Leveraging The Fisher Randomization Test Using Confidence Distributions: Inference, Combination And Fusion Learning
JOURNAL OF THE ROYAL STATISTICAL SOCIETY SERIES B-STATISTICAL METHODOLOGY(2021)
摘要
The flexibility and wide applicability of the Fisher randomization test (FRT) make it an attractive tool for assessment of causal effects of interventions from modern-day randomized experiments that are increasing in size and complexity. This paper provides a theoretical inferential framework for FRT by establishing its connection with confidence distributions. Such a connection leads to development's of (i) an unambiguous procedure for inversion of FRTs to generate confidence intervals with guaranteed coverage, (ii) new insights on the effect of size of the Monte Carlo sample on the estimation of a p-value curve and (iii) generic and specific methods to combine FRTs from multiple independent experiments with theoretical guarantees. Our developments pertain to finite sample settings but have direct extensions to large samples. Simulations and a case example demonstrate the benefit of these new developments.
更多查看译文
关键词
exact test, fiducial, model-free, monte carlo, p-value, sharp nulle
AI 理解论文
溯源树
样例
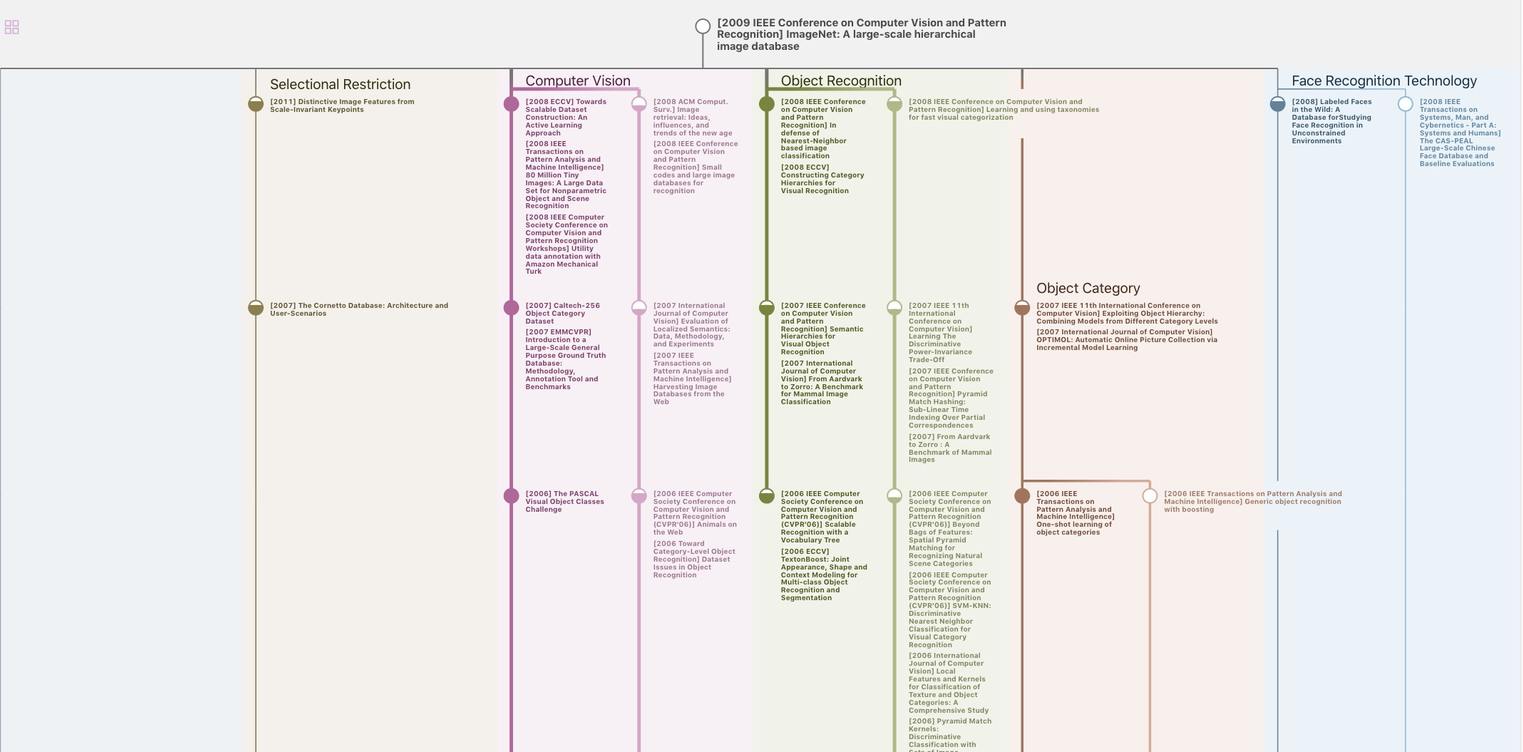
生成溯源树,研究论文发展脉络
Chat Paper
正在生成论文摘要