Label-Consistency based Graph Neural Networks for Semi-supervised Node Classification
SIGIR '20: The 43rd International ACM SIGIR conference on research and development in Information Retrieval Virtual Event China July, 2020(2020)
摘要
Graph neural networks (GNNs) achieve remarkable success in graph-based semi-supervised node classification, leveraging the information from neighboring nodes to improve the representation learning of target node. The success of GNNs at node classification depends on the assumption that connected nodes tend to have the same label. However, such an assumption does not always work, limiting the performance of GNNs at node classification. In this paper, we propose label-consistency based graph neural network (LC-GNN), leveraging node pairs unconnected but with the same labels to enlarge the receptive field of nodes in GNNs. Experiments on benchmark datasets demonstrate the proposed LC-GNN outperforms traditional GNNs in graph-based semi-supervised node classification. We further show the superiority of LC-GNN in sparse scenarios with only a handful of labeled nodes.
更多查看译文
关键词
graph neural networks, node classification, semi-supervised learning
AI 理解论文
溯源树
样例
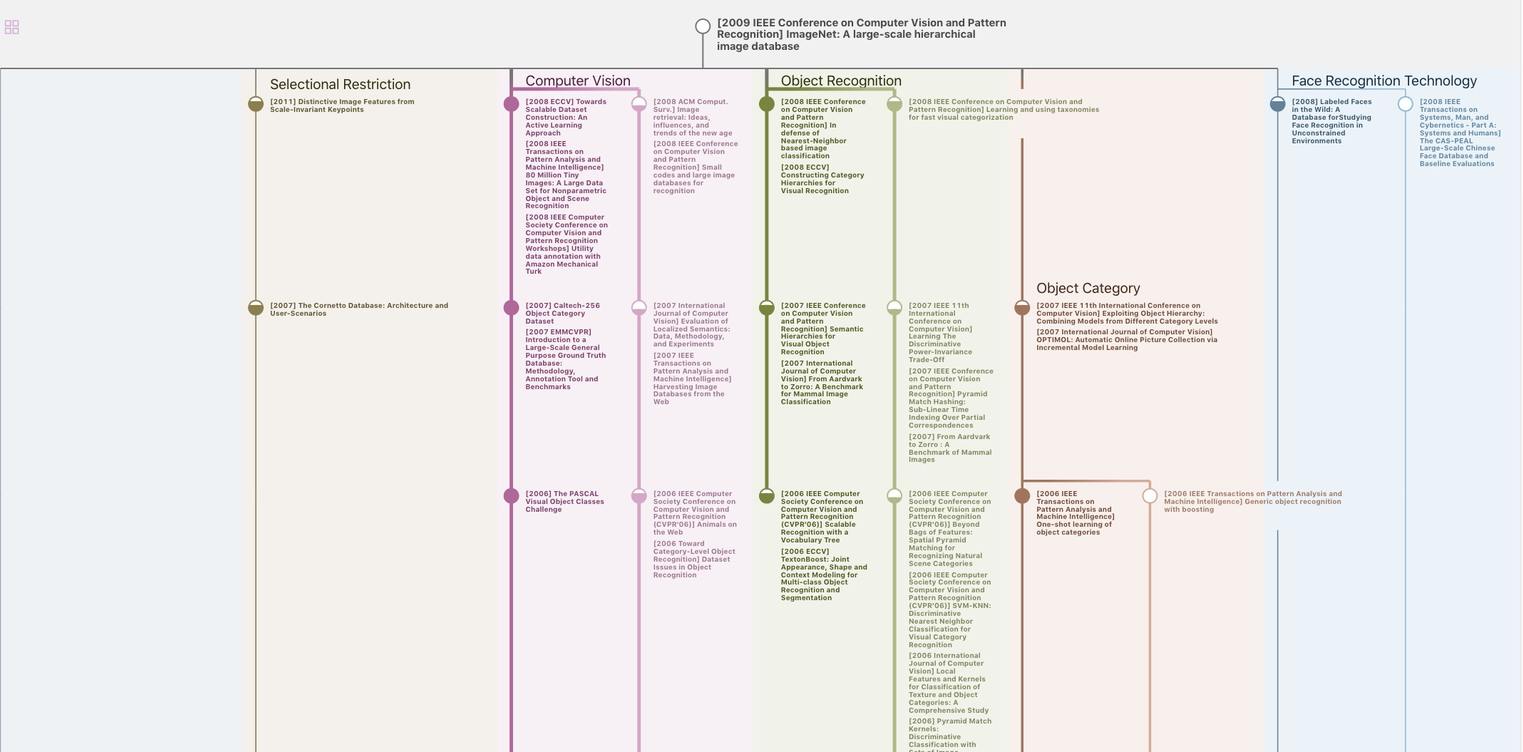
生成溯源树,研究论文发展脉络
Chat Paper
正在生成论文摘要