Bayesian Model Averaging as an Alternative to Model Selection for Multilevel Models
MULTIVARIATE BEHAVIORAL RESEARCH(2021)
摘要
We investigated the Bayesian model averaging (BMA) technique as an alternative method to the traditional model selection approaches for multilevel models (MLMs). BMA synthesizes the information derived from all possible models and comes up with a weighted estimate. A simulation study compared BMA with additional modeling techniques, including the single "best" model approach, Bayesian MLM using informative, diffuse, and inaccurate priors, and restricted maximum likelihood. A two-level random intercept and random slope model was examined with these modeling techniques. Generated data used two types of true models: a full MLM and a reduced MLM. Findings of the simulation study suggested that BMA was a trustworthy alternative to traditional model comparison and selection approaches through the Bayesian and the frequentist frameworks. We also include an empirical example highlighting the extension of MLMs into the BMA framework, as well as model interpretation.
更多查看译文
关键词
Bayesian model averaging, model selection, multilevel modeling, Bayesian estimation
AI 理解论文
溯源树
样例
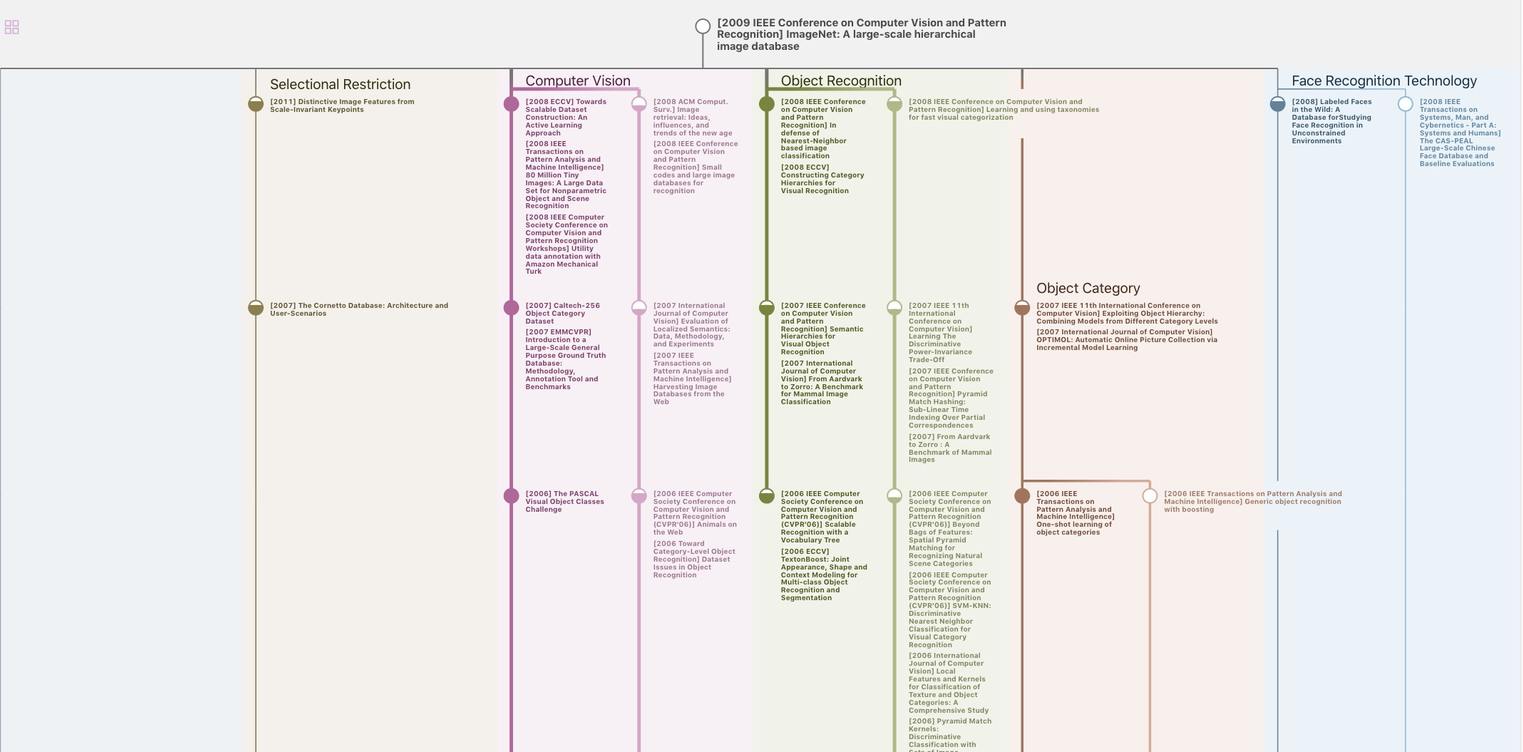
生成溯源树,研究论文发展脉络
Chat Paper
正在生成论文摘要