Leveraging Adversarial Training in Self-Learning for Cross-Lingual Text Classification
SIGIR '20: The 43rd International ACM SIGIR conference on research and development in Information Retrieval Virtual Event China July, 2020(2020)
摘要
In cross-lingual text classification, one seeks to exploit labeled data from one language to train a text classification model that can then be applied to a completely different language. Recent multilingual representation models have made it much easier to achieve this. Still, there may still be subtle differences between languages that are neglected when doing so. To address this, we present a semi- supervised adversarial training process that minimizes the maximal loss for label-preserving input perturbations. The resulting model then serves as a teacher to induce labels for unlabeled target lan- guage samples that can be used during further adversarial training, allowing us to gradually adapt our model to the target language. Compared with a number of strong baselines, we observe signifi- cant gains in effectiveness on document and intent classification for a diverse set of languages.
更多查看译文
关键词
Text Classification, Multilingual, Cross-Lingual, Semantics
AI 理解论文
溯源树
样例
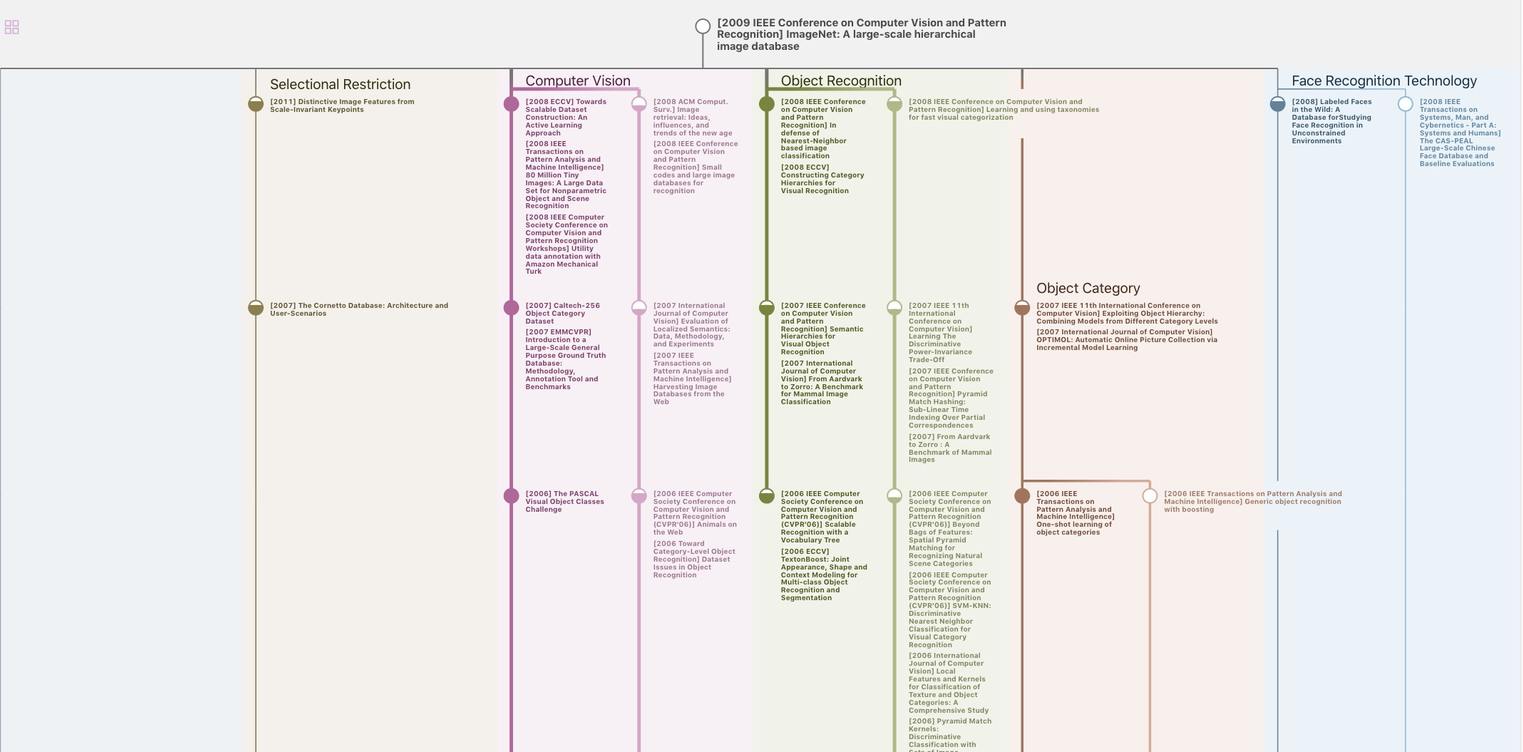
生成溯源树,研究论文发展脉络
Chat Paper
正在生成论文摘要