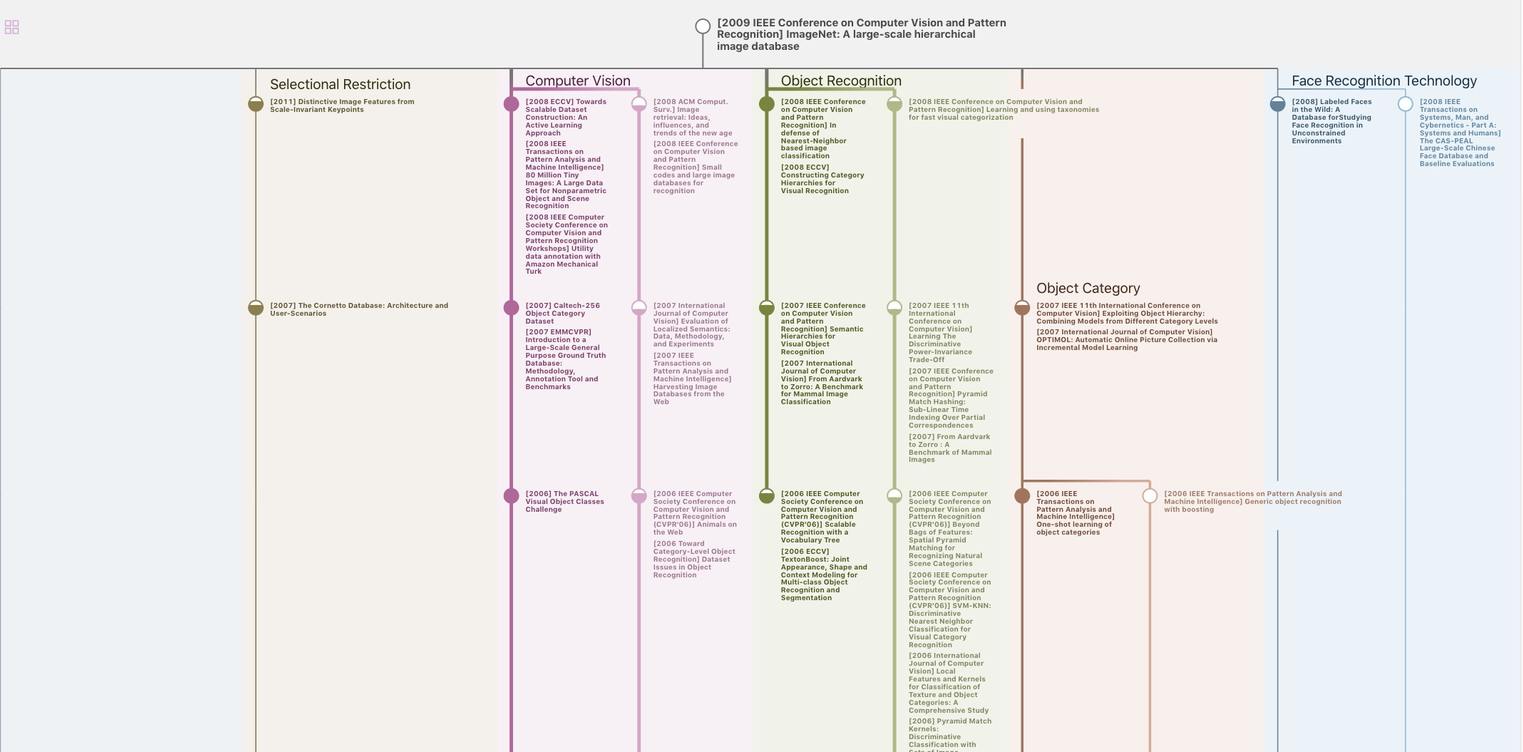
生成溯源树,研究论文发展脉络
Chat Paper
正在生成论文摘要