Having Your Cake and Eating it Too: Training Neural Retrieval for Language Inference without Losing Lexical Match
SIGIR '20: The 43rd International ACM SIGIR conference on research and development in Information Retrieval Virtual Event China July, 2020(2020)
摘要
We present a study on the importance of information retrieval (IR) techniques for both the interpretability and the performance of neural question answering (QA) methods. We show that the current state-of-the-art transformer methods (like RoBERTa) encode poorly simple information retrieval (IR) concepts such as lexical overlap between query and the document. To mitigate this limitation, we introduce a supervised RoBERTa QA method that is trained to mimic the behavior of BM25 and the soft-matching idea behind embedding-based alignment methods. We show that fusing the simple lexical-matching IR concepts in transformer techniques results in improvement a) of their (lexical-matching) interpretability, b) retrieval performance, and c) the QA performance on two multi-hop QA datasets. We further highlight the lexical-chasm gap bridging capabilities of transformer methods by analyzing the attention distributions of the supervised RoBERTa classifier over the context versus lexically-matched token pairs.
更多查看译文
关键词
Semantic alignment, Question answering, Interpretability
AI 理解论文
溯源树
样例
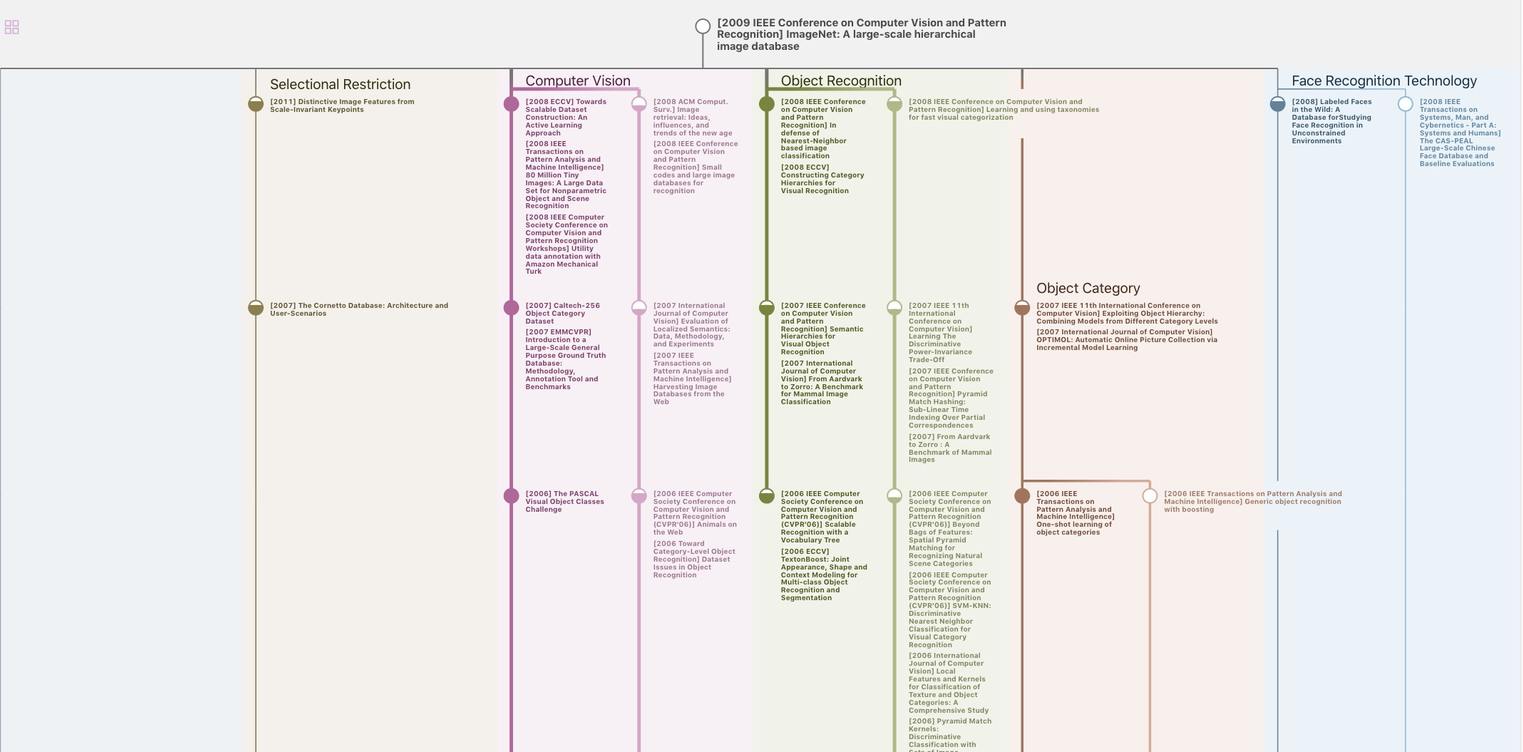
生成溯源树,研究论文发展脉络
Chat Paper
正在生成论文摘要