Deep subspace clustering to achieve jointly latent feature extraction and discriminative learning.
Neurocomputing(2020)
摘要
Deep subspace clustering based on autoencoder and self-expression layer has become popular for clustering method. However, these models only focus on feature extraction by minimizing reconstruction error rather than specific clustering task, leading to unsatisfactory performance. To overcome the above shortcoming, we propose a deep subspace clustering framework which jointly extracts features via the embedding neural network and performs subspace learning. The model contains three modules, an autoencoder, a self-expression layer and a supervised competitive feature learning module. The proposed model is highly capable of capturing characteristic features to guide the clustering task by using relative entropy to minimize probabilistic cluster assignments and the target variables. The three modules are consolidated to be jointly trained and optimized competitively. Experimental results on five benchmark datasets demonstrate the effectiveness of the proposed deep subspace clustering by comparing with eleven baseline methods.
更多查看译文
关键词
Deep clustering,Unsupervised learning,Deep networks,Autoencoder,Feature learning,Self-expression
AI 理解论文
溯源树
样例
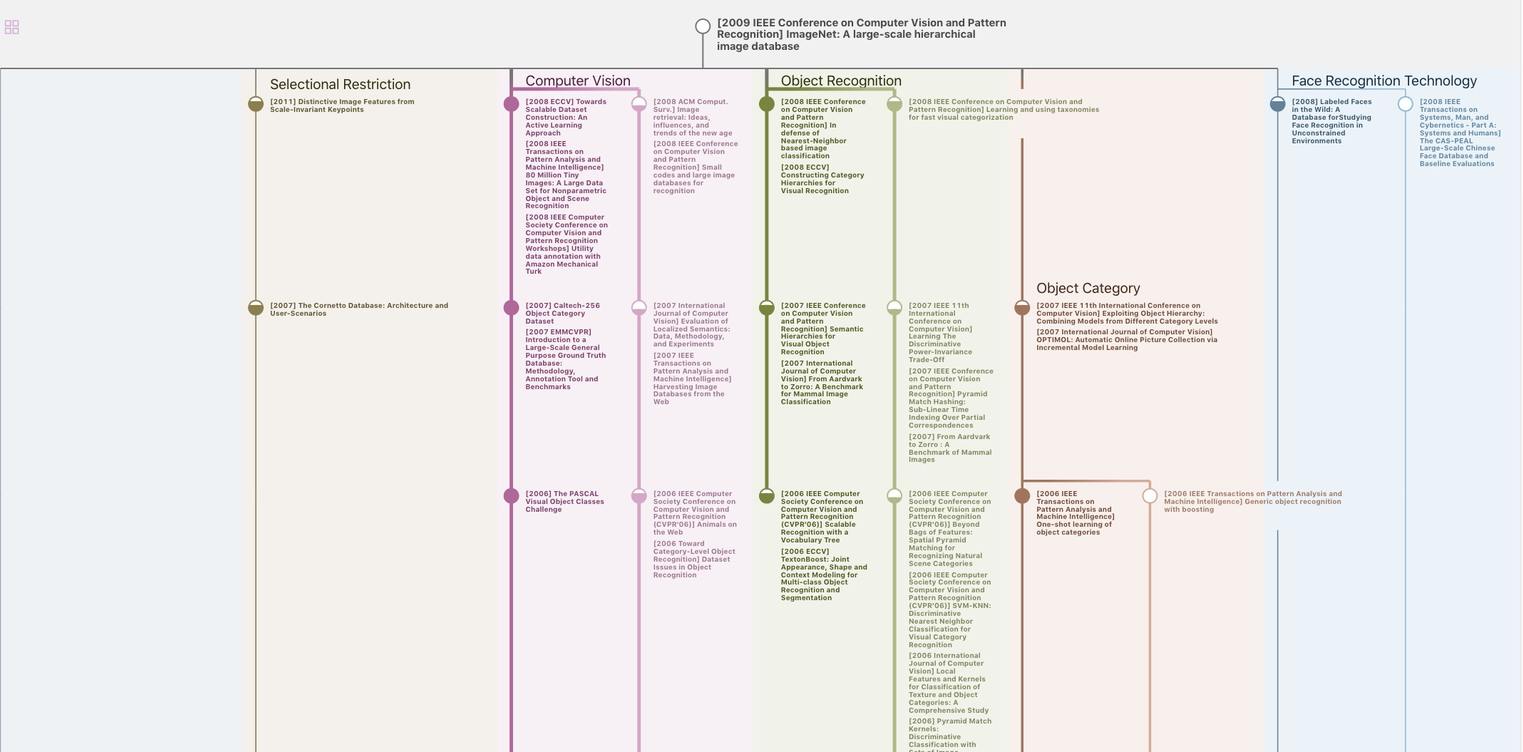
生成溯源树,研究论文发展脉络
Chat Paper
正在生成论文摘要