Structured feature for multi-label learning.
Neurocomputing(2020)
摘要
Multi-label learning tackles the problem in which each instance is composed of a single sample and associated with multiple labels simultaneously. In the past decades, many algorithms have been proposed for this emerging machine learning paradigm. However, these methods often focus on designing new classification strategies. If the original input data with a high dimensionality, it will suffer from the curse of dimensionality and get highly biased estimates. To address the issue, this paper proposes a novel feature construction approach named Structured Feature (SF), which can achieve a discriminant representation for multi-label data by exploiting the local and global geometric structures. These structures are characterized by the reconstruction coefficients of data in manifold and sparse subspaces. Since reconstruction coefficients reflect the data similarity relationships, and the similar data usually has the common class labels, SF incorporates the similarity relationships and supervised information into the feature representation. The SF contains supervised information, thus, even though classifier design ignores the correlations and interdependences between labels, it is able to obtain satisfactory classification performance.SF combines manifold and sparse structures in the feature representation for multi-label learning. Extensive experiments validate that SF can achieve comparable to or even better results than other state-of-the-art algorithms.
更多查看译文
关键词
Multi-label learning,Representation learning,Machine learning
AI 理解论文
溯源树
样例
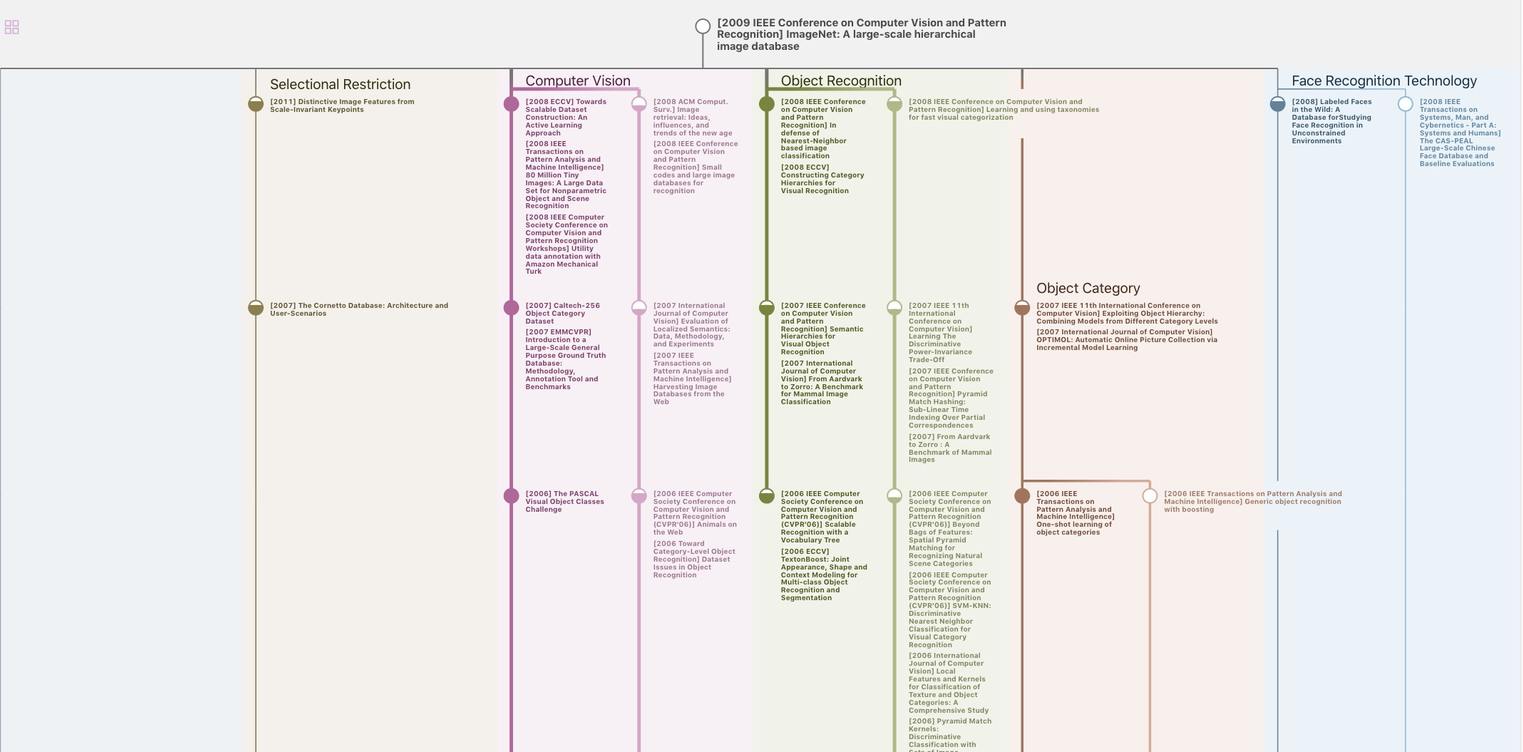
生成溯源树,研究论文发展脉络
Chat Paper
正在生成论文摘要