Task-adaptive Asymmetric Deep Cross-modal Hashing
KNOWLEDGE-BASED SYSTEMS(2021)
摘要
Supervised cross-modal hashing aims to embed the semantic correlations of heterogeneous modality data into the binary hash codes with discriminative semantic labels. It can support efficient large-scale cross-modal retrieval due to the fast retrieval speed and low storage cost. However, existing methods equally handle the cross-modal retrieval tasks, and simply learn the same couple of hash functions in a symmetric way. Under such circumstances, the characteristics of different cross-modal retrieval tasks are ignored and sub-optimal performance may be brought. Motivated by this, we present a Task-adaptive Asymmetric Deep Cross-modal Hashing (TA-ADCMH) method in this paper. It can learn task-adaptive hash functions for two sub-retrieval tasks via simultaneous modality representation and asymmetric hash learning. Different from previous cross-modal hashing methods, our learning framework jointly optimizes the semantic preserving from multi-modal features to the hash codes, and the semantic regression from query modality representation to the explicit labels. With our model, the learned hash codes can effectively preserve the multi-modal semantic correlations, and meanwhile, adaptively capture the query semantics. Besides, we design an efficient discrete optimization strategy to directly learn the binary hash codes, which alleviates the relaxing quantization errors. Extensive experiments demonstrate the state-of-the-art performance of the proposed TA-ADCMH from various aspects. (C) 2021 Elsevier B.V. All rights reserved.
更多查看译文
关键词
Cross-modal similarity retrieval,Task-adaptive,Asymmetric Deep Hashing Learning
AI 理解论文
溯源树
样例
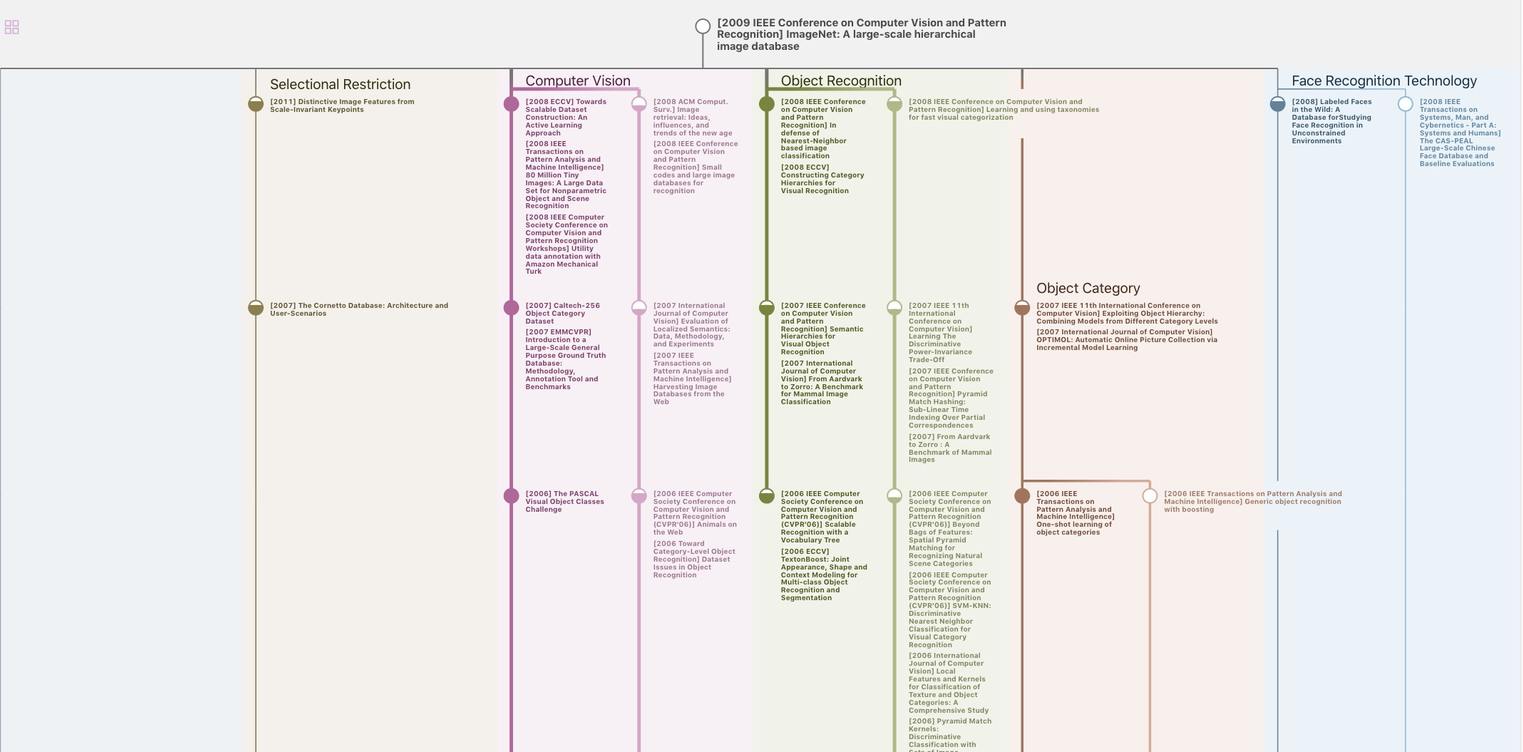
生成溯源树,研究论文发展脉络
Chat Paper
正在生成论文摘要