Influence Function for Unbiased Recommendation
SIGIR '20: The 43rd International ACM SIGIR conference on research and development in Information Retrieval Virtual Event China July, 2020(2020)
摘要
Recommender system is one of the most successful machine learning technologies for commerce. However, it can reinforce the closed feedback loop problem, where the recommender system generates items to users, then the further recommendation model is trained with the data that users' feedback to the items. Such self-reinforcing pattern can cause data bias problems. There are several debiasing methods, inverse-propensity-scoring (IPS) is a practical one for industry product. Since it is relatively easy to reweight training samples, and ameliorate the distribution shift problem. However,because of deterministic policy problem and confoundings in real-world data, it is hard to predict propensity score accurately. Inspired by the sample reweight work for robust deep learning, we propose a novel influence function based method for recommendation modeling, and analyze how the influence function corrects the bias. In the experiments, our proposed method achieves better performance against the state-of-the-art approaches.
更多查看译文
关键词
recommender system, Influence Function, counterfactual learning
AI 理解论文
溯源树
样例
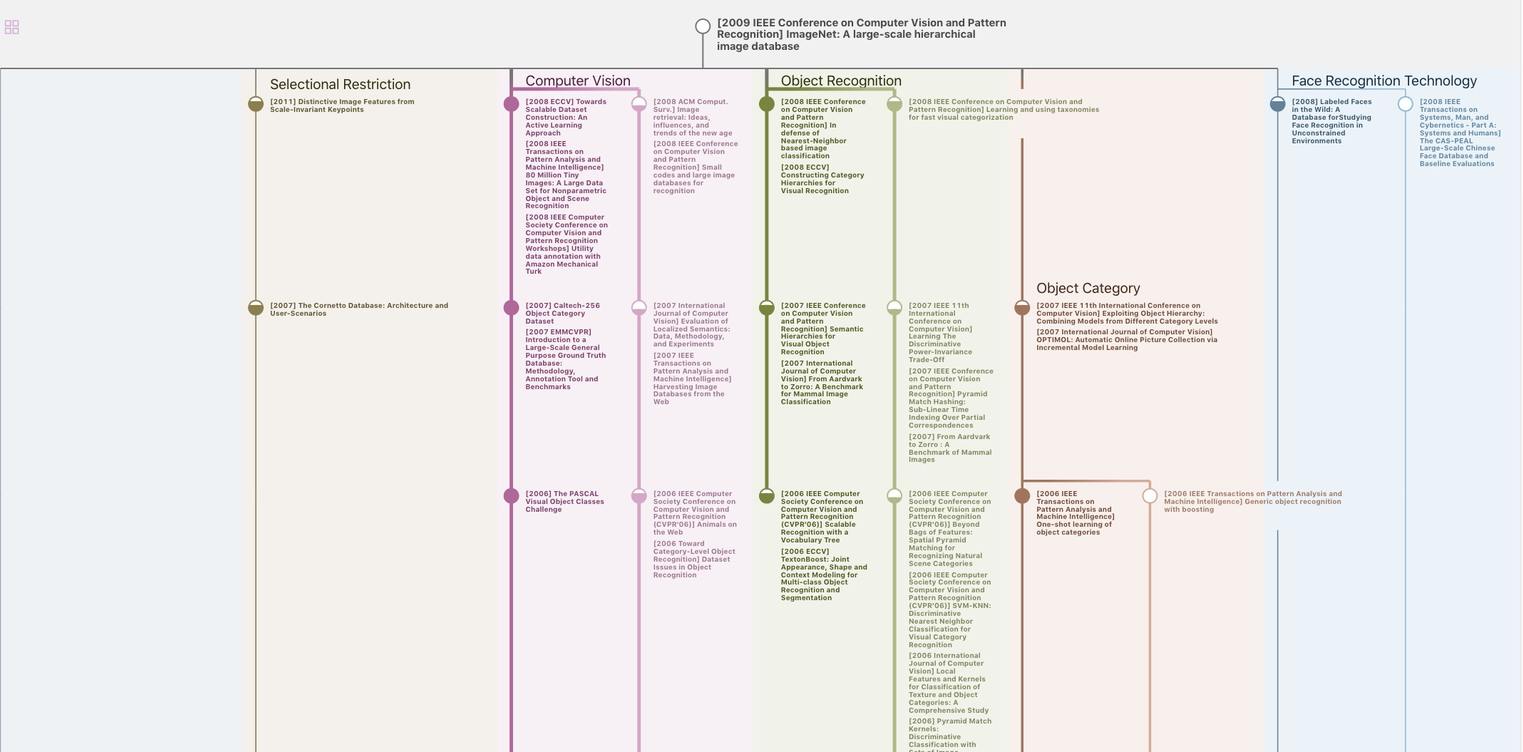
生成溯源树,研究论文发展脉络
Chat Paper
正在生成论文摘要